A cuproptosis-related signature for predicting the prognosis of gastric cancer
Highlight box
Key findings
• The cuproptosis-related signature is a valuable independent prognostic factor that can predict the prognosis of gastric cancer (GC).
What is known and what is new?
• Cuproptosis is a newly discovered type of cell death caused by protein toxicity stress, with copper playing a major role in GC.
• We established a cuproptosis-related signature, providing a new perspective from which to approach the treatment and prognosis of nonapoptotic cuproptosis in GC.
What is the implication, and what should change now?
• The cuproptosis-related prognostic signature can complement or optimize the existing prognosis model of cuproptosis in GC.
Introduction
Gastric cancer (GC) is the world’s fifth most widespread malignancy and the fourth leading cause of death (1). The incidence and fatality rates of GC have reduced in most populations due to improvements in hygiene and Helicobacter pylori (HP) prevention (2). However, the incidence of GC is increasing among young adults in some European countries, probably due to microbiota dysbiosis induced by antibiotic abuse (3,4).
Noncardiac GC is considered to be caused by chronic HP infection (5,6). The study has shown that HP infections trigger nuclear factor-κB (NF-κB)-induced inflammation by long noncoding RNA (lncRNA) H19, which leads to the growth of GC cells (7). Copper is an essential element and participates in many important redox reactions. The study has shown that copper is also associated with HP infection. For example, in mice, copper deprivation affects HP infection, and copper tetrathiomolybdate chelate reduces the role of macrophages in these infections by reducing copper utilization (8). Some studies have found that copper depletion caused by tetrathiomolybdate (TM) can affect immune response, which may regulate PD-L1 expression and affect tumor immune escape (9,10). and the study has also shown that the prognosis model constructed by the genes related to cuproptosis and ferroptosis has good prognosis benefits in liver cancer (11). It indicates that there is a correlation between cuproptosis and cancer development. Additionally, the study has claimed there to be a connection between serum copper levels and HP infection in the stomach (12). A previous study has also indicated that copper directly binds to the lipid components of the tricarboxylic acid (TCA) cycle, causing protein toxic stress and ultimately leading to cell death in a process called cuproptosis (13). Moreover, other research has found that the copper-exporting protein ATP7A promotes tumor growth by increasing copper levels in cells (14). Several studies have also indicated that copper plays a major role in GC. For example, the production of reactive oxygen species (ROS) by disulfiram (DSF)/Cu induces the growth inhibition of GC cells via glycolysis (15,16). Nevertheless, the role of cuproptosis in GC remains unclear.
Therefore, in the present study, we performed a functional analysis of the cuproptosis-related genes in GC, established a cuproptosis-related signature (CRs), and evaluated the tumor immunity of this signature. We then compared the expression of genes linked to immunology, m6A, m7G, ferroptosis, and chemoresistance in the 2 sets of the CRs. Finally, we used single-cell RNA sequencing (scRNA-seq) to analyze the expression of cuproptosis-related genes (CRGs) in different clusters and explored their potential functions. Although several similar reports about the CRs have been published (17-19) , the majority do not joint prediction of immunology, m6A, m7G, ferritosis, and chemoresistance effects of GC, and not combine scRNA-sq multi-dimensional analysis of the differential expression of CRGs in gastric cancer. We present the following article in accordance with the TRIPOD reporting checklist (available at https://jgo.amegroups.com/article/view/10.21037/jgo-23-62/rc).
Methods
Data collection
The RNA-seq data for stomach adenocarcinoma (STAD) transcriptome profiling data, single-nucleotide variation, and clinicopathological data of patients with GC were retrieved from The Cancer Genome Atlas (TCGA; http://www.cbioportal.org/). The corresponding CRGs were downloaded from a previous article (13). We also retrieved the GSE54129 scRNA-seq data for GC from the Gene Expression Omnibus (GEO) database (https://www.ncbi.nlm.nih.gov/geo/). The miRcode database (http://www.mircode.org/) was used to obtain the “highly conserved miRNA families” file.
The FerrDb database (http://www.zhounan.org/ferrdb/legacy/index.html) was used to collect the ferroptosis-related genes. The Molecular Signatures Database (MSigDB; https://www.gsea-msigdb.org/gsea/msigdb) was used to obtain the “hallmark gene sets browse 50 gene sets” file (H-related genes).
Differential expression analysis of CRGs in GC and tumor mutation burden (TMB)
The Pearson correlation coefficient was used to analyze the expression level of CRGs in GC, with P<0.05 being considered statistically significant. Genomic events in these genes and overall TMB analysis were visualized using the “maftools” package in R (The R Foundation for Statistical Computing).
Differentially expressed CRGs and CRs
The TCGA-STAD RNA-seq data set was analyzed using R studio software. The relationship between lncRNAs and the CRGs of GC was assessed using Pearson correlation coefficient. The CRs of GC were screened using a P value <0.05 and a correlation coefficient >0.3. The differentially expressed (DE)-CRGs were screened using a P value <0.05. A |log fold change (FC)| >1 and false discovery rate (FDR) <0.05 were used as cutoff points to identify DE-CRGs.
Functional analysis and construction of the gene expression networks
The pathway enrichment associated with DE-CRGs was determined based on the Kyoto Encyclopedia of Genes and Genomes (KEGG) and Gene Ontology (GO). Rstudio software was used to extract the DE-associated microRNAs (miRNAs) from the “highly conserved miRNA families” file from the miRcode database. The cuproptosis-related messenger RNA (mRNA)-lncRNA-miRNA network was constructed using Cytoscape (v. 3.8.0).
