Construction and validation of a novel prognostic model using the cellular senescence-associated long non-coding RNA in gastric cancer: a biological analysis
Introduction
Globally, gastric cancer (GC) was the 5th most prevalent cancer and 3rd major cause of mortality (1). Advances in oncology, such as radiation therapy, neo- and adjuvant chemotherapies, and more recently, immunotherapies, like the immune checkpoint inhibitors (ICIs), are used for treating advanced GC patients; however, in most cases, the prognosis is still poor (2). Therefore, it is essential to determine the significance of the potential biomarkers of GC to improve the prognosis of GC for guiding clinical treatment.
Cellular senescence refers to cells losing their ability to divide so that they are in a permanent state of cell-cycle arrest. Unlike body senescence, cellular senescence can occur at various stages of development and growth and is important for maintaining tissue homeostasis and preventing the expansion of damaged cells. Cellular senescence is linked to several diseases, like neurodegenerative Alzheimer’s disease (3), chronic kidney disease (4,5), non-alcoholic fatty liver disease (6), type 2 diabetes (7), and prostate disease in older men (8). However, the link between tumor formation and cellular senescence remains controversial. During the early stages of the senescence-associated secretory phenotype (SASP), SASP refers to the fact that senescent cells are in growth arrest, but still have relatively active metabolic activity, synthesizing a large number of proteins, such as interleukins, growth factors, chemokines, and matrix metalloproteinases. And affects the tissue microenvironment in a paracrine manner, which can promote tissue repair, activate the immune system, and initiate immune surveillance of senescent cells (9). However, prolonged SASP can reshape the microenvironment, leading to chronic inflammation and promoting tumor development. When considering the relationship between cellular senescence and tumors, it is important to systematically study the role of cellular senescence-related genes in GC.
The long non-coding RNAs (lncRNAs) are >200 nucleotides long and can control gene expression, RNA splicing, and microRNA (miRNA) regulation, but do not have protein translation functions. In the past few years, several researchers have noted that the cell senescence-linked lncRNAs were involved in the onset and progression of tumors, including cell senescence-linked lncRNA-HOXA-AS3 [HOXA cluster antisense RNA 3 (HOXA-AS3)] through the formation of the HOXA-AS3 duplex. The formation of more stable HOXA6 helps in promoting the invasion, proliferation, and migration of malignant lung adenocarcinoma cells (10). LncRNA-HOTAIR [HOX transcript antisense RNA (HOTAIR)] contributes to cellular senescence and chemoresistance in ovarian and other cancers by reducing Iκ-Bα (an NF-κB inhibitor) and regulating NF-κB activation (11). However, very few researchers have investigated the role played by the cellular senescence-linked lncRNAs in GC. Hence, it is important to identify lncRNAs that are associated with cellular senescence for predicting the prognosis of GC.
Although GC has a considerable number of prognostic markers such as carcinoembryonic antigen (CEA) and cancer antigen 199 (CA199), etc. But the prognosis and survival of GC are not good. Therefore, some new prognostic models have emerged to help clinical judgment and guide clinical treatment [e.g., N6-methyladenosine-related lncRNAs (12), ferroptosis-related lncRNAs (13), and hypoxia-related lncRNAs (14), etc.]. However, there is no research on cellular senescence-related lncRNAs in the stomach adenocarcinoma (STAD) prognostic model. In this research, it was hypothesized that the lncRNAs linked to cellular senescence could be used as promising prognostic biomarkers in the treatment of GC. The relationship between the OS, expression of the cellular senescence-linked lncRNAs, and the clinicopathological factors for STAD patients, was derived using The Cancer Genome Atlas (TCGA) database. Furthermore, a novel prognostic signature integrating 11 lncRNAs linked to cellular senescence was constructed, and its prognostic ability to accurately and independently predict the prognosis of STAD patients was determined. Figure 1 presents a schematic diagram depicting the workflow used in the study. We present the following article in accordance with the TRIPOD reporting checklist (available at https://jgo.amegroups.com/article/view/10.21037/jgo-22-662/rc).
