Identification of the key genes controlling stomach adenocarcinoma stem cell characteristics via an analysis of stemness indices
Introduction
Stomach adenocarcinoma (STAD) is the fourth most common malignant tumor in the world, and the third leading cause of death in patients with malignant tumors (1,2). In China, approximately 500,000 newly diagnosed cases are reported every year; thus, STAD represents a major clinical burden in China (3). Currently, multidisciplinary combination therapy has made great progress in improving the prognosis of patients with advanced STAD (4), but the prognosis of patients with STAD is still poor, and the 5-year survival rate is still <40% (5,6). These outcomes are mainly related to the characteristics of STAD, including its high recurrence, metastasis, and strong drug resistance (7,8). The sensitivity and specificity of traditional serological tumor markers are not sufficiently high to diagnose many tumors. Thus, it is of great significance to identify the key factors associated with the adverse prognosis of STAD and to identify reasonable and effective monitoring indicators to enable early intervention and prolong the overall survival rate of STAD patients.
Currently, the theory of cancer stem cells (CSCs) suggests that these cells have the characteristics of self-renewal and uncontrolled proliferation, and may cause metastasis, recurrence, and drug resistance in many tumors (9,10). Only a small number of CSCs are required to cause tumor recurrence and metastasis (11). Multiple studies have shown that CSCs are present in many solid tumors, such as lung cancer, colon cancer, and breast cancer (12-14). The existence of STAD stem cells was confirmed in 2009 when they were cultured in vitro to form distinct spherical colonies in serum-free medium and could be continuously subcultured (15). STAD stem cells have an obvious resistance to a variety of antitumor drugs and radiation. At present, more and more gastric cancer stem cell markers have been found, such as CD44, Lgr5, HER2 and so on. Compared with Lgr5− cells, Lgr5+ cells in patients with gastric cancer are more vulnerable to DNA damage (16). HER2 protein was overexpressed in a high proportion of gastric cancer cases and affected the maintenance of tumor stem cell subsets (17). A study has pointed out that STAD stem cells may promote the proliferation and differentiation of gastric cancer cells through Noth and mTORC signaling pathways (18). Despite the increasing number of studies evaluating STAD stem cells, their role in the pathogenesis and progression of STAD remains unclear.
Stemness indices were indicators to describe the similarity between tumor cells and stem cells. A study of tumor characteristics derived stemness indices using an one-class logistic regression (OCLR) algorithm trained on stem cell (ESC, embryonic stem cell; IPSC, induced pluripotent stem cell) classes and their differentiated ecto-, meso-, and endoderm progenitors (19). OCLR-based transcriptomic and epigenetic signatures were applied to The Cancer Genome Atlas (TCGA) datasets to calculate the mRNA gene expression-based stemness index (mRNAsi) and DNA methylation-based stemness index (mDNAsi) (20). The mRNAsi was an index calculated based on expression data, while the mDNAsi was an index calculated based on DNA methylation data. The two indices range from 0 to 1. The closer they were to 1, the less differentiated the cells were and the stronger the characteristics of stem cells were.
In this study, we sought to construct a weighted gene co-expression network analysis (WGCNA)-based model to identify key CSC-related genes in STAD by combining RNA sequencing expression data from TCGA with a mRNAsi score (21,22). At the same time, we not only discussed the correlation between these key genes and the prognosis of STAD, but also analyzed the expression of these genes in different stages of STAD and the infiltration of immune cells. In addition, the Expression Omnibus (GEO) database was used for further verification. In summary, we used a new method to identify genes associated with STAD CSCs and predict their role in cancer to provide new information on the diagnosis, treatment, and prognostic assessment of STAD.
Methods
Ethical statement
The study was conducted in accordance with the Declaration of Helsinki (as revised in 2013).
Data collection and pre-processing
In March 2020, the RNA sequencing (RNA-seq) expression data of 407 samples (including 32 normal samples and 375 STAD samples) and the corresponding clinical information (age, sex, survival, and tumor, node, metastasis stage) of 407 cases were downloaded from TCGA database (https://portal.gdc.cancer.gov). The mRNAsi was an index calculated based on expression data. A definite tumor sample had constant mRNAsi. This study used the samples from TCGA database, and the values of mRNAsi indices of the STAD patients were constant, which was obtained from a previous study (20). The RNA-seq results from the 32 normal samples and 375 cancer samples were merged into a Perl matrix file, and the gene identities were converted into gene symbols in the matrix profile. After defining the useful information, we extracted the clinical information about the corresponding STAD patients for further analysis.