By applying the single-sample gene set enrichment analysis (ssGSEA) method from the “GSVA” R package, we calculated the extent of H-, ferroptosis-, m6A-, and m7G-related genes according to their expression levels (ssGSEA-score). The relationship between CRGs and ssGSEA-score was assessed using Pearson correlation coefficient with the R package “Hmisc”.
Construction of the prognostic signature (CRs)
We examined the relationship between the overall survival (OS) and the 2 groups of the CRs using Cox regression. The DE-CRGs with P<0.05 were considered to have prognostic value. The training data set included 371 patients with GC from TCGA. Then, 199 patients within TCGA were randomly selected as the testing data set. We used least absolute shrinkage and selection operator (LASSO) and multivariate Cox regression analyses to construct the CRs using the following formula: risk score = (expression of lncRNA1) × coefficient (lncRNA1) + (expression of lncRNA2) × coefficient (lncRNA3) + (expression of lncRNAn) × coefficient (lncRNAn). The median risk score was used as the risk cutoff criteria to divide GC patients into low- and high-risk groups. Using the “survminer” R package via Rstudio, we generated Kaplan-Meier survival curves to analyze the OS of the low- and high-risk groups. The area under the receiver operating characteristic (ROC) curve (AUC) value was used to evaluate the performance of the CRs via the “timeROC” R package.
Independent prognostic analysis and nomogram construction
We used univariate and multivariate Cox regression analyses to evaluate the prognostic value of the CRs. Considering grade, stage, age, gender, and risk score, we evaluated the predictive prognostic accuracy of the CRs and other clinicopathological features using AUC values. The decision curve analysis (DCA) was used to assess the utility of the CRs for decision-making. A heatmap was used to clarify the correlation between clinicopathological features and risk scores. Finally, to evaluate the clinical utility of the CRs, we constructed a nomogram predicting the 1, 2, and 3-year OS.
Gene set enrichment analysis
We used the software (4.1.0) to perform GSEA and identify which signaling pathways were enriched in the different groups of the CRs. The canonical pathways MSigDB category (c2.cp.v7.5.symbols.gmt) were used as the reference gene set, and other parameters were set based on default values. The top 10 most relevant pathways were drawn using the “multiGSEA” package.
Immunity analysis and gene expression
Cell-type identification by estimating relative subsets of RNA transcripts (CIBERSORT), tumor immune estimation resource (TIMER), Microenvironment cell populations-counter (MCP-counter) and Cell types enrichment analysis (xCell) algorithms were used to quantify the tumor-infiltrating immune cells between the 2 groups of the CRs. Moreover, the immune function of the CRs was assessed using ssGSEA. The FerrDb was used to retrieve ferroptosis-related genes. Then, we analyzed the relation between the expressions of immunological checkpoints, m6A-, m7G-, and ferroptosis-related genes and the CRs.
Prediction of response to chemotherapy
The “pRRophetic” R package was used to predict the half maximal inhibitory concentration (IC50) of the common chemotherapeutic agents. IC50 indicates the effectiveness of a substance in inhibiting specific biological or biochemical functions. The difference between groups was tested with Wilcoxon test analysis.
Processing of the scRNA-Seq data
First, the matrix was converted to a Seurat object, and the data were filtered by setting genes >50 and mitochondrial content <5%. The dimension reduction analysis was based on the principal component analysis (PCA) algorithm and cluster analysis using the t-distributed stochastic neighbor embedding (tSNE) algorithm. Marker genes were screened by setting an adjusted P value <0.05 and |log Fc| >1 as the cutoff criteria. KEGG enrichment analysis and cellular functions of each cluster were performed using ClueGO in Cytoscape. Finally, the cellular annotation was processed using the R package ‘SingleR’, and the study was conducted in accordance with the Declaration of Helsinki (as revised in 2013).
Statistical analyses
R software (v. 3.6.3) was used to analyze the data. The Wilcoxon test was used to screen the DE-lncRNAs. The survival of patients with GC was assessed using Kaplan-Meier survival curves. The ROC and DCA curves were used to analyze the accuracy and detection rate of the CRs and clinicopathological variables for GC. Logistic regression analysis was used to explore the correlation between the CRs and clinicopathological symptoms. A P value <0.05 was considered statistically significant.
Results
Analysis of genetic differences and TMB of the CRGs in GC
A total of 8 DE-CRGs were obtained from the TCGA-STAD RNA-seq expression profile (Figure 1A). There were 9 CRGs with TMB >1% in GC (Figure 1B). The results showed that DE-CRGs were correlated with GC (P>0.05), and the TMB values suggested that the high expression of LIPT1 and ATP7A may have better immunotherapy effects.