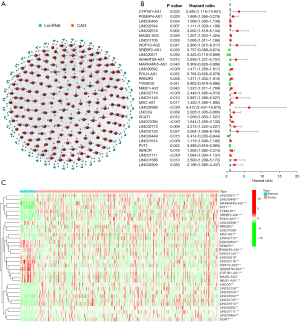
Methods
Obtaining GC data information from public databases
This study was carried out as per the Declaration of Helsinki (as revised in 2013). All RNA-seq data [fragments per kilobase million (FPKM) values] and the STAD-related clinical data were acquired from TCGA database (15). This included all information regarding the sex, age, tumor-node-metastasis (TNM) stage, and overall survival (OS) status of GC patients. For differentiating between the lncRNAs and mRNAs, the GTF files were also retrieved from Ensembl (https://uswest.ensembl.org/index.html) for additional analysis (16). The downloaded data were then normalized, processed, and assessed with the help of the R software 4.1.2. A total of 279 cellular senescence-linked genes were derived from the cellular senescence-associated database, i.e., CellAge (https://genomics.senescence.info/cells/) (17), and all the genes were verified experimentally.
Identification of lncRNAs associated with cellular senescence
Using the public database, i.e., CellAge, a total of 279 cellular senescence-linked genes were downloaded and experimentally verified. The detailed gene list is shown in Table S1. The expression data for the 279 cellular senescence-linked genes was further downloaded from the TCGA database. LncRNAs related to cellular senescence genes were detected by Pearson’s correlation analysis, and lncRNAs showing a P<0.001 and correlation coefficient |R2|>0.4 were screened out. These RNAs are considered to be highly correlated with cellular senescence in GC. Next, a univariate Cox regression analysis was carried out with the above-mentioned cell senescence-linked lncRNAs to screen the lncRNAs linked to the OS of the GC patients. Herein, P<0.05 was considered a screening condition.
Constructing and validating the aging-linked lncRNA risk signature
Survival data and expression data were combined, and 371 GC samples were left after excluding samples without any OS status or time. The above 371 GC patients were classified randomly into a test group and a training group in a 1:1 ratio. The best candidates were then examined using least absolute shrinkage and selection operator (LASSO) Cox regression analysis, and prognostic signatures were developed using various cellular senescence lncRNA signatures. The median risk score was employed for separating the low- and high-risk groups (categories). Using the log-rank test and Kaplan-Meier (KM) analysis, differences in the OS across various risk categories in the testing and training datasets were evaluated. The prediction capability of the survival signal was evaluated based on the area under the curve (AUC) values and the time-dependent receiver operating characteristic (ROC) curves. The R packages like caret, pheatmap, survival, and ggpupr were used in the aforementioned procedures.
Correlation between the 11 cellular senescence-linked lncRNA signature models and clinicopathological factors
The Cox regression analysis was implemented for assessing if the prognostic model comprising 11 cellular senescence-linked lncRNAs acted as an independent risk factor for the prognosis of the GC patients. The relationships between the clinical characteristics, molecular subtypes, and prognosis were determined to study the clinical value of molecular subtypes in GC. Age, sex, tumor site, and TNM stage were among the patient’s characteristics. The KM technique was employed for identifying the differences in OS status across different groups, and the survminer and survival modules of the R software were used to display the results.
Construction and assessment of the nomogram prediction model
Herein, a nomogram was developed using the risk score and the clinicopathological factors for predicting the 1-, 3-, and 5-year OS outcomes (prognoses) of the patients with GC. This nomogram could be used for assessing the probable clinical significance of the new prognostic model that was developed using the 11 cellular senescence-linked lncRNAs. The consistency between the observed and predicted OS rates by the nomogram was then evaluated using the calibration curves.
Gene set enrichment analysis (GSEA)
The GSEA technique was utilized for determining the associated functions and signaling pathways, whereas the javaGSEA tool was used for identifying the high and low scores of GC patients to determine the differences in the signaling pathways between both risk groups (18). Further investigation was carried out for understanding the biological functions and pathways associated with the differentially expressed genes (DEGs) in both the risk categories. DEGs between the high- and low-risk groups were identified using the cutoff values of false discovery rate (FDR) <0.05 and |log fold change (FC)|>1. The Kyoto Encyclopedia of Genes and Genomes (KEGG) and Gene Ontology (GO) enrichment analyses were carried out with the Profiler R package cluster (19).
