Screening of potential pain genes in pancreatic ductal adenocarcinoma (PDAC) based on bioinformatics methods
Highlight box
Key findings
• The dozens of secretory proteomics screened in this study provide important basis for the pain treatment of patients with PDAC tumors.
What is known and what is new?
• The cancerous pain of PDAC puzzles clinicians. It is of great significance to search for cancer-related pain genes.
• PTGS2, VCAN and CCL2 are directly related to the occurrence of cancer pain and can provide therapeutic targets for the treatment of PDAC.
What is the implication, and what should change now?
• Some potential pain-causing genes and signaling pathways have been identified through the analysis of PDAC expression data, and bioinformatics provides a simple and easy method to identify target genes.
Introduction
In clinical practice, pancreatic ductal adenocarcinoma (PDAC) is among the most prevalent gastrointestinal tumors. Early diagnosis of PDAC is difficult. When diagnosed, most patients have already reached the middle or late stages of the disease, and the prognosis is dismal. Less than 5% of individuals survive beyond five years, and 90% of patients die within 1 year of diagnosis (1,2). Despite improvements in treatment, PDAC’s 5-year survival rate has not shown similar improvement (3,4). The recent advances in science and technology and continuing research development have identified that approximately 5–10% of PDAC patients have a genetic background (5,6). Not only is the high mortality rate of PDAC a concern for clinicians, but also the cancer pain it causes has a significant impact on patients’ quality of life.
Cancer pain is bodily pain caused by the occurrence, development, and metastasis of cancer. Cancer occurrence has been proven to be related to oncogenes and is accompanied by physical pain (7,8). Approximately 30–50% of patients experience cancer pain in the early and middle stages and 75–90% experience pain in the advanced stages (9). Although some researchers have confirmed that 70–90% of cancer patients can receive some pain relief from effective treatment, about 30% of cancer patients suffer from severe pain that cannot be effectively treated (10). In these patients, the complex pain occurring in the late stage of cancer is an important factor leading to uncontrolled pain. Therefore, the creation of novel analgesic medicines is urgently required to provide palliative care that relieves pain and improves the quality of life for PDAC cancer patients. This requires researchers to focus on the tumor itself, especially the series of exocrine proteins secreted and released by the tumor because they are the bridge between tumors and nociceptors.
Tumor genomics, proteomics, epigenetics, and other studies have produced a significant amount of mutation and gene expression data. This study aimed to use bioinformatics technology to mine the gene expression of PDAC-secreted histones in the Gene Expression Omnibus (GEO) database to identify the pain-causing genes in tumors and explore the feasibility of using bioinformatics to mine omics data to identify new molecular targets. We present the following article in accordance with the STREGA reporting checklist (available at https://jgo.amegroups.com/article/view/10.21037/jgo-23-94/rc).
Methods
GEO data analysis
The GEOquery package was used to download the RNA expression dataset GSE50570 from the GEO database (https://www.ncbi.nlm.nih.gov/geo/query/acc.cgi), and then the probes that related to multiple molecules were eliminated. Only the probe with the highest signal value was kept when multiple probes for the same molecule were encountered. The study was conducted in accordance with the Declaration of Helsinki (as revised in 2013).
Differential expression analysis
GEO2R is an online tool for analyzing differential expression. T-tests were used in differential gene expression (DGE) analyses to determine P values and adjusted P values. The following criteria were employed in the selection of genes retained from various tissues: |log2(fold-change)| >1, adjusted P value <0.05. Additionally, we acquired a dataset of related genes from the human secretome, which we intersected with GSE50570 to identify the associated differentially expressed genes (DEGs). A Venn diagram of DEGs was created using the Venny 2.1 online tool (https://bioinfogp.cnb.csic.es/tools/venny/index.html), and a heat map of DEGs was created using Rsoftware.
Gene Ontology (GO) and Kyoto Encyclopedia of Genes and Genomes (KEGG) pathway enrichment analysis and gene set enrichment analysis (GSEA)
The biological roles of the differentially expressed genes in PDAC were investigated using GO and KEGG analyses. A sophisticated bioinformatics tool called GO analysis was employed to locate the biological processes (BPs), cellular components (CCs), and molecular functions (MFs) related to differentially expressed genes.