Clinical characteristics correlation analysis
We used the survival package in R to investigate the prognostic value of mRNAsi. The beeswarm package in R was used to analyze the correlation between clinical characteristics and mRNAsi.
Screening of DEGs
The raw expression data obtained from TCGA was log2 transformed and the limma package in R language was used to identify the differentially expressed genes (DEGs). The cut-off standards for DEG selection were as follows: |log2-fold change| >1, P<0.01, and a false discovery rate (FDR) <0.05. The limma and pheatmap packages in R were used to draw heatmaps and volcano plots.
WGCNA and module preservation
First, the RNA-seq data of the STAD patients was filtered to reduce outliers. The correlation coefficient of genes was then used to construct a Pearson’s correlation matrix, which was then transformed into a weighted adjacency matrix using the following formula: Amn = |Cmn|*β, where Cmn = the Pearson correlation coefficient between gene m and gene n, and Amn = adjacency between gene m and gene n (23). Finally, we selected appropriate β values to ensure scale-free network operations. The weighted adjacency matrix was then transformed into a topological overlap matrix (TOM). Based on the TOM, with a minimum size of 30 as the standard for the gene dendrogram, similar genes were divided into modules and the hierarchical clustering of average links was established.
Identifying key modules and genes
In order to identify the genes that maintain the characteristics of STAD stem cells, we used the WGCNA method. First, we assigned genes with similar expression patterns to a module. To determine the significance of each module, we first calculated the gene significance (GS); that is, the correlation between the sample traits and gene expression levels. The module eigengenes (ME) was considered a key element in the analysis of the principal components of each gene module, and the expression patterns of all the genes was summarized as a single feature expression profile within a given module. Next, for the linear regression between clinical data and gene expression, GS was defined as the log10 conversion of P values (GS=lgP). The module significance (MS) was the average GS of a particular module and represents the correlation between the sample traits and module. The correlation P values were used to determine statistical significance. To increase the capacity of the modules, we selected a cut-off point (<0.25) to merge highly similar modules. After identifying the involved modules, we calculated the GS and module membership (MM) of each gene, and the correlation between the gene expression profile between genes in a module. The threshold values of the key genes in the screening module were defined as MM >0.8 and GS >0.5.
Functional annotation and pathway enrichment analysis
The clusterProfiler package in R was used to perform Kyoto Encyclopedia of Genes and Genomes (KEGG) and Gene Ontology (GO) functional annotation and analysis to study and visualize the biological functions behind the key genes. A P value <0.05 and a FDR <0.05 were considered statistically significant.
Co-expression analysis of the key genes and analysis of the protein-protein interaction network
To determine the co-expression relationship between the key genes, we calculated the Pearson correlation coefficient at the transcriptional level using the corrplot package in R. We chose the Search Tool for the Retrieval of Interacting Genes (STRING) and Cytoscape to evaluate the protein-protein interaction (PPI) network between key genes (24).
Data validation
A Gene Expression Profiling Interactive Analysis (GEPIA; http://GEPIA.cancer-pku.cn/) was conducted to detect the expression of the key genes in different stages of STAD (25). Additionally, using the TIMER (https://cistrome.shinyapps.io/timer/) data, we evaluated the expression of the key genes associated with the immune infiltrates (26). The Kaplan-Meier plotter online tool (http://www.kmplot.com/) was used to examine the correlation between STAD survival and key genes. Finally, we selected 2 data sets [i.e., GSE118916 and GSE112369) from the Gene Expression Omnibus (GEO) database (www.ncbi.nlm.nih.gov/GEO/], and using the online tool of GEO2R with a |log fold change| >1 and an adjusted P value <0.05 as filter conditions, we identified the DEGs, and Venny 2.1.0 online tools (https://bioinfogp.cnb.csic.es/tools/venny/index.html) were then used to define the key genes and common DEGs.
