Comprehensive bioinformatics analysis of functional molecules in colorectal cancer
Introduction
With more than 1.9 million cases and 850,000 deaths annually, colorectal cancer (CRC) is the 3rd most common cancer and the 2nd leading cause of cancer-related death worldwide (1,2). Advances in early diagnosis and intervention have been effective in improving the overall survival (OS) rates of CRC patients, but the incidence of CRC remains high. Surgery, radiotherapy, chemotherapy, as well as targeted immunotherapy, which has been introduced in recent years, are treatment options for CRC. However, the treatment of advanced CRC still faces significant challenges as only moderate improvement in patient survival has been accomplished. The 5-year survival rate of patients with metastatic CRC is only 14% (3,4). Thus, further success in combating CRC relies on early diagnosis and innovation in treatment strategies which both need insight from understanding of molecular mechanisms that lead to cancer development.
Recently, gene sequencing technology combined with bioinformatics tools have been widely used in the discovery of tumor markers. Databases, such as The Cancer Genome Atlas (TCGA), Gene Expression Omnibus (GEO), and ONCOMINE, are commonly used to screen differentially expressed genes (DEGs) associated with CRC (5-8). These tools extend understandings of the mechanisms underlying the development of CRC and are used to find novel targets for diagnosis and prognosis. Thus, a bioinformatics analysis is a valuable method for screening DEGs from gene expression data to identify the hub genes associated with CRC.
In the present research, the GSE151021, GSE156720, and GSE156719 data sets were downloaded from the GEO database. The differentially expressed messenger RNAs (DEmRNAs), microRNAs (DEmiRNAs), and long non-coding RNAs (DElncRNAs), associated with CRC were identified through the online tool GEO2R. Subsequently, Gene Ontology (GO) and Kyoto Encyclopedia of Genes and Genomes (KEGG) were used to analyze the biological significance of the identified molecules. The expressions of the hub genes were then verified by Gene Expression Profiling Interactive Analysis 2 (GEPIA2) and UALCAN. Kaplan-Meier plotter (KM plotter) was used in the survival analyses of the hub genes. We further constructed mRNA-miRNA, miRNA-lncRNA, and competitive endogenous RNA (ceRNA) networks for CRC using online databases. Through these comprehensive bioinformatics analyses, we explored potential candidate biomarkers related to the diagnosis and prognosis of CRC.
We present the following article in accordance with the REMARK reporting checklist (available at https://jgo.amegroups.com/article/view/10.21037/jgo-21-921/rc).
Methods
Microarray data and identification of DEGs
To acquire the gene expression data sets of CRC, the microarray data were downloaded from the GEO database (https://www.ncbi.nlm.nih.gov/gds/). The data set was chosen because it met the following criteria: “CRC”, and “Expression profiling by array”, and “Homo sapiens”, and “tissues”. After comprehensive analysis, GSE151021 [platforms: GPL24592, Arraystar human SuperEnhancer microarray (Agilent-085154), and colorectal tumoral tissue: colorectal peritumoral tissue =4:4], GSE156720 [platforms: GPL26963, Arraystar human lncRNA V5 microarray (Agilent-085982), and colorectal tumoral tissue: colorectal peritumoral tissue =3:3], and GSE156719 [platforms: GPL20712, Agilent-070156 human miRNA (miRNA version), and colorectal tumoral tissue: colorectal peritumoral tissue =3:3] were downloaded, and selected. The DEGs were obtained from the GEO database by a way of GEO2R analysis (https://www.ncbi.nlm.nih.gov/geo/geo2r/). A P value <0.05 and a |log fold change| >1 were set as DEG cutoff criteria. The volcano plot was visualized by ‘ggplot2’ R package based on the expression values of the DEGs. All the data are available, free and open source, and the current study did not involve human or animal experiments. The study was conducted in accordance with the Declaration of Helsinki (as revised in 2013).
GO annotation and KEGG pathway enrichment analyses of DEGs
To examine the functions of DEGs, the online Database for Annotation, Visualization and Integrated Discovery (DAVID; https://david.ncifcrf.gov/) (9) was used to perform the GO and KEGG pathway enrichment analyses. The GO terms included the following 3 categories: biological process (BP), cellular component (CC), and molecular function (MF). To better understand how the DEGs are involved in the BPs, the cluster profiler R package was used in the GO and KEGG pathway analyses. A P value <0.05 was considered significant.