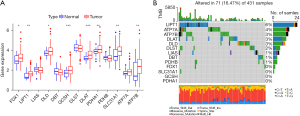
Enrichment analyses and construction of an mRNA-lncRNA-miRNA Network
A total of 697 DE-CRGs were obtained from the TCGA-STAD RNA-seq expression profile, and 83 lncRNA-associated miRNAs were retrieved from miRcode (https://cdn.amegroups.cn/static/public/jgo-23-62-1.xlsx). Then, we constructed a cuproptosis-related mRNA-lncRNA-miRNA network (Figure 2A). The GO analysis showed that the 8 DE-CRGs, among which included DLAT, GCSH, PDHA1, FDX1, and LIPT1, mainly participated in the following biological functions: acetyl-CoA biosynthetic process from pyruvate, thioester biosynthetic process, nucleoside bisphosphate biosynthetic process, and tricarboxylic acid (TCA) cycle. These genes participated in the regulation of the following molecular functions: oxidoreductase, s-acyltransferase activity, metal ion transmembrane transporter activity, cell composition of the mitochondrial matrix, oxidoreductase complex, dihydrolipoyl dehydrogenase complex, and TCA cycle enzyme complex (Figure 2B). The KEGG enrichment analysis revealed that the 8 DE-CRGs, among which were DLAT, PDHA1, and DLD, were primarily involved in carbon metabolism, platinum drug resistance, TCA cycle, multiple amino acid metabolic pathways, pyruvate metabolism, and glycolysis/gluconeogenesis (Figure 2C).
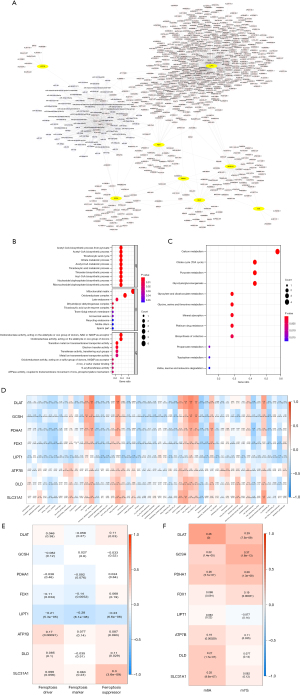
Through Pearson correlation analysis, the results showed that the DE-CRGs were associated with cell junction, repair of DNA damage , genes encoding cell cycle related targets of E2F transcription factors, mTOR signaling pathway, regulation of cancer promoting gene MYC, oxidative phosphorylation process, P53 signaling pathway, notch signaling pathway, spermatogenesis, TGF-β signaling pathway, and UV damage response process (Figure 2D), indicating that DE-CRGs may be involved in DNA repair process, cell cycle and proliferation, epithelial cell–mesenchymal transformation (EMT), mTOR signaling pathway, regulatory process of activating proto-oncogene Myc, oxidative phosphorylation pathway, P53 signaling pathway, and Notch signaling pathway. In the correlation analysis with ferroptosis, m6A, and m7G, the results showed that LIPT1 and FDX1 had a significant correlation with ferroptosis (P<0.05; Figure 2E); DLAT, GCSH, PDHA1, FDX1, LIPT1, and ATP7B had significant correlations with m6A and m7G (P<0.05); and DLD and SLC31A1 had a significant correlation with m6A (P<0.05; Figure 2F), suggesting that the occurrence of cuproptosis may be related to the m6A and m7G methylation pathways and the ferroptosis pathway.
Construction of the CRs
The multivariate Cox analysis identified 6 DE-CRGs (AL512506.1, AC016737.1, AC090204.1, AP001363.2, TYMSOS, and AL353804.2) that were associated with GC prognosis (Table S1, Figure 3A). The heatmaps of 6 CRs associated with GC prognosis are shown in Figure 3B. Next, we constructed a CRs. In the training data set, patients with GC were separated into 2 groups: high-risk (n=185) and low-risk (n=186). The high-risk group had a significantly lower OS rate than did the low-risk group (Figure 3C; P<0.01). The time-dependent AUCs were used to evaluate the validity of the CRs. The AUC values of the CRs were 0.668, 0.697, and 0.733 for 1-, 2-, and 3-year OS, respectively (Figure 3D). In the testing data set, patients with GC were also separated into 2 groups: high risk (n=100) and low risk (n=99). Significant variations in the OS rates were detected between the 2 groups (Figure 3E; P<0.01). The AUC values for the 1-, 2-, and 3-year OS were 0.638, 0.715, and 0.715, respectively (Figure 3F).
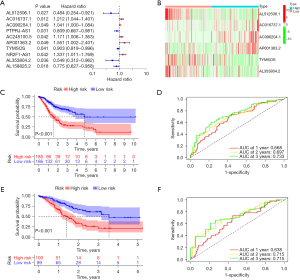
Independent prognostic analyses and nomogram construction
The CRs, stage, and age were strongly related to the poor outcome of patients with GC in both the univariate and multivariate Cox regression analyses (Figure 4A,4B). The AUC of the CRs was 0.668 (Figure 4C). The DCA demonstrated that the CRs provided greater predictive benefit compared to the original clinicopathological features (Figure 4D). Based on the nomogram, the greater the value of the CRs was, the higher the contribution to the model, and the lower the 1-, 3-, and 5-year survival rates were (Figure 4E). Finally, we used a heatmap to explore the association between the CRs and traditional clinicopathological manifestations (Figure 4F).