Statistical analysis
The R 3.6.0 software was used to analyze all statistical data. T-test was used for continuous variables. All statistical tests were two-sided, with P<0.05 as the significance level. Spearman’s rank correlation coefficients were used to analyze the correlation between two variables.
Results
Correlation between mRNAsi and clinical characteristics
The mRNAsi of the STAD tissue was significantly higher than that of the normal tissue (P=3.761e-09; see Figure 1A). In the survival analysis, patients with a high mRNAsi score had a lower survival rate than those with a low mRNAsi score (P<0.001; see Figure 1B). The mRNAsi score of the elderly group was significantly higher than that of the non-elderly group (P=0.030; see Figure 1C). There were obvious differences in mRNAsi expression at different stages of STAD, and the mRNAsi score showed a gradually decreasing trend with the worsening of tumor grade (P=0.001; see Figure 1D), tumor stage (P=0.034; see Figure 1E), and T-stage (P=0.024; see Figure 1F).
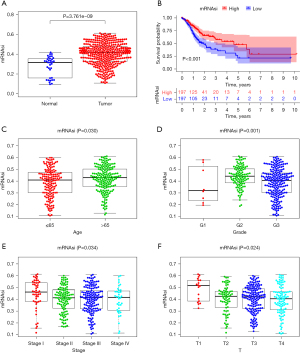
Screening of DEGs
The mRNAsi of the normal tissue differed significantly to that of the STAD tissue, and a total of 6,739 differentially expressed genes (DEGs) were identified, of which 1,146 were downregulated and 5,593 were upregulated (see Figure 2A).
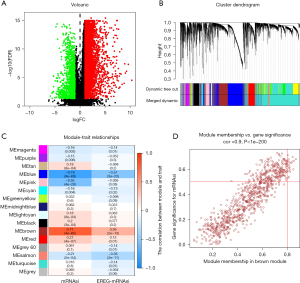
Identification of mRNAsi-related modules and key genes in the WGCNA model
By constructing a co-expression network of the DEGs via a WGCNA, and using the average linkage hierarchical clustering method, all the DEGs were classified into biological gene modules (see Figure 2B). We then identified the gene Bulga stemness closely related to the DEG gene. In this survey, we chose β=4 (scale-free R2 =0.950) as the soft threshold to ensure a scale-free network (see Figure S1) and obtained 11 modules for subsequent analysis. To analyze the relationship between the module and sample traits, we chose MS as the overall gene expression level of the corresponding module and used the clinical phenotype to calculate its correlation. The most significant correlation between the mRNAsi and the brown module was 0.77. Further, there was a relatively high negative correlation between the blue module and the mRNAsi, with a correlation coefficient of –0.78 (see Figure 2C). Thus, we chose the brown module as the most interesting module and used it for the subsequent analysis.
With GS >0.5 and MM >0.8 as the selection criteria, the key genes from the mRNAsi group were identified in the selected brown module. Ultimately, we identified the following 19 key genes: BUB1, BUB1B, KIF14, NCAPH, RACGAP1, KIF15, CENPF, TPX2, RAD54L, KIF18B, KIF4A, TTK, SGO2, PLK4, ARHGAP11A, XRCC2, C1orf112, NCAPG, and ORC6 (see Figure 2D). Additionally, we extracted the specific expression value of each key gene and constructed a heat map (see Figure 3A); the results indicated that all the key genes were significantly overexpressed in STAD (see Figure 3B).
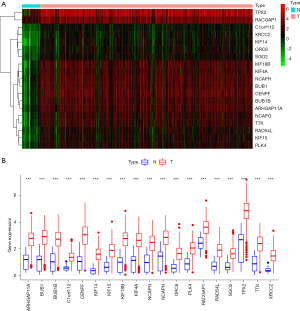
Functional annotation and pathway analysis of genes
The results of the GO and KEGG analyses showed that the main biological processes of the key genes in the brown module involved sister chromatid segregation, mitotic sister chromatid segregation, and nuclear segregation, and their biological functions were mainly related to microtubule motor activity, microtubule binding, and motor activity (see Figure 4). In the signal pathway enrichment analysis, the key genes were mainly involved in the cell cycle (see Figure 4B).