PPI network analysis of DEGs
The online tool String (https://cn.string-db.org/) (10) was applied to establish the DEG interaction network. To visualize the interaction of DEGs, Cytoscape 3.8.2 (11) was used to construct the network model. The top 10 hub genes from the PPI network were chosen by the plug-in cytoHubba. The Molecular Complex Detection (MCODE) was used to screen modules of the PPI network with the following criteria: cutoff =2, node score cutoff =0.2, k-core =2, and maximum depth =100.
Validation of hub genes
To evaluate the mRNA expression levels of the screened hub genes in the CRC and non-tumor samples, UALCAN online software (http://ualcan.path.uab.edu/) (12) and the GEPIA2 (http://gepia2.cancer-pku.cn/#index) (13) database was used to visualize the hub gene expression. A P value <0.05 was considered statistically significant.
Construction of the miRNA-mRNA, miRNA-lncRNA, and lncRNA-miRNA-mRNA networks
To explore the miRNA-mRNA interaction network, the miRCancer (http://mircancer.ecu.edu/index.jsp) (14) and TargetScan (http://www.targetscan.org/vert_72/) (15) databases were selected for prediction. Further, we determined the overlapping miRNAs of the predicted miRNAs and the DEmiRNAs of GSE156719 as potential target miRNAs for the DEGs. The miRNAs for which mRNA targets with the opposite expression patterns were selected for the miRNA-mRNA network construction. To determine the interactions between the miRNAs and lncRNAs, the DIANA-LncBase v2 (https://carolina.imis.athena-innovation.gr/diana_tools/web/) (16) and the GSE156720 data set were used to predict the common target lncRNAs of the potential miRNAs. The results were visualized using Cytoscape v3.8.2 software. Finally, based on the constructed miRNA-mRNA and miRNA-lncRNA networks, we established the lncRNA-miRNA-mRNA ceRNA network by Cytoscape v3.8.2.
Survival analyses for hub genes
KM plotter (https://kmplot.com/analysis/index.php?p=background) (17) has been widely used to explore the roles of 54K genes (mRNAs, miRNAs, and proteins) in survival in 21 cancer types. The source of KM plotter is GEO, European Genomephenome Archive (EGA), and TCGA databases, which include the mRNA of 165 rectum adenocarcinoma patients. The relationship between OS and the hub genes expressed in patients with CRC was revealed by KM survival analysis (17). Log-rank test results with a P value <0.05 were considered statistically significant.
Statistical analysis
The GraphPad Prism 7 was used for statistical analysis. The ANOVA was used to analyze statistical significance. Data were expressed as the mean ± SD. P<0.05 were regarded as statistically significant.
Results
Identification of DEGs
Based on the cutoff criteria, we identified 1,286 mRNAs (798 upregulated and 588 downregulated), 1,571 lncRNAs (778 upregulated and 793 downregulated) in GSE156720, 148 mRNA (99 upregulated and 49 downregulated) in GSE151021, and 127 miRNAs (71 upregulated and 56 downregulated) in GSE156719 as shown in the volcano plot (Figure 1A-1D). Next, we identified a total of 23 upregulated DEGs and 10 downregulated DEGs that were commonly expressed in GSE156720 and GSE151021 by a Venn diagram (Figure 1E).
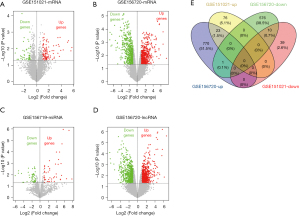
DEGs involved in CRC
To further evaluate gene functions of the identified DEGs, we performed GO and KEGG pathway analyses (Figure 2). The top 3 GO BPs were markedly enriched in the positive regulation of the intrinsic apoptotic signaling pathway, reactive oxygen species metabolic process, and responses to drugs (Figure 2A). For the GO CC/MF analysis, the extracellular space, nucleus, proteinaceous extracellular matrix, mitochondrion, and protein heterodimerization activity were significantly enriched (Figure 2B,2C). The KEGG enriched groups were cancer and the cytokine-cytokine receptor interaction (Figure 2D).