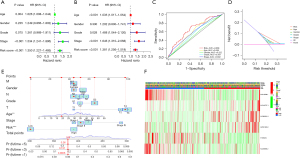
Gene set enrichment analysis
The GSEA indicated that the high-risk samples were involved in hypertrophic cardiomyopathy, dilated cardiomyopathy, tumor growth factor-β (TGF-β) signaling pathway, extracellular matrix (ECM)-receptor interaction, vascular smooth muscle contraction, focal adhesion, complement and coagulation cascades, glycosaminoglycan biosynthesis-keratan sulfate, and cytochrome P450 metabolism (Figure 5A). Meanwhile, low-risk samples were enriched in base excision repair, DNA mismatch repair (MMR), primary immunodeficiency, DNA replication, homologous recombination (HR), RNA degradation, nucleotide excision repair (NER), pantothenate and CoA biosynthesis, nicotinate and nicotinamide metabolism, and terpenoid backbone biosynthesis (Figure 5B).
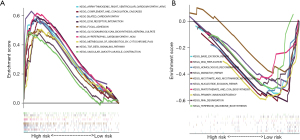
Immunity analyses and gene expression
Based on TCGA-STAD data, a heatmap of tumor-immune infiltrates according to different algorithms between the 2 groups of the CRs was created (Figure 6A). MHC class-I and type II interferon (IFN) response was remarkably different between the 2 subgroups of the CRs according to the immune function correlation study based on TCGA-STAD data (Figure 6B). A significant disparity in the expression of immunological checkpoints, including TNFSF18, CD200, TNFRSF25, NRP1, LGALS9, and TNFRSF14, among others, was also detected between the 2 groups of the CRs (Figure 6C).
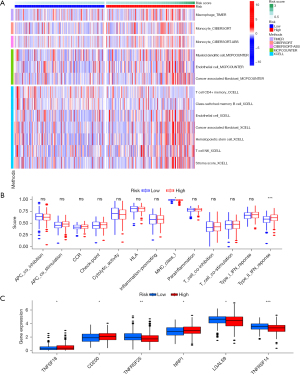
Moreover, the correlation analysis indicated that the levels of m6A-related genes, including ZC3H13, RBM15, YTHDC1, METTL3, and YTHDC2, and m7G-related genes, including METTL1 and WDR4, were remarkably different among the 2 groups of the CRs (Figure 7A). Interestingly, the expression of many ferroptosis-related genes, including ferroptosis-driver, ferroptosis-suppressor, and ferroptosis-marker genes, were remarkably different between the 2 groups of the CRs (Figure 7B-7D).
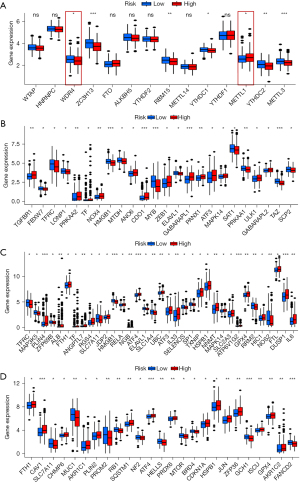
Drug sensitivity prediction
Based on the pRRophetic algorithm, we explored the relationship between risk score and chemoresistance by calculating the IC50 of the 7 common chemotherapeutic agents (elesclomol, sorafenib, DMOG, epothilone B, GDC0941, embelin, and FH535) for GC. The results showed that the IC50 value of these drugs in the high-risk group of the CRs was greater than that in the low-risk group and had a statistically significant value (P<0.05), suggesting that the prognosis model of CRs was related to drug sensitivity in patients with GC (Figure 8A-8G).
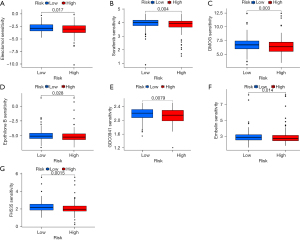
Single-cell sequencing analysis
The scRNA-seq data were used to identify cell clusters and the expression of 12 CRGs in different cell clusters using the tSNE algorithm. The DLAT, FDX1, and LIPT1 genes were upregulated in cluster 0; ATP7B, PDHA1, PDHB, and GCSH were upregulated in clusters 1 and 4; and SLC31A1 was upregulated in cluster 5 (Figure 9A,9B). The cell annotation results showed that clusters 0, 1, 2, 3, and 4 were composed of endothelial cells, whereas cluster 5 was composed of macrophages (Table S2). KEGG pathway enrichment analysis (https://cdn.amegroups.cn/static/public/jgo-23-62-2.xlsx) in ClueGO indicated that the genes of cluster 0 were primarily enriched in the following signaling pathways: epithelial cell signaling in HP infection; interactions of viral proteins with cytokine receptor and cytokine; and NF-κB, interleukin 17 (IL-17), NOD-like receptor, and TNF signaling pathways (Figure 9C). The genes in cluster 1 were mainly involved in protein digestion and absorption, complement and coagulation cascades, IL-17 signaling pathway, epithelial cell signaling, the apelin signaling pathway, and ECM-receptor interaction (Figure 9D). The cluster 2 genes were primarily enriched in the Huntington disease, prion disease, and neurodegeneration pathways (Figure 9E). Cluster 3 genes were linked to mineral absorption, glioma, fluid shear stress, and atherosclerosis (Figure 9F). The genes in cluster 4 were mainly involved in the proteasome, ribosome, coronavirus disease, oxidative phosphorylation, cardiac muscle contraction, and thermogenesis (Figure 9G). The genes of cluster 5 were primarily concentrated in immune-related signaling pathways, including the NF-κB signaling pathway; antigen processing and presentation; TNF signaling pathway; inflammatory bowel disease; B-cell receptor signaling pathway; toll-like receptor signaling pathway; chemokine signaling pathway; and the intestinal immune network for immunoglobin A (IgA) production, and T helper type 1 (Th1), Th2, and Th17 cell differentiation (Figure 9H).