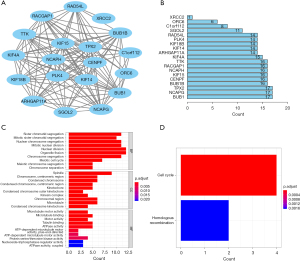
Correlation between key transcription genes and protein levels
The Pearson’s correlation analysis revealed a strong and statistically significant correlation between the key genes (P<0.01). Among these, the highest correlation coefficient between CENPF and KIF4 was 0.88, while the lowest correlation coefficient between ORC6 and KIF4 was 0.52 (see Figure 5). The STRING analysis revealed a strong interaction between the key genes (see Figure 4C). The BUB1, NCAPG, and TPX2 genes had the most edges in the PPI network (see Figure 4D).
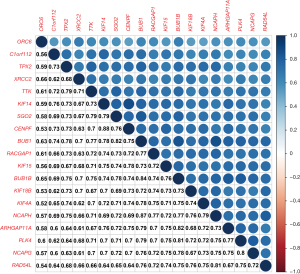
Data verification
To verify the prognostic value of the key genes, we constructed an overall survival curve. There were 2 genes with no data available in the Kaplan-Meier plotter. Among the remaining 17 key genes, 16 were significantly associated with STAD survival (see Figure S2). Among them, the high expression of BUB1, BUB1B, KIF14, NCAPH, RACGAP1, KIF15, CENPF, KIF4A, TTK, PLK4, ARHGAP11A, C1orf112, and NCAPG was associated with a lower overall survival rate for STAD patients. However, the low expression of TPX2, RAD54L, and XRCC2 was associated with a lower overall survival rate for STAD patients. To further examine the key genes, we also conducted a GEPIA to analyze the expression of the key genes in STAD tissues in relation to the pathological stage of the tumor. The violin spectrogram showed that the expression of all the key genes did not differ at different periods (see Figure S3). Additionally, the infiltration of immune cells associated with the expression of the key genes was studied using the TIMER online tool. The results showed that all the key genes were significatively and negatively correlated with the following 6 immune cells: B cells, cluster of differentiation (CD)8+ T cells, CD4+ T cells, macrophages, neutrophils, and dendritic cells (P<0.05; see Figure S4).
Finally, we attempted to validate the key genes using microarray data. We downloaded the GSE112369 and GSE118916 chip datasets from the GEO database, extracted the DEGs using the online tool GEO2R and screened the significantly DEGs using the filter conditions of |log fold change| >1 and an adjusted P value <0.05. The DEGs of GSE112369, the DEGs of GSE118916, and the key genes were plotted using Venn diagrams. TPX2 was identified as common DEG (see Figure 6).
Discussion
STAD is a malignant tumor with high morbidity and mortality, and low 5-year survival rate. The current prevention and treatment strategies for STAD are inadequate. In recent years, CSCs have been reported to be closely related to tumors, including tumor progression, development, drug resistance, and recurrence (27). Thus, it is important and urgent to identify the key genes involved in the transformation of dormant tumor stem cells into cancer cells that are no longer capable of regeneration. In this study, we used WGCNA to identify the key genes that maintain the characteristics of STAD CSCs, and discussed some biological processes involved in these genes and their correlation with the prognosis of STAD. First we explored the correlation between the STAD mRNAsi score and the patient survival rate, and consistent with the results of an mRNAsi study by Malta et al. (20), our results suggested that a higher mRNAsi score is correlated with a lower overall survival rate and progression after initial treatment. The MRNAsi score of the STAD tissue was distinctly higher than that of the normal tissue. The mRNAsi score decreased as tumor stage and grade increased, and stage 1 and grade 1 tumors had the highest stem cell characteristics.