Assessment of the immune microenvironment, infiltration status of immune cells, and the immune surveillance points
The estimation technique of the “estimates” package was used for estimating the proportions of the tumor microenvironment (TME) yielding the immune, stromal, and estimated scores. The proportion of every corresponding component in the TME was seen to increase as the score increased. The activity of the 13 immune-associated pathways was assessed using the single-sample GSEA (ssGSEA) technique, an enrichment technique frequently employed in medical research. The immune infiltration rates of 22 different immunological cells in the tumor samples were determined using the CIBERSORT method. Furthermore, the variations in the Immune Checkpoint genes in both risk groups were determined based on previously published reports (20,21).
Statistical analysis
R soft 4.1.2 was used to process all the data. The correlation between the co-expression of lncRNAs and genes linked to cellular senescence was investigated using Pearson’s correlation analysis. Prognostic characteristics were found using LASSO regression analysis. Additionally, the univariate and multivariate Cox analyses were used for evaluating if the risk score could be employed as an independent prognostic factor for STAD. The risk model’s sensitivity and specificity were assessed using the Area under the ROC curve. The Fisher’s exact or chi-square tests were implemented for probing into the differences across all categorical variables. Furthermore, the Student’s t-test was used for comparing the normally-distributed continuous variables between both the risk groups.
Results
Identification of lncRNAs associated with cellular senescence
Figure S1 displays the entire process used in this study in detail. TCGA database was utilized to gather the gene expression data of 279 genes linked to cellular senescence in the GC patients, which included 32 normal as well as 375 tumor samples. Pearson’s correlation analysis was implemented for identifying the lncRNAs linked to the genes that were related to cellular senescence, and lncRNAs with correlation coefficients |R2|>0.4 and P<0.001 were filtered out (Figure 1A). P<0.05 was the screening threshold, and 33 lncRNAs associated with the OS status of the patients with GC were examined, as presented in Figure 1B. Figure 1C illustrates the gene expression patterns of lncRNAs in the normal and malignant tissue tumor samples. Subsequently, the expression level of these lncRNAs was examined.
Constructing and validating the prognostic model of cellular senescence-related lncRNAs
Excluding samples without OS status or time, the remaining 371 GC patients were randomly categorized into the test group (n=184) and the training group (n=187) in a 1:1 ratio. LASSO Cox regression analysis was performed based on 33 cellular senescence-associated lncRNAs in the training cohort. It was found that 11 characteristic lncRNAs related to cellular senescence could be used for model construction (Figure 2A,2B). The risk scores for every patient were estimated using the data for the 11 relevant lncRNAs along with their correlation coefficients based on the formula below:
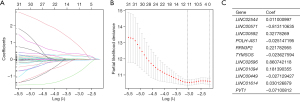
Risk score = LINC02544 × 0.011000997 + LINC00571 × −0.813110635 + LINC00592 × 0.32778269 + POLMSH-AS1 × −0.025147195 + RRN3P2 × 0.221782955 + TYOS × −0.023627594 + LINC02696 × 0.860742118 + LINC01094 × 0.181390355 + LINC00449 × −0.027129427 + LINC01614 × 0.030126879 + PVT1 × −0.07100812.
The related lncRNAs and their correlation coefficients are shown in Figure 2C (the names of these RNAs represent their expression levels). The GC patients included in the training and test groups were further categorized into the low-risk and high-risk categories depending on their median risk scores. Figure 3A,3B present the risk score, OS status, and gene expression in the GC patients, while Figure 3C shows a heatmap of the 11 characteristic genes that were used for constructing the prognostic model.
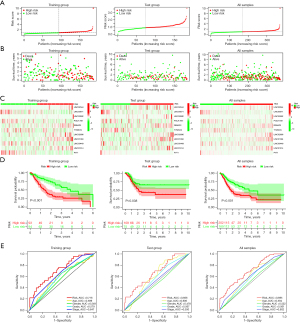
KM survival curve analysis was employed for assessing the model’s prognostic validity in GC patients, and the findings demonstrate that the high-risk category patients showed considerably worse OS rates (P<0.001) (Figure 3D). Analysis of the ROC curves helped in assessing the model’s prediction accuracy. The results revealed that the AUC value in the training group was 0.716 (Figure 3E). Furthermore, the efficacy and accuracy of the predictions based on the 11 lncRNAs linked to cellular senescence were further verified in the test and combination groups (including all the samples). Figure 3D illustrates the results of a KM survival analysis, which showed that the 5-year OS rate in the high-risk GC category was considerably decreased in the verification (P<0.38) and combination groups (P<0.001). The ROC analysis indicated that the AUC values in the test and combination groups were recorded to be 0.666 and 0.695, respectively, as presented in Figure 3E. These results show that cellular senescence-related lncRNAs have strong prognostic predictive value.