Protein-protein interaction (PPI) network analysis of common differentially expressed genes
For predicting PPI, we retrieved the interactions between these proteins through the STRING database. Additionally, we used Cytoscape v3.6.0 software (https://cytoscape.org/) to construct and visualize the PPI network. Key PPI network modules were identified by a cluster analysis of gene networks using the molecular combinatorial detection (MCODE) method. MCODE’s role is to choose important sub-networks, termed modules. A module that points to a function is referred to as a PPI module. Various genes in a module have different module scores, and essential genes could be chosen based on the scores. A significant difference was deemed to exist using a P value <0.05 to identify essential modules.
Statistical analysis
We used GraphPad Prism 7.0 software (https://www.graphpad.com/scientific-software/prism/) to create graphs and perform the statistical analysis. The data are presented as means ± standard deviations (SDs). T-tests were performed to analyze DGEs and ascertain P values. We used false discovery rate (FDR)-adjusted P values, where a P value <0.05 indicated a statistically significant difference.
Results
Identification of differentially expressed genes
The microarray expression profiling dataset GSE50570 was downloaded from the GEO database, and a volcano map (Figure 1A), principal component analysis (PCA) map (Figure 1B), box plot (Figure 1C), and heat map (Figure 1D) were created. Then, we constructed a Venn diagram of these differentially expressed genes and corresponding genes from the human secretome. We found that 12 genes corresponding to secretory proteins were upregulated in PDAC (Figure 1E) (see Table 1 for details), and 81 genes corresponding to the secreted proteins were downregulated in PDAC (Figure 1F) (see Table 2 for details). The differences in the expression of these 93 genes in PDAC and their significance are illustrated by a volcano plot (Figure 2).
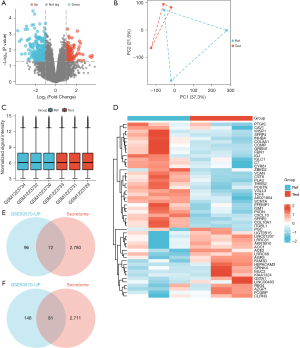
Table 1
FCGBP | AGR3 | RBP4 | SCGB2A1 |
ANG | BPIFB1 | ABAT | CYP2S1 |
ENTPD5 | MUC5AC | F5 | UGT2B28 |
PDAC, pancreatic ductal adenocarcinoma.
Table 2
SFRP2 | THBS2 | COMP | COL8A1 | VCAN | CST6 | CXCL10 | GREM1 | COL10A1 | C7 |
EFEMP1 | SFRP4 | ITGBL1 | PLAU | POSTN | ISM1 | INHBA | PXDN | FBN1 | COL6A3 |
COL1A2 | LTBP2 | COL5A2 | IGFBP5 | FN1 | BGN | GFPT2 | CDH11 | PTGS2 | IGFBP3 |
ROBO1 | SULF1 | COL5A1 | FSTL3 | COL1A1 | DSE | ISLR | C1S | ADAMTS2 | TNS1 |
MOXD1 | CCL2 | COL6A1 | LAMB1 | COL4A1 | PRELP | AEBP1 | EFEMP2 | CXCL8 | SRPX2 |
BOC | PAPLN | PTGDS | SERPINE1 | LOXL4 | FSTL1 | OLFML2A | GPNMB | IL7R | COL3A1 |
LTBP1 | TGFB2 | LYPD1 | COL4A2 | IGLV1-44 | PDGFRA | SLIT2 | LAMC2 | GLS | TIMP2 |
IGFBP7 | JAM3 | LAMA2 | COL16A1 | TMEM119 | PCOLCE | DPEP1 | MFAP2 | ESM1 | APOD |
LOXL2 |
PDAC, pancreatic ductal adenocarcinoma.