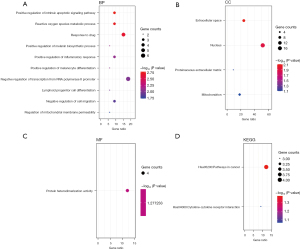
Construction PPI network of DEGs
The 1,286 DEmRNAs were analyzed by the online software String to construct the PPI network, and the isolated genes not involved in the interaction were removed.
The PPI network included 60 nodes and 529 edges. Next, the PPI network data were imported into Cytoscape for the visualization analysis (Figure 3A). The plug-in functions cytoHubba and MCODE were used to parse the network. The top 10 hub genes were not in the DEmRNAs (see Figure 3B). Additionally, the top 3 significant modules were chosen by running MCODE (see Figure 3C-3E), and 3 upregulated mRNAs [i.e., nucleolar protein 14 (NOP14), sonic hedgehog (SHH) signaling molecule, and phorbol-12-myristate-13-acetate-induced protein 1 (PMAIP1)], and 2 downregulated mRNAs [i.e., BCL2 apoptosis regulator (BCL2) and zinc finger E-box binding homeobox 2 (ZEB2)] were identified in the DEmRNAs.
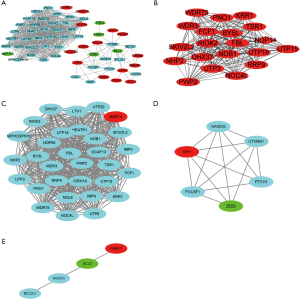
Validation of mRNA expression of the 5 hub genes in CRC
Based on the above results, we found that NOP14, SHH and PMAIP1 were upregulated in CRC, and BCL2 and ZEB2 were downregulated in CRC. To further validate the results, the UALCAN and GEPIA2 databases were analyzed. The results revealed that the mRNA expression of NOP14, SHH and PMAIP1 were significantly higher in the CRC tissues than the normal tissues (P<0.001; Figure 4A-4C), and the mRNA expression of ZEB2 and BCL2 were significantly lower in the CRC tissues than the normal tissues (P<0.001; Figure 4D,4E).
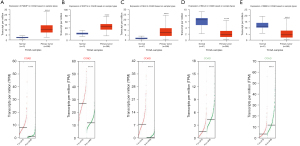
Prognostic values of the hub genes in CRC
The prognostic values of the 5 hub genes were determined through KM plotter. As Figure 5A shows, high expression levels of PMAIP1 were associated with better OS, but were not statistically significant. As Figure 5B shows, high expression levels of NOP14 in CRC patients were related to better OS (log-rank P=0.0024). As Figure 5C shows, high expression levels of SHH were associated with better OS, but were not statistically significant. As Figure 5D shows, high expression levels of BCL2 in CRC patients were related to better OS (log-rank P=0.0091). As Figure 5E shows, high expression levels of ZEB2 were associated with better OS, but were not statistically significant.
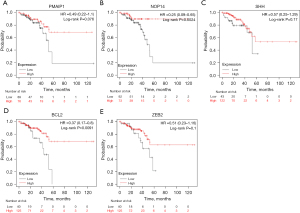
Prediction of the corresponding target miRNAs of the hub mRNAs
To construct the miRNA-mRNA network, miRcancer, TargetScan, and GSE156719 were used to screen the target miRNAs of NOP14, SHH, PMAIP1, ZEB2, and BCL2 in CRC. PMAIP1, NOP14, and SHH predicted 2, 3, and 2 downregulated target miRNAs, respectively (Figure 6A-6C). BCL2 and ZEB2 predicted 4 and 2 upregulated targeted miRNAs, respectively (Figure 6D,6E). The network of 4 upregulated miRNAs (i.e., hsa-miR-1246, hsa-miR-17-5p, hsa-miR-196b-5p, and hsa-miR-92a-3p) and 3 downregulated miRNAs (hsa-miR-145-5p, hsa-miR-193a-5p, and hsa-miR-485-5p) and their 5 shared identified mRNA targets were visualized by Cytoscape for the miRNA-mRNA interaction network (Figure 6F).