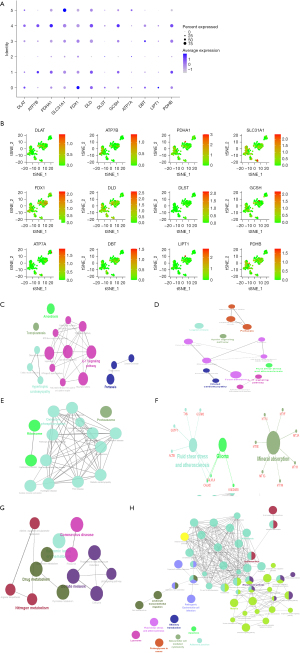
Discussion
Cuproptosis involves a novel type of cell death caused by toxic copper-dependent protein stress. Many copper ion carriers are widely used as antitumor drugs, such as quinolin-8-ol, flavonoids, and carbamate (20-22). Hence, cuproptosis might be a breakthrough strategy for cancer therapy. Based on TCGA and GEO scRNA data sets, we first identified 8 DE-CRGs in GC. There were 9 CRGs with TMB values >1%. We then examined the correlation between the 2 sets of the CRs and tumor-infiltrating immune cells, immunological function, and m6A-, m7G, and ferroptosis-related gene expressions. Finally, the single-cell sequencing was performed to assess the expression of these CRGs in different clusters and explore their cellular functions. Overall, we found potential biomarkers and functions related to cuproptosis in GC.
Previous study has shown that most cancer cells prefer glycolysis over oxygen circulation, a process known as the Warburg effect (23). Here, we showed that CRGs, such as DLAT, PDHA1, and DLD, were primarily engaged in glycolysis/gluconeogenesis and oxidative phosphorylation pathway. This suggested that the CRGs might influence the development of GC by regulating the Warburg effect. Additionally, cancer cell proliferation is inhibited without the electron receptors provided by respiration. Tumor cells often lack the ability to de novo synthesize L-asparagine (24). In this case, pyruvate serves as an additional electron acceptor in the respiratory metabolism, and the promotion of cancer cells can be promoted by using the extra pyruvate to generate L-aspartic acid (25). In the present study, DLD was involved in the pyruvate metabolic pathway. Hence, by converting lactic acid from glycolysis to pyruvate, DLD might boost cancer cell growth. Some studies have found that the mitochondrial respiration of cancer cells also requires electron-accepting cofactors, such as nicotinamide adenine dinucleotide (NAD) and flavin adenine dinucleotide (FAD) (26,27). Based on our previous findings, we postulated that CRGs, such as DLAT, PDA1, and DLD, are involved in GC cell hyperproliferation via glycolysis.
In the present study, DE-CRGs, including DLAT, PDHA1, and DLD, were found to be involved in mitochondrial electron transport, NAD binding process, and oxidoreductase activity acting on NAD(P)H. Moreover, these genes were enriched in the TCA cycle and iron–sulfur cluster binding. Recent study has shown that copper ions can cause protein stress and downregulation of iron-sulfur cluster proteins by binding to the fatty acylated components of the TCA cycle in mitochondrial respiration, ultimately leading to cell death. Copper binding to the lipid-acylated TCA cyclin leads to oligomerization of DLAT (13). Moreover, the inactivation of PDHA1 results in the decrease of TCA cycle intermediates and affects ATP and acetyl-CoA levels as well as the NADH/NAD ratio (28). DLD is the component of the pyruvate dehydrogenase complex that catalyzes the conversion of pyruvate into the precursor for the TCA cycle (29). These characteristics are consistent with our current results.
Furthermore, we constructed a CRs consisting of AL512506.1, AC016737.1, AC090204.1, AP001363.2, TYMSOS, and AL353804.2. The GC cases with high-risk scores lived for a shorter time than did those with low-risk scores. Compared with other models (30,31), this paper found that CRs have independent GC prognostic characteristics through univariate and multivariate cox regression analysis, and can be used as an independent prognostic factor of gastric cancer. Risk score is unique and sensitive in predicting the prognosis of patients with gastric cancer (Figure 4A,4B). The three-year AUC of risk score (AUC >0.7) is also higher than other clinicopathological features, indicating that CRs is a reliable prognostic risk model for gastric cancer. Compared to age, gender, grade, and stage, the CRs demonstrated high predictive ability and applicability in clinic. It has been found that TYMSOS is linked not only to a poor outcomes for those with non-small cell lung cancer, but also to the proliferation, migration, and invasion of GC (32). Recent study has found that AL512506.1 and AC016737.1 are independent prognostic factors for GC (33), which consistent with our current results. Hence, developing a CRs model is critical for GC. AL512506.1 and AC016737.1 are also inflammation-related lncRNAs that have an important function in regulating the development of innate immune cells and the expression of inflammatory genes (34). These lncRNAs might also play a major role in converting inflammation into malignancy. Thus, we hypothesized that the CRs could be linked to antitumor immune processes. Therefore, we analyzed the relation between tumor immune infiltration and the CRs. We detected significant differences in MHC class I, type II INF responders, and the levels of multiple immune checkpoints between the 2 groups of the CRs.