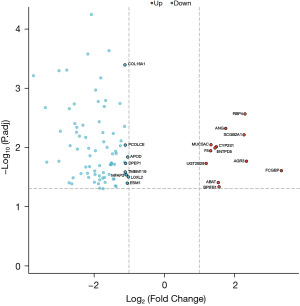
GO and KEGG enrichment analyses
We used the DAVID online database to conduct the enrichment analysis of the identified common differentially expressed genes compared with human secretory proteome, and the GO enrichment information was obtained. The “biological processes” enriched by these differential genes mainly include extracellular structure organization, extracellular matrix, signal pathway cell matrix adhesion, and response to amino acids (Figure 3A). Differential genes are primarily abundant in the extracellular matrix comprising collagen, endoplasmic reticulum lumen, basement membrane, collagen trimer complex, vesicle, cytoplasmic vesicle, and secretory granule lumen, among other “cell composition” components (Figure 3B). In terms of “molecular function”, it is suggested that the differential genes are mainly related to endopeptidase regulation activity, protease binding, platelet-derived growth factor binding, collagen binding, copper ion binding, proteoglycan binding fibronectin binding, and insulin-like growth factor binding (Figure 3C). According to the KEGG enrichment analysis, these differentially expressed genes may affect PDAC’s various cancer pain manifestations by being involved in the PI3K-Akt signaling pathway, digestion and absorption of protein, extracellular matrix (ECM) receptor interaction, AGE-RAGE (advanced glycation endproducts-the Receptor of Advanced Glycation Endproducts) signaling pathway, relaxin signaling pathway, interleukin-17 (IL-17) signaling pathway, and transforming growth factor-β (TGF-β) signaling pathway, among other pathways (Figure 3D).
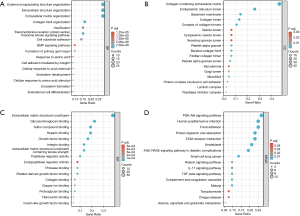
Protein interaction network construction and module analysis of common differentially expressed genes and core gene screening
We used the STRING database to construct the protein interaction networks for common differentially expressed genes (Figure 4A). The results were imported into Cytoscape software for the visual analysis and identification of the protein interaction networks. We used the Network Analyzer tool in Cytoscape to calculate the non-directional score of each node in the protein interaction network and obtain the degree of each node. We used the MCODE plug-in to cluster these important protein molecules with the default parameters of Node Score Cutoff =0.2, K-core =2, and Max Depth =100. According to the association analysis, three clusters with higher scores were obtained, namely Cluster1 (Figure 4B), Cluster2 (Figure 4C), and Cluster3 (Figure 4D).
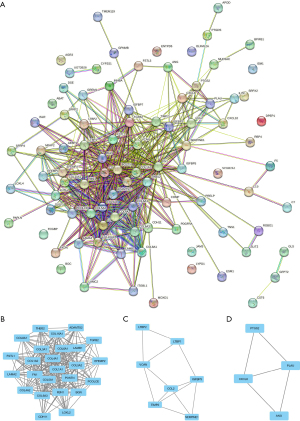
Discussion
The advancement of modern science and technology and the development of medical technology have greatly extended the survival of cancer patients. However, the complex pain caused by the tumor itself remains a serious problem. The International Pain Research Society has stated that pain is the fifth vital sign after blood pressure, pulse, respiration, and body temperature. As a long-term symptom, cancer pain has affected patients and their family members in terms of physiology, psychology, spirit, and quality of life (11). Studying the genes associated with PDAC cancer pain will therefore have significant clinical implications and will help to provide a foundation for PDAC patients’ pain management.
In this study, the latest bioinformatics combined with big data tumor analysis methods were utilized to perform the GO functional annotation, KEGG pathway enrichment, PPI network diagram, key gene screening, and key gene determination on the GSE50570 chip data downloaded from the GEO database. We first performed a differential analysis on the GSE50570, followed by intersection with corresponding genes from the human secretome to identify associated DEGs. A total of 12 upregulated and 81 downregulated genes were found by constructing a Venn diagram. Some of the top 20 most differentially expressed genes have been previously studied in tumor-related pain. For example, inflammatory mononuclear cells (Iba-1+ CCR2+ Ly6C+ TMEM119− cells) function in neuropathic pain (12); cancer-related bone pain is markedly reduced by Ang-(1-7)/MasR activation without many of the adverse effects associated with opioids. Therefore, for approximately 90% of advanced cancer patients who experience significant pain, Ang-(1-7) may be an alternate therapy option (13); the ABAT gene on chromosome 16 contains an exon single nucleotide polymorphism (SNP) (rs1641025) that might be a possible candidate locus for response to opioid analgesics, and in the development of opioid analgesic-adjunct drug therapy, γ-aminobutyric acid (GABA) transaminase might be a potential target (14).