A WGCNA groups genes with similar expression patterns to further analyze the correlation between different gene groups and certain characteristics. This tool is being increasingly used to analyze gene expression patterns across multiple samples (21,22). We constructed a co-expression module using the DEG expression profile of WGCNA in STAD and normal tissues to assess the strength of the correlation between the gene module and the clinical characteristics of interest. In the brown module, which had the highest positive correlation with the mRNAsi, we selected 19 key genes (i.e., BUB1, BUB1B, KIF14, NCAPH, RACGAP1, KIF15, CENPF, TPX2, RAD54L, KIF18B, KIF4A, TTK, SGO2, PLK4, ARHGAP11A, XRCC2, C1orf112, NCAPG, and ORC6) according to the GS and MM scores. The interactions between the proteins of these key genes was obvious. Some of the most closely related proteins were BUB1, NCAPG, and TPX2. Further, these key genes were significantly upregulated in the STAD tissues. Based on the analysis of the key genes correlated with the survival of STAD patients, we identified 13 genes [BUB1, BUB1B (SSK1), KIF14, NCAPH, RACGAP1, KIF15, CENPF, KIF4A, TTK (CT96), PLK4, ARHGAP11A, C1orf112, and NCAPG (CAP-G)] whose lower expression was correlated with higher survival rates, and 3 genes [TPX2 (P100), RAD54L, and XRCC2], whose higher expression was correlated with lower survival rates. Our findings suggested that some of these key genes were protective genes, and some contributed to the pathogenesis of STAD. In the functional analysis of the key genes, we found that these genes were mainly associated with chromosome division, microtubule activity, and activity, which also suggested that they play a role in the regulation of the cell cycle and have properties that exactly match the characteristics of tumor stem cells.
To verify the role of these key genes, we also analyzed their expression in different stages of STAD. Our findings indicated that the expression of the selected key genes did not differ across the different tumor stages of STAD, which suggested that the STAD stem cells expressing these key genes maintained their cell stemness. However, the investigation of the expression of these key genes in infiltrated immune cells revealed that 17 key genes were negatively correlated with the levels of infiltration of B cells, CD8+ T cells, CD4+ T cells, macrophages, neutrophils, and dendritic cells, which may explain why it is so difficult for the body’s immune system to recognize the presence of tumors and why CSCs are insensitive to drug therapy. There was no information available in the TIMER database for 2 of these genes (i.e., ORC6 and SGO2).
Finally, we also verified the expression of the key genes of the brown module in the GSE112369 and GSE118916 data sets, and the only common gene was TPX2. TPX2 has recently been shown to be closely associated with different tumors, its physiological function is to promote cell mitosis, it is involved in DNA damage repair and apoptosis, and its abnormal expression in many solid tumors has been reported to promote tumor cell proliferation and progression (28-30). TPX2 is highly expressed in clear cell carcinoma of the kidney and has been suggested to be an indicator of prognosis (31). TPX2 expression promotes the growth and invasion levels of glioma cells by activating the protein kinase B signaling pathway (32). TPX2 can also promote the proliferation and invasion of liver cancer cells (33). Consistent with the findings of previous research (34,35), in STAD, TPX2 promotes the proliferation, invasion, and metastasis of STAD cells, and its elevated expression predicts the poor prognosis of patients. However, it should be noted that the findings of this study are based on the bioinformatics analysis of retrospective data and additional samples. The main limitation of this study was the lack of experimental data, and additional biological studies were needed to confirm our findings.
In summary, we identified 19 key genes that play an important role in the maintenance of stem cells and whose expression could predict the prognosis of STAD patients. The cell-cycle pathway was the most important signaling pathway for the key genes associated with STAD stem cells. These findings may provide a new rationale for screening therapeutic targets as STAD stem cell inhibitors.
Acknowledgments
Funding: This work was funded by the High-Level Hospital Construction Research Project of Maoming People’s Hospital (Project No. SY2022002), Medical Science and Technology Research Foundation of Guangdong Province (Project No. B2020194).
Footnote
Conflicts of Interest: All authors have completed the ICMJE uniform disclosure form (available at https://jgo.amegroups.com/article/view/10.21037/jgo-22-244/coif). The authors have no conflicts of interest to declare.
Ethical Statement: The authors are accountable for all aspects of the work in ensuring that questions related to the accuracy or integrity of any part of the work are appropriately investigated and resolved. This study was conducted in accordance with the Declaration of Helsinki (as revised in 2013).
Open Access Statement: This is an Open Access article distributed in accordance with the Creative Commons Attribution-NonCommercial-NoDerivs 4.0 International License (CC BY-NC-ND 4.0), which permits the non-commercial replication and distribution of the article with the strict proviso that no changes or edits are made and the original work is properly cited (including links to both the formal publication through the relevant DOI and the license). See: https://creativecommons.org/licenses/by-nc-nd/4.0/.