Moreover, we performed GSEA to analyze the molecular functions of different subcohorts of the CRs. The high-risk group was mainly enriched for angiogenesis, and important metabolic pathways, such as ECM-receptor interaction, vascular smooth muscle contraction, focal adhesion, glycosaminoglycan biosynthesis-keratan sulfate, TGF-beta signaling pathway, and the metabolism of xenobiotics by cytochrome P450. ECM HAPL1N1 protein remodeling promotes lymph node metastasis in melanoma (35), while ECM stiffness stimulates tumor angiogenesis (36,37). The TGF-β signaling pathway is well known for its role in carcinogenesis and progression, promoting tumor migration and invasion. 1α,25-dihydroxyvitamin D3 upregulates the TGF-β/Smad2/3 signaling pathway, which promotes angiogenesis in rats (38). Cytochrome P450 induces mutations and cell transformation by covalent binding of DNA with electrophilic intermediates produced by the activation of numerous carcinogenic chemicals, ultimately leading to cancer development (39). Therefore, we hypothesized that the poor outcome of those with high-risk GC was associated with the activation of these pathways, which promote GC development.
Previous study has found that m6A methylation can interfere with the antitumor effect of copper chelating agents. For example, PRPF6 m6A methylation inhibits the anticancer effects induced by copper chelating agents (40). Additionally, m7G is a posttranslational modification of mRNAs and lncRNAs in eukaryotic cells that is critical for effective gene expression and cell viability (41). Here, we showed that the CRs was related to m6A methylation regulatory genes including RBM15, YTHDC1, YTHDC2, and METTL3, as well as m7G regulatory genes, including METTL1 and WDR4. The Study has also revealed that m6A methyltransferase METTL3 affects autophagy and progression of nasopharyngeal carcinoma by regulating the stability of the lncRNA ZFAS1 (42). This indicates that m6A and m7G methylation mechanisms might be related to cuproptosis. Additionally, other study has found that DSF/Cu can cause iron-like cell death by severely impairing mitochondrial homeostasis and enhancing lipid peroxidation. DSF/Cu combined with sorafenib can effectively inhibit tumor growth in vivo (43). Additionally, the loss of fumarase mitigates ferroptosis by interfering with the TCA cycle (44). Finally, the downstream metabolic products of the TCA cycle can trigger ferroptosis (45).
Copper ions can bind to the fatty acylated components in the TCA cycle, resulting in protein toxicity stress, ultimately leading to cuproptosis. The mechanisms of ferroptosis and cuproptosis are related to mitochondrial components and the TCA cycle. However, the relationship between ferroptosis and cuproptosis has not been studied. In our study, we found that the CRs was highly associated with the most ferroptosis-driven, ferroptosis-marker, and ferroptosis-suppressor genes. Hence, ferroptosis and cuproptosis might be linked by lncRNAs. Moreover, previous study has found that endothelial cells are involved in tumor angiogenesis and can determine disease prognoses (46), which is consistent with our findings. In this study, we analyzed the drug sensitivity of the copper-based carrier drug elesclomol and found that the drug sensitivity differed between the high- and low-risk groups. The ClueGO and scRNA-seq analyses demonstrated that the marker genes in endothelial cells were enriched in angiogenesis-related pathways, including in protein digestion and absorption, ECM-receptor interaction, NF-κB signaling pathway, and focal adhesion. NF-κB triggers endothelial cells that affect the tumor microenvironment and promote tumor angiogenesis (47). The scRNA-seq analysis revealed that 11 of the 12 CRGs were upregulated in endothelial cells, indicating that the CRGs might be involved in angiogenesis. Finally, the high-risk group of the CRs was associated with some critical angiogenesis pathways. Therefore, CRGs might have a potential role in angiogenesis via lncRNAs.
Conclusions
The CRs was demonstrated to be a valuable independent prognostic factor that can predict the prognosis of GC. The signature risk score was related to immune cell content and function, as well as the levels of immune checkpoints, m6A-, m7G-, and ferroptosis-related genes.
Acknowledgments
We thank the TCGA and GEO databases for providing their platforms and their contributors for uploading their valuable data sets.
Funding: This work was supported by the National Natural Science Foundation of China (No. 32060159) and Guangxi Natural Science Foundation (No. 2019JJA140462).
Footnote
Reporting Checklist: The authors have completed the TRIPOD reporting checklist. Available at https://jgo.amegroups.com/article/view/10.21037/jgo-23-62/rc
Conflicts of Interest: All authors have completed the ICMJE uniform disclosure form (available at https://jgo.amegroups.com/article/view/10.21037/jgo-23-62/coif). The authors have no conflicts of interest to declare.
Ethical Statement: The authors are accountable for all aspects of the work in ensuring that questions related to the accuracy or integrity of any part of the work are appropriately investigated and resolved. The study was conducted in accordance with the Declaration of Helsinki (as revised in 2013).
Open Access Statement: This is an Open Access article distributed in accordance with the Creative Commons Attribution-NonCommercial-NoDerivs 4.0 International License (CC BY-NC-ND 4.0), which permits the non-commercial replication and distribution of the article with the strict proviso that no changes or edits are made and the original work is properly cited (including links to both the formal publication through the relevant DOI and the license). See: https://creativecommons.org/licenses/by-nc-nd/4.0/.