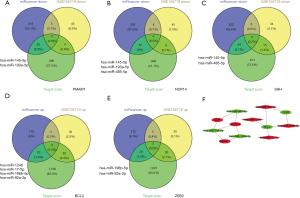
Prediction of miRNA-lncRNA interactions
The intended lncRNAs were those that met all the requirement for prediction by online software DIANA-LncBase v2 and chip array GSE156720. As a result, 21, 9, and 21 upregulated target lncRNAs of hsa-145-5p, hsa-miR-193a-5p, and hsa-miR-485-5p, respectively, were obtained based on the cutoff (Figure 7A-7C). 13, 17, 3, and 10 downregulated lncRNAs met the criteria for hsa-miR-1246, hsa-miR-17-5p, hsa-miR-196b-5p, and hsa-miR-92a-3p, respectively (Figure 7D-7G). The miRNA-upregulated lncRNAs and the miRNA downregulated lncRNA networks are shown in Figure 7H.
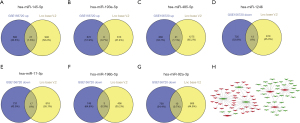
Construction of the lncRNA-miRNA-mRNA interactions network in CRC
To further understand the pivotal roles of the DEmiRNA, DElncRNA, and DEmRNA in CRC, the ceRNA network was constructed. The network comprised 91 nodes and 107 edges, including 7 miRNAs (4 upregulated and 3 downregulated), 79 lncRNAs (40 upregulated and 39 downregulated), and 5 mRNAs (3 upregulated and 2 downregulated). The entire lncRNA-miRNA- mRNA ceRNA regulatory network was visualized by Cytoscape (version 3.8.2) (Figure 8).
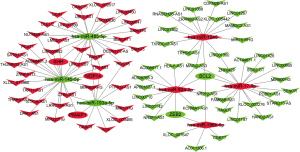
Discussion
In the present research, we systematically analyzed 3 microarray data sets from the GEO database. A total of 33 DEGs (23 upregulated and 10 downregulated) were identified by comparing the CRC and para-carcinoma tissues. The GO annotation enrichment analyses revealed that the DEGs were enriched in BPs that can play a role in tumor development, such as the positive regulation of intrinsic apoptotic signaling pathway, the reactive oxygen species metabolic process, the negative regulation of cell migration, and cell proliferation. These results are consistent with the previous understanding that the gain or loss of these functions plays a major role in the occurrence and progression of CRC (18). The KEGG pathway analysis revealed that the DEGs were obviously related to pathways in cancer and cytokine-cytokine receptor interactions. Cytokines are small proteins secreted by cells to regulate the immune and inflammatory responses. By binding with specific receptor proteins on the cell membrane, cytokines act on target cells, regulate cell growth and differentiation, and participate in immune, inflammatory responses, and wound healing (19). Our findings revealed that these DEGs may affect the occurrence and prognosis of CRC.
A PPI network was constructed by the DEGs and 5 hub genes were identified from the DEGs; that is, NOP14, SHH, PMAIP1, BCL2, and ZEB2. We then validated their RNA expression levels, and the results were consistent with those of the previous analysis. Additionally, we conducted survival analyses on the hub genes to determine the prognostic values of the genes. Further, the 5 hub mRNAs predicted the mRNA-miRNA paired targets by miRcancer, TargetScan, and GSE156719. 13 mRNA-miRNA paired targets were identified.
NOP14 encodes a protein that plays a role in pre-18S ribosomal RNA (rRNA) processing and small ribosomal subunit assembly (20). Previous research has reported that NOP14 is overexpressed in CRC, and promotes the proliferation, growth, and metastasis of CRC cells (21). The SHH signaling molecule is instrumental in patterning early embryos and regulating growth in different tissues (22). Farooqi et al. reported that the hedgehog (HH)/GLISHH signaling molecule/GLI signaling pathway was upregulated in and associated with the development and progression of CRC (23). BCL2 and PMAIP1, which belongs to the BCL2 homology domain 3 (BH3) only subfamily, play a role in determining whether a cell commits to apoptosis (24) in cancers, including CRC, lung cancer, and acute myeloid leukemia (25-27). Consistent with our results, BCL2 has also been shown to be decreased in CRC and PMAIP1 increased in CRC (28,29).