Our GO and KEGG enrichment analysis found that these differentially expressed genes may be involved in the PI3K-Akt signaling pathway, digestion and absorption of protein, ECM receptor interaction, AGE-RAGE signaling pathway, relaxin signaling pathway, IL-17 signaling pathway, and TGF-β signaling pathway, thus affecting the different manifestations of PDAC cancer pain. There are also related studies to support these possible mechanism pathways. For example, morphine inhibits the inflammatory microenvironment via the PI3K-Akt-NF-κB pathway that promotes chronic tibial cancer pain (15); electroacupuncture inhibits the PI3K/Akt/JNK1/2 signaling pathway that weakens the tolerance to morphine in rats with bone cancer pain (16); inhibition of bone cancer pain by Long-Teng-Tong-Luo (LTTL) gel in a rat model involves transient receptor potential channels and interleukin 17A (17); and from a neuroinflammation perspective, IL-17 is a potential therapeutic target for chronic pain (18). These previous studies also reflect the credibility and clinical value of our research results. Finally, we further identified the core genes by constructing a protein interaction network and module analysis of common differentially expressed genes. In this study, three clusters with high scores were obtained, namely Cluster1, Cluster2, and Cluster3. Some key genes in these clusters have also been studied in cancer pain, such as SNPs in PTGS2 and LTA, which have been shown to predict long-term lung cancer survivors’ pain and quality of life (19); regulatory VCAN polymorphisms are related to shoulder pain and disability in breast cancer survivors (20); and the analgesic effect of rolipram is associated with the inhibition of spinal cord astrocyte Jun amino-terminal kinase (JNK) bone cancer pain/CCL2 pathway activation (21). These studies provide a good reference for further in vivo and in vitro experiments in the future.
The present investigation was mainly based on the screening of omics data and lacked verification based on experimental animal data, which is the main limitation of this study. Our literature review revealed that the main findings of this study were also verified by previous research, indicating that the use of bioinformatics technology to mine omics data can be used as an effective method for identifying target genes. However, whether the dozens of secretome genes identified in the present investigation take part in the occurrence and maintenance of PDAC cancer pain requires further experimental verification.
Conclusions
In summary, some potential pain-causing genes and signaling pathways have been identified through the analysis of PDAC expression data, and bioinformatics provides a simple and easy method to identify target genes.
Acknowledgments
Funding: None.
Footnote
Reporting Checklist: The authors have completed the STREGA reporting checklist. Available at https://jgo.amegroups.com/article/view/10.21037/jgo-23-94/rc
Peer Review File: Available at https://jgo.amegroups.com/article/view/10.21037/jgo-23-94/prf
Conflicts of Interest: All authors have completed the ICMJE uniform disclosure form (available at https://jgo.amegroups.com/article/view/10.21037/jgo-23-94/coif). The authors have no conflicts of interest to declare.
Ethical Statement: The authors are accountable for all aspects of the work in ensuring that questions related to the accuracy or integrity of any part of the work are appropriately investigated and resolved. The study was conducted in accordance with the Declaration of Helsinki (as revised in 2013).
Open Access Statement: This is an Open Access article distributed in accordance with the Creative Commons Attribution-NonCommercial-NoDerivs 4.0 International License (CC BY-NC-ND 4.0), which permits the non-commercial replication and distribution of the article with the strict proviso that no changes or edits are made and the original work is properly cited (including links to both the formal publication through the relevant DOI and the license). See: https://creativecommons.org/licenses/by-nc-nd/4.0/.