References
- Sung H, Ferlay J, Siegel RL, et al. Global Cancer Statistics 2020: GLOBOCAN Estimates of Incidence and Mortality Worldwide for 36 Cancers in 185 Countries. CA Cancer J Clin 2021;71:209-49. [Crossref] [PubMed]
- Howson CP, Hiyama T, Wynder EL. The decline in gastric cancer: epidemiology of an unplanned triumph. Epidemiol Rev 1986;8:1-27. [Crossref] [PubMed]
- Arnold M, Park JY, Camargo MC, et al. Is gastric cancer becoming a rare disease? A global assessment of predicted incidence trends to 2035. Gut 2020;69:823-9. [Crossref] [PubMed]
- Heer EV, Harper AS, Sung H, et al. Emerging cancer incidence trends in Canada: The growing burden of young adult cancers. Cancer 2020;126:4553-62. [Crossref] [PubMed]
- Infection with Helicobacter pylori. IARC Monogr Eval Carcinog Risks Hum 1994;61:177-240.
- Plummer M, Franceschi S, Vignat J, et al. Global burden of gastric cancer attributable to Helicobacter pylori. Int J Cancer 2015;136:487-90. [Crossref] [PubMed]
- Zhang Y, Yan J, Li C, et al. LncRNA H19 induced by helicobacter pylori infection promotes gastric cancer cell growth via enhancing NF-κB-induced inflammation. J Inflamm (Lond) 2019;16:23. [Crossref] [PubMed]
- Esposito R, Vllahu M, Morello S, et al. Low copper availability limits Helicobacter infection in mice. FEBS J 2020;287:2948-60. [Crossref] [PubMed]
- Voli F, Valli E, Lerra L, et al. Intratumoral Copper Modulates PD-L1 Expression and Influences Tumor Immune Evasion. Cancer Res 2020;80:4129-44. [Crossref] [PubMed]
- Liu YL, Bager CL, Willumsen N, et al. Tetrathiomolybdate (TM)-associated copper depletion influences collagen remodeling and immune response in the pre-metastatic niche of breast cancer. NPJ Breast Cancer 2021;7:108. [Crossref] [PubMed]
- Ma Q, Hui Y, Huang BR, et al. Ferroptosis and cuproptosis prognostic signature for prediction of prognosis, immunotherapy and drug sensitivity in hepatocellular carcinoma: development and validation based on TCGA and ICGC databases. Transl Cancer Res 2023;12:46-64. [Crossref] [PubMed]
- Janjetic MA, Goldman CG, Balcarce NE, et al. Iron, zinc, and copper nutritional status in children infected with Helicobacter pylori. J Pediatr Gastroenterol Nutr 2010;51:85-9. [Crossref] [PubMed]
- Tsvetkov P, Coy S, Petrova B, et al. Copper induces cell death by targeting lipoylated TCA cycle proteins. Science 2022;375:1254-61. [Crossref] [PubMed]
- Aubert L, Nandagopal N, Steinhart Z, et al. Copper bioavailability is a KRAS-specific vulnerability in colorectal cancer. Nat Commun 2020;11:3701. [Crossref] [PubMed]
- Liu Y, Guan X, Wang M, et al. Disulfiram/Copper induces antitumor activity against gastric cancer via the ROS/MAPK and NPL4 pathways. Bioengineered 2022;13:6579-89. [Crossref] [PubMed]
- Du C, Guan X, Liu Y, et al. Disulfiram/copper induces antitumor activity against gastric cancer cells in vitro and in vivo by inhibiting S6K1 and c-Myc. Cancer Chemother Pharmacol 2022;89:451-8. [Crossref] [PubMed]
- Wang J, Qin D, Tao Z, et al. Identification of cuproptosis-related subtypes, construction of a prognosis model, and tumor microenvironment landscape in gastric cancer. Front Immunol 2022;13:1056932. [Crossref] [PubMed]
- Tang X, Guo T, Wu X, et al. Clinical Significance and Immune Infiltration Analyses of the Cuproptosis-Related Human Copper Proteome in Gastric Cancer. Biomolecules 2022;12:1459. [Crossref] [PubMed]
- Nie H, Wang H, Zhang M, et al. Comprehensive analysis of cuproptosis-related genes in prognosis, tumor microenvironment infiltration, and immunotherapy response in gastric cancer. J Cancer Res Clin Oncol 2022; Epub ahead of print. [Crossref]
- Denoyer D, Masaldan S, La Fontaine S, et al. Targeting copper in cancer therapy: 'Copper That Cancer'. Metallomics 2015;7:1459-76. [Crossref] [PubMed]
- Hunsaker EW, Franz KJ. Copper potentiates azole antifungal activity in a way that does not involve complex formation. Dalton Trans 2019;48:9654-62. [Crossref] [PubMed]
- Lelièvre B, Drouillard I, Thill C, et al. Severe poisoning with naproxen causing coagulopathy. Basic Clin Pharmacol Toxicol 2020;126:458-63. [Crossref] [PubMed]
- Liberti MV, Locasale JW. Correction to: 'The Warburg Effect: How Does it Benefit Cancer Cells?' Trends Biochem Sci 2016;41:287. [Trends in Biochemical Sciences, 41 (2016) 211].