ZEB2 is located in the nucleus and functions as a deoxyribonucleic acid-binding transcriptional repressor that interacts with activated SMADs, and is mainly involved in epithelial-to-mesenchymal transition (EMT) (30,31). EMT is a conserved process during which a mature and adherent epithelial-like state is converted into a mobile mesenchymal state. There is emerging evidence that ZEB2 plays a major role in EMT-induced processes, such as differentiation, development, metastasis, cell-cycle arrest, drug resistance, cancer stem cell-like traits, tumor recurrence, and survival (32). Yan et al. reported that ZEB2 was downregulated in CRC (33), which we further verified in our study. Moreover, miR-145-5p, miR-193a-5p, and miR-485-5p were downregulated in CRC, consistent with their reported roles in cell proliferation, migration, invasion, and tumorigenesis (34-42). Additionally, consistent with previous results, miR-196b-5p, miR-17-5p, miR-92a-3p, and miR-1246 were more augmented in CRC tissues than the adjacent tissues and promoted CRC progression (43-47). These results also indicated that these miRNA-mRNA pairs act synergistically in CRC.
GSE156720 and LncBase V2 were used to predict the miRNA-lncRNA interactions. Based on the mRNA-miRNA network and miRNA-lncRNA network, the ceRNA network was constructed. The network elucidates the potential molecular mechanisms by which each member in the network is involved in the CRC pathobiology. The miRNAs bind to the 3' untranslated region of the target mRNAs and regulate their expression level. Conversely, miRNAs may be targeted by lncRNAs, which can act as miRNA sponges and attenuate their regulatory effect on the target mRNAs (48,49). There is extensive research that the ceRNA network is related to the progression of CRC. For example, lncRNA SNHG6 interacts with miR-26a/26b/214 and regulates their common target EZH2 in CRC (50). LINC01278 also accelerates CRC progression via miR-134-5p (51), and lncRNA SNHG1 regulates CRC cell growth through miR-154-5p (52). In our study, the expression levels of SNHG16, HCG18, and CASC9 were significantly higher in CRC tissues than the peritumoral tissues, consistent with their role in tumorigenesis (53-55). Other lncRNAs, such as HAND2-AS1 and NR2F1-AS1, were downregulated in CRC (56,57). In sum, the ceRNA networks are related to CRC and represent the interactions between lncRNAs, miRNAs, and mRNAs.
Conclusions
In summary, we undertook a systematic analysis of the molecules involved in CRC, including lncRNAs, miRNAs, and mRNAs, and showed potential interaction networks in which these molecules may cotribute to CRC development and patient survival. Further studies need to be conducted to determine how these molecules affect the development and progression of CRC.
Acknowledgments
Funding: This project was supported by the National Natural Science Foundation of Xinjiang Uygur Autonomous Region (Nos. 2021D01C396 and 2017D01C385).
Footnote
Reporting Checklist: The authors have completed the REMARK reporting checklist. Available at https://jgo.amegroups.com/article/view/10.21037/jgo-21-921/rc
Conflicts of Interest: All authors have completed the ICMJE uniform disclosure form (available at https://jgo.amegroups.com/article/view/10.21037/jgo-21-921/coif). XZ and LN are from CheerLand Clinical Laboratory Co., Ltd. LN and CC are from Shenzhen Cheerland Biotechnology Co., Ltd. The other authors have no conflicts of interest to declare.
Ethical Statement: The authors are accountable for all aspects of the work in ensuring that questions related to the accuracy or integrity of any part of the work are appropriately investigated and resolved. The study was conducted in accordance with the Declaration of Helsinki (as revised in 2013).
Open Access Statement: This is an Open Access article distributed in accordance with the Creative Commons Attribution-NonCommercial-NoDerivs 4.0 International License (CC BY-NC-ND 4.0), which permits the non-commercial replication and distribution of the article with the strict proviso that no changes or edits are made and the original work is properly cited (including links to both the formal publication through the relevant DOI and the license). See: https://creativecommons.org/licenses/by-nc-nd/4.0/.