Correlation between 11 lncRNA signature models associated with cellular senescence and clinicopathological factors
The Cox regression analysis was conducted to determine if the developed prognostic model that was constructed using the 11 cellular senescence-linked lncRNAs can be utilized as the independent risk factor for the GC prognosis. T (P=0.49), age (P=0.01), stage (P<0.001), N (P<0.001), and risk score (P<0.001) were all significant factors that affected the prediction of the GC prognosis, as per the results displayed by the univariate Cox regression results analysis (Figure 4A). Figure 4B displays the multivariate regression analysis results that indicated that the age [P<0.001; hazard ratio (HR): 1.032; 95% confidence interval (CI): 1.013–1.052] and the risk score (P<0.001; HR: 1.387; 95% CI: 1.247–1.543) were used as the independent prognostic indicators for GC. In addition, Figure 4C illustrates the correlation between the clinicopathological factors and the expression profile of the 11 cellular senescence-linked lncRNAs.
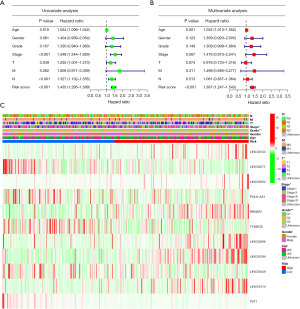
For validating the stability of this prognostic model, the GC samples were classified into different categories as per their varying clinical or pathological features. The KM survival analysis was carried out for every subgroup. Results are presented for all the subgroups in terms of factors like age, sex, T stage, N stage, M stage, clinical stage, and grade (Figure 5A-5N, respectively). The high-risk groups showed a lower OS rate for all the above factors (except the M stage). These results show the accuracy and stability of the predictive model.
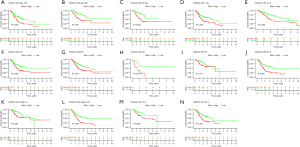
Developing and evaluating a prognostic nomogram depending on the cellular senescence-linked lncRNAs
To assess the potential clinical utility of a prognostic model based on 11 cellular senescence-associated lncRNAs, a nomogram was constructed that determined the likelihood of OS in these patients after adding the scores of many associated factors. The 1-, 3-, and 5-year OS values in the patients with GC were accurately predicted by comparison with ideal prediction models (Figure 6A,6B).
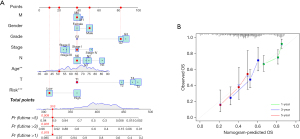
Molecular function (MF) and pathway discovery by GSEA, GO, and KEGG analyses
To investigate the pathway differences between different groups of 11 cellular senescence-related lncRNAs, the GSEA technique was implemented. The results indicated that many pathways associated with tumor progression were enriched significantly in the high-risk category, like JAK-STAT and MAPK signaling pathways. Additionally, the patients in the high-risk category showed significant enrichment in the immune-related pathways, like the B-cell and T-cell receptor-related pathways, NK-cell-mediated cytotoxicity-based pathways, and the gut immune network-linked pathways. Significant enrichment of high-risk groups was found in many tumors and immune-related diseases, such as autoimmune-related diseases, melanoma, basal cell carcinoma, and renal cell carcinoma, as shown in Figure 7. Table S2 presents additional details. The low-risk patients had similar genes that were primarily enriched in the DNA repair-associated pathways and pyrimidine metabolism-related pathways, as shown in Figure 7. Table S3 presents additional details. The functional and pathway enrichment analyses associated with 2 sets of DEGs were conducted. Cutoff thresholds for |logFC|>1 and FDR <0.05 followed by the annotated KEGG and GO enrichment analyses (P<0.05), were utilized to identify the DEGs between both the risk groups. Similar to the GSEA findings, KEGG analysis revealed a high enrichment of numerous immune-related pathways (Figure 7B). A GO analysis was carried out and the findings revealed that MF, biological process (BP), and cellular component (CC) were subjected to enrichment. In Figure 7C, the outcomes of the above 3 analyses were displayed. Taken together, all the findings suggested that the risk scores for 11 cellular senescence-associated lncRNA signatures are primarily involved in tumor development and are associated with tumor immunity.