References
- Bray F, Ferlay J, Soerjomataram I, et al. Global cancer statistics 2018: GLOBOCAN estimates of incidence and mortality worldwide for 36 cancers in 185 countries. CA Cancer J Clin 2018;68:394-424. [Crossref] [PubMed]
- Ang TL, Fock KM. Clinical epidemiology of gastric cancer. Singapore Med J 2014;55:621-8. [Crossref] [PubMed]
- Torre LA, Bray F, Siegel RL, et al. Global cancer statistics, 2012. CA Cancer J Clin 2015;65:87-108. [Crossref] [PubMed]
- Takahashi T, Saikawa Y, Kitagawa Y. Gastric cancer: current status of diagnosis and treatment. Cancers (Basel) 2013;5:48-63. [Crossref] [PubMed]
- Lazăr DC, Tăban S, Cornianu M, et al. New advances in targeted gastric cancer treatment. World J Gastroenterol 2016;22:6776-99. [Crossref] [PubMed]
- Mihmanli M, Ilhan E, Idiz UO, et al. Recent developments and innovations in gastric cancer. World J Gastroenterol 2016;22:4307-20. [Crossref] [PubMed]
- Li K, Dan Z, Nie YQ. Gastric cancer stem cells in gastric carcinogenesis, progression, prevention and treatment. World J Gastroenterol 2014;20:5420-6. [Crossref] [PubMed]
- Wu X, Tao P, Zhou Q, et al. IL-6 secreted by cancer-associated fibroblasts promotes epithelial-mesenchymal transition and metastasis of gastric cancer via JAK2/STAT3 signaling pathway. Oncotarget 2017;8:20741-50. [Crossref] [PubMed]
- Horikoshi Y, Yan Y, Terashvili M, et al. Fatty Acid-Treated Induced Pluripotent Stem Cell-Derived Human Cardiomyocytes Exhibit Adult Cardiomyocyte-Like Energy Metabolism Phenotypes. Cells 2019;8:1095. [Crossref] [PubMed]
- Paolillo M, Colombo R, Serra M, et al. Stem-Like Cancer Cells in a Dynamic 3D Culture System: A Model to Study Metastatic Cell Adhesion and Anti-Cancer Drugs. Cells 2019;8:1434. [Crossref] [PubMed]
- Pan Y, Ma S, Cao K, et al. Therapeutic approaches targeting cancer stem cells. J Cancer Res Ther 2018;14:1469-75. [Crossref] [PubMed]
- Maiuthed A, Chantarawong W, Chanvorachote P. Lung Cancer Stem Cells and Cancer Stem Cell-targeting Natural Compounds. Anticancer Res 2018;38:3797-809. [Crossref] [PubMed]
- Gupta R, Bhatt LK, Johnston TP, et al. Colon cancer stem cells: Potential target for the treatment of colorectal cancer. Cancer Biol Ther 2019;20:1068-82. [Crossref] [PubMed]
- Quaglino E, Conti L, Cavallo F. Breast cancer stem cell antigens as targets for immunotherapy. Semin Immunol 2020;47:101386. [Crossref] [PubMed]
- Wu Y, Li Z, Zhang C, et al. CD44 family proteins in gastric cancer:a meta-analysis and narrative review. Int J Clin Exp Med 2015;8:3595-606. [PubMed]
- Uehara T, Ma D, Yao Y, et al. H. pylori Infection Is Associated with DNA Damage of Lgr5- Positive Epithelial Stem Cells in the Stomach of Patients with Gastric Cancer. Dig Dis Sci 2013;58:140-9. [Crossref] [PubMed]
- Song Y, Tong C, Wang Y, et al. Effective and persistent antitumor activity of HER2-directed CAR-T cells against gastric cancer cells in vitro and xenotransplanted tumors in vivo. Protein Cell 2018;9:867-78. [Crossref] [PubMed]
- Hibdon ES, Razumilava N, Keeley TM, et al. Notch and mTOR signaling pathways promote human gastric cancer cell proliferation. Neoplasia 2019;21:702-12. [Crossref] [PubMed]