References
- Sung H, Ferlay J, Siegel RL, et al. Global Cancer Statistics 2020: GLOBOCAN Estimates of Incidence and Mortality Worldwide for 36 Cancers in 185 Countries. CA Cancer J Clin 2021;71:209-49. [Crossref] [PubMed]
- Biller LH, Schrag D. Diagnosis and Treatment of Metastatic Colorectal Cancer: A Review. JAMA 2021;325:669-85. [Crossref] [PubMed]
- Siegel RL, Miller KD, Goding Sauer A, et al. Colorectal cancer statistics, 2020. CA Cancer J Clin 2020;70:145-64. [Crossref] [PubMed]
- Dekker E, Tanis PJ, Vleugels JLA, et al. Colorectal cancer. Lancet 2019;394:1467-80. [Crossref] [PubMed]
- Ahluwalia P, Kolhe R, Gahlay GK. The clinical relevance of gene expression based prognostic signatures in colorectal cancer. Biochim Biophys Acta Rev Cancer 2021;1875:188513. [Crossref] [PubMed]
- Tang Q, Chen J, Di Z, et al. TM4SF1 promotes EMT and cancer stemness via the Wnt/β-catenin/SOX2 pathway in colorectal cancer. J Exp Clin Cancer Res 2020;39:232. [Crossref] [PubMed]
- Li Q, Pan Y, Cao Z, et al. Comprehensive Analysis of Prognostic Value and Immune Infiltration of Chromobox Family Members in Colorectal Cancer. Front Oncol 2020;10:582667. [Crossref] [PubMed]
- Li C, Sun YD, Yu GY, et al. Integrated Omics of Metastatic Colorectal Cancer. Cancer Cell 2020;38:734-47.e9. [Crossref] [PubMed]
- Jiao X, Sherman BT. DAVID-WS: a stateful web service to facilitate gene/protein list analysis. Bioinformatics 2012;28:1805-6. [Crossref] [PubMed]
- Szklarczyk D, Gable AL, Lyon D, et al. STRING v11: protein-protein association networks with increased coverage, supporting functional discovery in genome-wide experimental datasets. Nucleic Acids Res 2019;47:D607-13. [Crossref] [PubMed]
- Shannon P, Markiel A, Ozier O, et al. Cytoscape: a software environment for integrated models of biomolecular interaction networks. Genome Res 2003;13:2498-504. [Crossref] [PubMed]
- Chandrashekar DS, Bashel B, Balasubramanya SAH, et al. UALCAN: A Portal for Facilitating Tumor Subgroup Gene Expression and Survival Analyses. Neoplasia 2017;19:649-58. [Crossref] [PubMed]
- Tang Z, Kang B, Li C, et al. GEPIA2: an enhanced web server for large-scale expression profiling and interactive analysis. Nucleic Acids Res 2019;47:W556-60. [Crossref] [PubMed]
- Xie B, Ding Q, Han H, et al. miRCancer: a microRNA-cancer association database constructed by text mining on literature. Bioinformatics 2013;29:638-44. [Crossref] [PubMed]
- Agarwal V, Bell GW, Nam JW, et al. Predicting effective microRNA target sites in mammalian mRNAs. Elife 2015;4:e05005. [Crossref] [PubMed]
- Paraskevopoulou MD, Vlachos IS, Karagkouni D, et al. DIANA-LncBase v2: indexing microRNA targets on non-coding transcripts. Nucleic Acids Res 2016;44:D231-8. [Crossref] [PubMed]
- Győrffy B. Survival analysis across the entire transcriptome identifies biomarkers with the highest prognostic power in breast cancer. Comput Struct Biotechnol J 2021;19:4101-9. [Crossref] [PubMed]
- Markowitz SD, Bertagnolli MM. Molecular origins of cancer: Molecular basis of colorectal cancer. N Engl J Med 2009;361:2449-60. [Crossref] [PubMed]