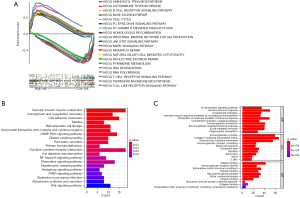
Association of cellular senescence-associated lncRNAs with the infiltration of the immune cells and different immune checkpoints
Using the ESTIMATE algorithm, the immune, stromal, and ESTIMATE scores of the 2 risk groups were predicted. As presented in Figure 8A-8C, some differences were noted in the immune (P<0.001), stromal (P<0.001), and ESTIMATE (P<0.001) scores across the groups. In comparison to high-risk patients, the low-risk patients showed a higher tumor purity. The ssGSEA-based correlation analysis was carried out between different immune cell subsets and the associated functions and the findings revealed substantial differences in the immune functions across the groups. Immune cell functions such as type I interferon (IFN) response, inflammation-promoting, type II IFN response, T cell co-stimulation, antigen presenting cell (APC) co-inhibition, cytolytic activity, checkpoint, T cell co-inhibition, human leukocyte antigen (HLA), para-inflammation, APC co-stimulation, and CC chemokine receptor (CCR), were increased significantly (P<0.001) in the high-risk group (Figure 8D). Correlation analyses among the 22 immune cells revealed differences in 4 cell types, including resting dendritic cells, monocytes, M2 macrophages, and plasma cells. Plasma cells were observed to be prevalent in the patients included in the low-risk category, whereas the high-group patients showed a higher prevalence of the other three cell types (Figure 8E). The results also showed that blocking the immune checkpoint pathways could be a very promising strategy for achieving anticancer immunity. As a result, the variations in the expression of multiple ICGs were assessed and compared between both groups. Nearly all the immune checkpoints were different between both groups. According to the findings (Figure 9), the immune-linked genes were more prevalent in the high-risk category, suggesting that ICIs are more responsive in the patients included in the high-risk category, who were receiving immunotherapy.
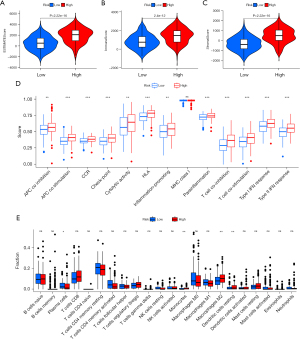
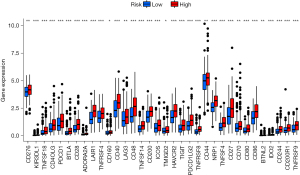
Discussion
Many current reports have focused on determining the role played by the cellular senescence-linked genes in tumors. Recently, lncRNAs related to cellular senescence have attracted more and more attention. For example, Si et al. reported that lncRNA-HEIH promoted ovarian cancer cell proliferation by suppressing cellular senescence through the miR-3619-5p/CTTNBP2 axis (22). Li et al. found that the lncRNA USP2-AS1 acts as a direct transcriptional target of c-Myc to inhibit senescence and promote tumor progression (23). Hence, it is essential to identify potential markers of cellular senescence-related lncRNAs in cancer. However, the current research in GC is still insufficient.
In this research, the expression profiles of 279 cellular senescence-linked genes were analyzed, and they were observed to be updated by the CellAge database and validated experimentally. Depending on the co-expression of the cellular senescence-linked genes, the related lncRNAs were then screened. Next, the expression profiles of the senescence-linked lncRNAs and the prognosis of the patients with GC were downloaded using the TCGA cohort and then examined. In total, 33 prognostic lncRNAs were identified using these results. The best candidates were further analyzed using the LASSO Cox regression analysis for constructing the novel prognostic model integrating 11 cellular senescence-linked lncRNAs. This novel model was verified using the internal cohort. Additionally, a predictive nomogram was constructed after combining the clinical traits with the lncRNA markers related to cellular senescence. The design of the new signature could help in differentiating among the different risk samples. The OS status of the STAD in the training cohort was lower in the high-risk category in comparison to that in the low-risk subtype, and similar findings were observed in the test cohort. Furthermore, the risk scores were found to be the independent predictor of OS in STAD patients, depending on the findings of the univariate and multivariate Cox regression analyses. Altogether, these findings show how the prognostic signatures integrating cellular senescence-linked lncRNAs could successfully predict the OS rate in GC patients.