References
- Kardosh A, Lichtensztajn DY, Gubens MA, et al. Long-Term Survivors of Pancreatic Cancer: A California Population-Based Study. Pancreas 2018;47:958-66. [Crossref] [PubMed]
- Neesse A, Bauer CA, Öhlund D, et al. Stromal biology and therapy in pancreatic cancer: ready for clinical translation? Gut 2019;68:159-71. [Crossref] [PubMed]
- Wasif N, Ko CY, Farrell J, et al. Impact of tumor grade on prognosis in pancreatic cancer: should we include grade in AJCC staging? Ann Surg Oncol 2010;17:2312-20. [Crossref] [PubMed]
- Meng Y, Li Y, Fang D, et al. Identification of solute carrier family genes related to the prognosis and tumor-infiltrating immune cells of pancreatic ductal adenocarcinoma. Ann Transl Med 2022;10:57. [Crossref] [PubMed]
- Klatte DCF, Wallace MB, Löhr M, et al. Hereditary pancreatic cancer. Best Pract Res Clin Gastroenterol 2022;58-59:101783. [Crossref] [PubMed]
- Hayashi A, Hong J, Iacobuzio-Donahue CA. The pancreatic cancer genome revisited. Nat Rev Gastroenterol Hepatol 2021;18:469-81. [Crossref] [PubMed]
- Bao YJ, Hua BJ, Hou W, et al. Alleviation of cancerous pain by external compress with Xiaozheng Zhitong Paste. Chin J Integr Med 2010;16:309-14. [Crossref] [PubMed]
- Li L, Su H, Yang Y, et al. Screening key genes related to neuropathic pain-induced depression through an integrative bioinformatics analysis. Ann Transl Med 2022;10:1348. [Crossref] [PubMed]
- Gao L, Yang YJ, Xu HY, et al. A randomized clinical trial of nerve block to manage end-stage pancreatic cancerous pain. Tumour Biol 2014;35:2297-301. [Crossref] [PubMed]
- Sakiroglu CO, Wood C, Cunin-Roy C. Pain in adolescents with cancer. Bull Cancer 2009;96:37-45. [Crossref] [PubMed]
- Reynolds TD, Osborn HL. The use of cannabinoids in chronic pain. BMJ Case Rep 2013;2013:bcr2013010417. [Crossref] [PubMed]
- Montague-Cardoso K, Pitcher T, Chisolm K, et al. Changes in vascular permeability in the spinal cord contribute to chemotherapy-induced neuropathic pain. Brain Behav Immun 2020;83:248-59. [Crossref] [PubMed]
- Forte BL, Slosky LM, Zhang H, et al. Angiotensin-(1-7)/Mas receptor as an antinociceptive agent in cancer-induced bone pain. Pain 2016;157:2709-21. [Crossref] [PubMed]
- Yokoshima Y, Sumitani M, Nishizawa D, et al. Gamma-aminobutyric acid transaminase genetic polymorphism is a candidate locus for responsiveness to opioid analgesics in patients with cancer pain: An exploratory study. Neuropsychopharmacol Rep 2018;38:175-81. [Crossref] [PubMed]
- Ling D, Zhao Y, Zhang Z, et al. Morphine inhibits the promotion of inflammatory microenvironment on chronic tibial cancer pain through the PI3K-Akt-NF-kappaB pathway. Am J Transl Res 2020;12:6868-78.
- Jiang B, Zhong X, Fang J, et al. Electroacupuncture Attenuates Morphine Tolerance in Rats with Bone Cancer Pain by Inhibiting PI3K/Akt/JNK1/2 Signaling Pathway in the Spinal Dorsal Horn. Integr Cancer Ther 2021;20:1534735421995237. [Crossref] [PubMed]
- Wang J, Zhang R, Dong C, et al. Transient Receptor Potential Channel and Interleukin-17A Involvement in LTTL Gel Inhibition of Bone Cancer Pain in a Rat Model. Integr Cancer Ther 2015;14:381-93. [Crossref] [PubMed]
- Jiang X, Zhou R, Zhang Y, et al. Interleukin-17 as a potential therapeutic target for chronic pain. Front Immunol 2022;13:999407. [Crossref] [PubMed]
- Rausch SM, Gonzalez BD, Clark MM, et al. SNPs in PTGS2 and LTA predict pain and quality of life in long term lung cancer survivors. Lung Cancer 2012;77:217-23. [Crossref] [PubMed]
- Mafu TS, September AV, Shamley D. Regulatory VCAN polymorphism is associated with shoulder pain and disability in breast cancer survivors. Hum Genomics 2021;15:36. [Crossref] [PubMed]
- Guo CH, Bai L, Wu HH, et al. The analgesic effect of rolipram is associated with the inhibition of the activation of the spinal astrocytic JNK/CCL2 pathway in bone cancer pain. Int J Mol Med 2016;38:1433-42. [Crossref] [PubMed]
(English Language Editor: D. Fitzgerald)