- Sokolov A, Paull EO, Stuart JM. ONE-CLASS DETECTION OF CELL STATES IN TUMOR SUBTYPES. Pac Symp Biocomput 2016;21:405-16. [Crossref] [PubMed]
- Malta TM, Sokolov A, Gentles AJ, et al. Machine Learning Identifies Stemness Features Associated with Oncogenic Dedifferentiation. Cell 2018;173:338-354.e15. [Crossref] [PubMed]
- Pei G, Chen L, Zhang W. WGCNA Application to Proteomic and Metabolomic Data Analysis. Methods Enzymol 2017;585:135-58. [Crossref] [PubMed]
- Tomczak K, Czerwińska P, Wiznerowicz M. The Cancer Genome Atlas (TCGA): an immeasurable source of knowledge. Contemp Oncol (Pozn) 2015;19:A68-77. [Crossref] [PubMed]
- Chen Y, Bi F, An Y, et al. Coexpression network analysis identified Krüppel-like factor 6 (KLF6) association with chemosensitivity in ovarian cancer. J Cell Biochem 2018; [Epub ahead of print]. [PubMed]
- Szklarczyk D, Gable AL, Lyon D, et al. STRING v11: protein-protein association networks with increased coverage, supporting functional discovery in genome-wide experimental datasets. Nucleic Acids Res 2019;47:D607-13. [Crossref] [PubMed]
- Tang Z, Li C, Kang B, et al. GEPIA: a web server for cancer and normal gene expression profiling and interactive analyses. Nucleic Acids Res 2017;45:W98-W102. [Crossref] [PubMed]
- Li T, Fan J, Wang B, et al. TIMER: A Web Server for Comprehensive Analysis of Tumor-Infiltrating Immune Cells. Cancer Res 2017;77:e108-10. [Crossref] [PubMed]
- Aghaalikhani N, Rashtchizadeh N, Shadpour P, et al. Cancer stem cells as a therapeutic target in bladder cancer. J Cell Physiol 2019;234:3197-206. [Crossref] [PubMed]
- Yang W, Wan H, Shan R, et al. The clinical significance and prognostic value of Xenopus kinesin-like protein 2 expressions in human tumors: A systematic review and meta-analysis. J Cell Physiol 2019; [Epub ahead of print]. [Crossref] [PubMed]
- Wang S, Chen Y, Chai Y. Prognostic role of targeting protein for Xklp2 in solid tumors: A PRISMA-compliant systematic review and meta-analysis. Medicine (Baltimore) 2018;97:e13018. [Crossref] [PubMed]
- Neumayer G, Helfricht A, Shim SY, et al. Targeting protein for xenopus kinesin-like protein 2 (TPX2) regulates γ-histone 2AX (γ-H2AX) levels upon ionizing radiation. J Biol Chem 2012;287:42206-22. [Crossref] [PubMed]
- Glaser ZA, Love HD, Guo S, et al. TPX2 as a prognostic indicator and potential therapeutic target in clear cell renal cell carcinoma. Urol Oncol 2017;35:286-93. [Crossref] [PubMed]
- Gu JJ, Zhang JH, Chen HJ, et al. TPX2 promotes glioma cell proliferation and invasion via activation of the AKT signaling pathway. Oncol Lett 2016;12:5015-22. [Crossref] [PubMed]
- Liang B, Jia C, Huang Y, et al. TPX2 Level Correlates with Hepatocellular Carcinoma Cell Proliferation, Apoptosis, and EMT. Dig Dis Sci 2015;60:2360-72. [Crossref] [PubMed]
- Liang B, Zheng W, Fang L, et al. Overexpressed targeting protein for Xklp2 (TPX2) serves as a promising prognostic marker and therapeutic target for gastric cancer. Cancer Biol Ther 2016;17:824-32. [Crossref] [PubMed]
- Wang G, Wang Q, Li Z, et al. Clinical value of Xenopus kinesin-like protein 2 as a prognostic marker in patients with digestive system cancers: a systematic review and meta-analysis. Onco Targets Ther 2018;11:1229-43. [Crossref] [PubMed]
(English Language Editor: L. Huleatt)