The TME is crucial for the advancement of malignant tumors, immune evasion, and treatment resistance. TME can be impacted by variations in any gene. Therefore, in this report, the correlation noted between the infiltration status of immune cells in the tumor tissues and the cellular senescence-linked lncRNAs was examined.
Tumor-associated macrophages have been extensively studied, and their numbers are thought to correlate with tumor grade and survival (24,25). Although the function of tumor-associated macrophages is highly plastic, tumor-associated macrophages eventually transition to an immunosuppressive phenotype as the tumor progresses. As characteristic surface molecules of tumor-associated macrophages, CD163 and CD206 have properties related to stimulating angiogenesis, inhibiting adaptive immunity, and promoting tumor growth and metastasis (26-28). The predominant phenotype of tumor-associated macrophages is currently considered to be the M2 type. Some studies have found that lung cancer cells can induce the polarization of macrophages to the M2 subtype, which in turn enhances the invasion, migration, and epithelial-mesenchymal transition (EMT) of lung cancer cells (29,30). Yamaguchi et al. reported that M2 macrophages promote peritoneal dissemination in GC patients and lead to tumor spread and progression (31). The M2 isoform promotes EMT in pancreatic cancer cells through the TLR-4/IL-10 signaling pathway (32). M2 macrophages are considered tumor-promoting “bad” macrophages because they produce growth factors, activate tissue repair and angiogenesis, and have high scavenging activity that suppresses adaptive immune responses (33). These results are consistent with those observed in this study, where the levels of M2 macrophages were seen to be significantly enhanced in the high-risk category compared to the low-risk category. Macrophages are derived from monocytes. The study found that after monocytes reach the tumor after the Notch signaling transcriptional regulator RBPJ is activated, they differentiate into tumor-associated macrophages (34). This finding is also consistent with the findings reported in this study, and the enrichment analysis showed significant enrichment in the Notch pathway. Immune cell analysis also indicated that the GC patients in the high-risk category showed a higher level of monocytes compared to the low-risk GC patients. Therefore, the high-risk category was significantly associated with immunity in the TME and likely contributed to the progression of GC by increasing the infiltration level of M2-type macrophages. Immune checkpoints include immunostimulatory factors and immunosuppressive molecules. In recent years, ICIs represented by the PD-1/PD-L1 antibodies were commonly used in tumors. Moreover, the variations noted in the gene expression of immune checkpoint genes between both the categories were assessed, and the results revealed that the GC patients in the high-risk category showed a higher level of immune-related genes, indicating that ICIs are more sensitive in high-risk GC patients receiving immunotherapy.
This study has a few drawbacks. Firstly, the TCGA database was used as the sole data source in this study, with no additional datasets used to verify our findings. Other datasets should be used in future studies to confirm the performance of the proposed predictive signature. Secondly, the results noted in the study need to be validated, both in vivo and in vitro. The underlying processes of the cellular senescence-linked lncRNAs were not studied. Therefore, to assess the predictive performance of the constructed signature and identify the relevant regulatory mechanisms, functional studies should explore these 11 lncRNAs separately and thoroughly. Despite all these drawbacks, this is, to the best of the researcher’s knowledge, the first report where the cellular senescence-linked lncRNAs have been established and validated in a GC prognostic model.
Conclusions
According to the findings presented in this study, the cellular senescence-linked lncRNA signatures can act as an effective prognostic marker in GC patients. It will direct the development of the GC biomarkers and a more precise immune modulation.
Acknowledgments
Funding: This work was supported by the Application Foundation Project of Changzhou Science and Technology Bureau (No. CJ20210165) and the General Project of Nanjing Medical University (No. NMUB2020073).