- Pieters R, Appel I, Kuehnel HJ, et al. Pharmacokinetics, pharmacodynamics, efficacy, and safety of a new recombinant asparaginase preparation in children with previously untreated acute lymphoblastic leukemia: a randomized phase 2 clinical trial. Blood 2008;112:4832-8. [Crossref] [PubMed]
- Vyas S, Zaganjor E, Haigis MC. Mitochondria and Cancer. Cell 2016;166:555-66. [Crossref] [PubMed]
- Birsoy K, Wang T, Chen WW, et al. An Essential Role of the Mitochondrial Electron Transport Chain in Cell Proliferation Is to Enable Aspartate Synthesis. Cell 2015;162:540-51. [Crossref] [PubMed]
- Carey BW, Finley LW, Cross JR, et al. Intracellular alpha-ketoglutarate maintains the pluripotency of embryonic stem cells. Nature. 2015;518:413-6. [Crossref] [PubMed]
- Chen J, Guccini I, Di Mitri D, et al. Compartmentalized activities of the pyruvate dehydrogenase complex sustain lipogenesis in prostate cancer. Nat Genet 2018;50:219-28. [Crossref] [PubMed]
- Komurov K. Modeling community-wide molecular networks of multicellular systems. Bioinformatics 2012;28:694-700. [Crossref] [PubMed]
- Liu Y, Huang Z, Cheng G, et al. Development of a four-gene prognostic model for clear cell renal cell carcinoma based on transcriptome analysis. Genomics 2021;113:1816-27. [Crossref] [PubMed]
- He L, Chen J, Xu F, et al. Prognostic Implication of a Metabolism-Associated Gene Signature in Lung Adenocarcinoma. Mol Ther Oncolytics 2020;19:265-77. [Crossref] [PubMed]
- Yuan Y, Jiang X, Tang L, et al. FOXM1/lncRNA TYMSOS/miR-214-3p-Mediated High Expression of NCAPG Correlates With Poor Prognosis and Cell Proliferation in Non-Small Cell Lung Carcinoma. Front Mol Biosci 2021;8:785767. [Crossref] [PubMed]
- Zhang S, Li X, Tang C, et al. Inflammation-Related Long Non-Coding RNA Signature Predicts the Prognosis of Gastric Carcinoma. Front Genet 2021;12:736766. [Crossref] [PubMed]
- Elling R, Chan J, Fitzgerald KA. Emerging role of long noncoding RNAs as regulators of innate immune cell development and inflammatory gene expression. Eur J Immunol 2016;46:504-12. [Crossref] [PubMed]
- Ecker BL, Kaur A, Douglass SM, et al. Age-Related Changes in HAPLN1 Increase Lymphatic Permeability and Affect Routes of Melanoma Metastasis. Cancer Discov 2019;9:82-95. [Crossref] [PubMed]
- Bordeleau F, Califano JP, Negrón Abril YL, et al. Tissue stiffness regulates serine/arginine-rich protein-mediated splicing of the extra domain B-fibronectin isoform in tumors. Proc Natl Acad Sci U S A 2015;112:8314-9. [Crossref] [PubMed]
- Kretschmer M, Rüdiger D, Zahler S. Mechanical aspects of angiogenesis. Cancers (Basel) 2021;13:4987. [Crossref] [PubMed]
- Zhang Y, Mu Y, Ding H, et al. 1α,25-Dihydroxyvitamin D3 promotes angiogenesis after cerebral ischemia injury in rats by upregulating the TGF-β/Smad2/3 signaling pathway. Front Cardiovasc Med 2022;9:769717. [Crossref] [PubMed]
- Abu-Bakar A, Tan BH, Halim H, et al. Cytochromes P450: role in carcinogenesis and relevance to cancers. Curr Drug Metab 2022;23:355-73. [Crossref] [PubMed]
- Li X, Li N, Huang L, et al. Is hydrogen sulfide a concern during treatment of lung adenocarcinoma with ammonium tetrathiomolybdate? Front Oncol 2020;10:234. [Crossref] [PubMed]
- Tomikawa C. 7-Methylguanosine Modifications in Transfer RNA (tRNA). Int J Mol Sci 2018;19:4080. [Crossref] [PubMed]
- Ren X, Li Y, Zhou Y, et al. Overcoming the compensatory elevation of NRF2 renders hepatocellular carcinoma cells more vulnerable to disulfiram/copper-induced ferroptosis. Redox Biol 2021;46:102122. [Crossref] [PubMed]
- Stockwell BR, Jiang X, Gu W. Emerging mechanisms and disease relevance of ferroptosis. Trends Cell Biol 2020;30:478-90. [Crossref] [PubMed]
- Kumar VK, Lackey A, Snyder J, et al. Mitochondrial Membrane Intracellular Communication in Healthy and Diseased Myocardium. Front Cell Dev Biol 2020;8:609241. [Crossref] [PubMed]
- Maiborodin I, Mansurova A, Chernyavskiy A, et al. Cancer angiogenesis and opportunity of influence on tumor by changing vascularization. J Pers Med 2022;12:327. [Crossref] [PubMed]
- Ekambaram P, Lee JL, Hubel NE, et al. The CARMA3-Bcl10-MALT1 Signalosome Drives NFκB Activation and Promotes Aggressiveness in Angiotensin II Receptor-Positive Breast Cancer. Cancer Res 2018;78:1225-40. [Crossref] [PubMed]
- Bauer M, Nascakova Z, Mihai AI, et al. The ALPK1/TIFA/NF-κB axis links a bacterial carcinogen to R-loop-induced replication stress. Nat Commun 2020;11:5117. [Crossref] [PubMed]
(English Language Editor: J. Gray)