Footnote
Reporting Checklist: The authors have completed the TRIPOD reporting checklist. Available at https://jgo.amegroups.com/article/view/10.21037/jgo-22-662/rc
Conflicts of Interest: All authors have completed the ICMJE uniform disclosure form (available at https://jgo.amegroups.com/article/view/10.21037/jgo-22-662/coif). The authors have no conflicts of interest to declare.
Ethical Statement: The authors are accountable for all aspects of the work in ensuring that questions related to the accuracy or integrity of any part of the work are appropriately investigated and resolved. The study was conducted in accordance with the Declaration of Helsinki (as revised in 2013).
Open Access Statement: This is an Open Access article distributed in accordance with the Creative Commons Attribution-NonCommercial-NoDerivs 4.0 International License (CC BY-NC-ND 4.0), which permits the non-commercial replication and distribution of the article with the strict proviso that no changes or edits are made and the original work is properly cited (including links to both the formal publication through the relevant DOI and the license). See: https://creativecommons.org/licenses/by-nc-nd/4.0/.
References
- Li Y, Yan J, Wang Y, et al. LINC00240 promotes gastric cancer cell proliferation, migration and EMT via the miR-124-3p / DNMT3B axis. Cell Biochem Funct 2020;38:1079-88. [Crossref] [PubMed]
- Kwak Y, Seo AN, Lee HE, et al. Tumor immune response and immunotherapy in gastric cancer. J Pathol Transl Med 2020;54:20-33. [Crossref] [PubMed]
- Kritsilis M, V, Rizou S, Koutsoudaki PN, et al. Ageing, Cellular Senescence and Neurodegenerative Disease. Int J Mol Sci 2018;19:2937. [Crossref] [PubMed]
- Wang Y, Wang Y, Yang M, et al. Implication of cellular senescence in the progression of chronic kidney disease and the treatment potencies. Biomed Pharmacother 2021;135:111191. [Crossref] [PubMed]
- Tan H, Xu J, Liu Y. Ageing, cellular senescence and chronic kidney disease: experimental evidence. Curr Opin Nephrol Hypertens 2022;31:235-43. [Crossref] [PubMed]
- Engelmann C, Tacke F. The Potential Role of Cellular Senescence in Non-Alcoholic Fatty Liver Disease. Int J Mol Sci 2022;23:652. [Crossref] [PubMed]
- Narasimhan A, Flores RR, Robbins PD, et al. Role of Cellular Senescence in Type II Diabetes. Endocrinology 2021;162:bqab136. [Crossref] [PubMed]
- Fiard G, Stavrinides V, Chambers ES, et al. Cellular senescence as a possible link between prostate diseases of the ageing male. Nat Rev Urol 2021;18:597-610. [Crossref] [PubMed]
- Zhang JW, Zhang D, Yu BP. Senescent cells in cancer therapy: why and how to remove them. Cancer Lett 2021;520:68-79. [Crossref] [PubMed]
- Zhang H, Liu Y, Yan L, et al. Increased levels of the long noncoding RNA, HOXA-AS3, promote proliferation of A549 cells. Cell Death Dis 2018;9:707. [Crossref] [PubMed]
- Özeş AR, Miller DF, Özeş ON, et al. NF-κB-HOTAIR axis links DNA damage response, chemoresistance and cellular senescence in ovarian cancer. Oncogene 2016;35:5350-61. [Crossref] [PubMed]
- Xu S, Chen W, Wang Y, et al. N6-methyladenosine-related lncRNAs identified as potential biomarkers for predicting the overall survival of Asian gastric cancer patients. BMC Cancer 2022;22:721. [Crossref] [PubMed]
- Li J, Xiang R, Song W, et al. A Novel Ferroptosis-Related LncRNA Pair Prognostic Signature Predicts Immune Landscapes and Treatment Responses for Gastric Cancer Patients. Front Genet 2022;13:899419. [Crossref] [PubMed]
- Fan Z, Wang Y, Niu R. Identification of the three subtypes and the prognostic characteristics of stomach adenocarcinoma: analysis of the hypoxia-related long non-coding RNAs. Funct Integr Genomics 2022; Epub ahead of print. [Crossref] [PubMed]
- Hutter C, Zenklusen JC. The Cancer Genome Atlas: Creating Lasting Value beyond Its Data. Cell 2018;173:283-5. [Crossref] [PubMed]
- Howe KL, Achuthan P, Allen J, et al. Ensembl 2021. Nucleic Acids Res 2021;49:D884-91. [Crossref] [PubMed]
- Basisty N, Kale A, Jeon OH, et al. A proteomic atlas of senescence-associated secretomes for aging biomarker development. PLoS Biol 2020;18:e3000599. [Crossref] [PubMed]
- Subramanian A, Tamayo P, Mootha VK, et al. Gene set enrichment analysis: a knowledge-based approach for interpreting genome-wide expression profiles. Proc Natl Acad Sci U S A 2005;102:15545-50. [Crossref] [PubMed]
- Yu G, Wang LG, Han Y, et al. clusterProfiler: an R package for comparing biological themes among gene clusters. OMICS 2012;16:284-7. [Crossref] [PubMed]
- Newman AM, Liu CL, Green MR, et al. Robust enumeration of cell subsets from tissue expression profiles. Nat Methods 2015;12:453-7. [Crossref] [PubMed]
- Zhang Y, Zheng J. Functions of Immune Checkpoint Molecules Beyond Immune Evasion. Adv Exp Med Biol 2020;1248:201-26. [Crossref] [PubMed]
- Si L, Chen J, Yang S, et al. lncRNA HEIH accelerates cell proliferation and inhibits cell senescence by targeting miR-3619-5p/CTTNBP2 axis in ovarian cancer. Menopause 2020;27:1302-14. [Crossref] [PubMed]
- Li B, Zhang G, Wang Z, et al. c-Myc-activated USP2-AS1 suppresses senescence and promotes tumor progression via stabilization of E2F1 mRNA. Cell Death Dis 2021;12:1006. [Crossref] [PubMed]
- Tiainen S, Tumelius R, Rilla K, et al. High numbers of macrophages, especially M2-like (CD163-positive), correlate with hyaluronan accumulation and poor outcome in breast cancer. Histopathology 2015;66:873-83. [Crossref] [PubMed]
- Gartrell RD, Marks DK, Hart TD, et al. Quantitative Analysis of Immune Infiltrates in Primary Melanoma. Cancer Immunol Res 2018;6:481-93. [Crossref] [PubMed]
- Ino Y, Yamazaki-Itoh R, Shimada K, et al. Immune cell infiltration as an indicator of the immune microenvironment of pancreatic cancer. Br J Cancer 2013;108:914-23. [Crossref] [PubMed]
- Di Caro G, Cortese N, Castino GF, et al. Dual prognostic significance of tumour-associated macrophages in human pancreatic adenocarcinoma treated or untreated with chemotherapy. Gut 2016;65:1710-20. [Crossref] [PubMed]
- Noy R, Pollard JW. Tumor-associated macrophages: from mechanisms to therapy. Immunity 2014;41:49-61. [Crossref] [PubMed]
- Guo Z, Song J, Hao J, et al. M2 macrophages promote NSCLC metastasis by upregulating CRYAB. Cell Death Dis 2019;10:377. [Crossref] [PubMed]
- Yu X, Xu M, Li N, et al. β-elemene inhibits tumor-promoting effect of M2 macrophages in lung cancer. Biochem Biophys Res Commun 2017;490:514-20. [Crossref] [PubMed]
- Yamaguchi T, Fushida S, Yamamoto Y, et al. Tumor-associated macrophages of the M2 phenotype contribute to progression in gastric cancer with peritoneal dissemination. Gastric Cancer 2016;19:1052-65. [Crossref] [PubMed]
- Liu CY, Xu JY, Shi XY, et al. M2-polarized tumor-associated macrophages promoted epithelial-mesenchymal transition in pancreatic cancer cells, partially through TLR4/IL-10 signaling pathway. Lab Invest 2013;93:844-54. [Crossref] [PubMed]
- Tariq M, Zhang J, Liang G, et al. Macrophage Polarization: Anti-Cancer Strategies to Target Tumor-Associated Macrophage in Breast Cancer. J Cell Biochem 2017;118:2484-501. [Crossref] [PubMed]
- Franklin RA, Liao W, Sarkar A, et al. The cellular and molecular origin of tumor-associated macrophages. Science 2014;344:921-5. [Crossref] [PubMed]
(English Language Editor: R. Scott)