- Dey R, Ji K, Liu Z, et al. A cytokine-cytokine interaction in the assembly of higher-order structure and activation of the interleukine-3:receptor complex. PLoS One 2009;4:e5188. [Crossref] [PubMed]
- Du Y, Liu Z, You L, et al. Pancreatic Cancer Progression Relies upon Mutant p53-Induced Oncogenic Signaling Mediated by NOP14. Cancer Res 2017;77:2661-73. [Crossref] [PubMed]
- Zhu X, Jia W, Yan Y, et al. NOP14 regulates the growth, migration, and invasion of colorectal cancer cells by modulating the NRIP1/GSK-3β/β-catenin signaling pathway. Eur J Histochem 2021;65:3246. [Crossref] [PubMed]
- Skoda AM, Simovic D, Karin V, et al. The role of the Hedgehog signaling pathway in cancer: A comprehensive review. Bosn J Basic Med Sci 2018;18:8-20. [Crossref] [PubMed]
- Farooqi AA, de la Roche M, Djamgoz MBA, et al. Overview of the oncogenic signaling pathways in colorectal cancer: Mechanistic insights. Semin Cancer Biol 2019;58:65-79. [Crossref] [PubMed]
- Morsi RZ, Hage-Sleiman R, Kobeissy H, et al. Noxa: Role in Cancer Pathogenesis and Treatment. Curr Cancer Drug Targets 2018;18:914-28. [Crossref] [PubMed]
- Zhao Q, Zhong J, Bi Y, et al. Gambogenic acid induces Noxa-mediated apoptosis in colorectal cancer through ROS-dependent activation of IRE1α/JNK. Phytomedicine 2020;78:153306. [Crossref] [PubMed]
- Nechiporuk T, Kurtz SE, Nikolova O, et al. The TP53 Apoptotic Network Is a Primary Mediator of Resistance to BCL2 Inhibition in AML Cells. Cancer Discov 2019;9:910-25. [Crossref] [PubMed]
- Do H, Kim D, Kang J, et al. TFAP2C increases cell proliferation by downregulating GADD45B and PMAIP1 in non-small cell lung cancer cells. Biol Res 2019;52:35. [Crossref] [PubMed]
- Ramesh P, Medema JP. BCL-2 family deregulation in colorectal cancer: potential for BH3 mimetics in therapy. Apoptosis 2020;25:305-20. [Crossref] [PubMed]
- Jeong S, Yun HK, Jeong YA, et al. Cannabidiol-induced apoptosis is mediated by activation of Noxa in human colorectal cancer cells. Cancer Lett 2019;447:12-23. [Crossref] [PubMed]
- Birkhoff JC, Huylebroeck D, Conidi A. ZEB2, the Mowat-Wilson Syndrome Transcription Factor: Confirmations, Novel Functions, and Continuing Surprises. Genes (Basel) 2021;12:1037. [Crossref] [PubMed]
- Fardi M, Alivand M, Baradaran B, et al. The crucial role of ZEB2: From development to epithelial-to-mesenchymal transition and cancer complexity. J Cell Physiol 2019; Epub ahead of print. [Crossref] [PubMed]
- Vandewalle C, Comijn J, De Craene B, et al. SIP1/ZEB2 induces EMT by repressing genes of different epithelial cell-cell junctions. Nucleic Acids Res 2005;33:6566-78. [Crossref] [PubMed]
- Yan Z, Bi M, Zhang Q, et al. LncRNA TUG1 promotes the progression of colorectal cancer via the miR-138-5p/ZEB2 axis. Biosci Rep 2020;40:BSR20201025. [Crossref] [PubMed]
- Chen ZL, Li XN, Ye CX, et al. Elevated Levels of circRUNX1 in Colorectal Cancer Promote Cell Growth and Metastasis via miR-145-5p/IGF1 Signalling. Onco Targets Ther 2020;13:4035-48. [Crossref] [PubMed]
- Chen Q, Zhou L, Ye X, et al. miR-145-5p suppresses proliferation, metastasis and EMT of colorectal cancer by targeting CDCA3. Pathol Res Pract 2020;216:152872. [Crossref] [PubMed]
- Niu Y, Zhang J, Tong Y, et al. miR-145-5p restrained cell growth, invasion, migration and tumorigenesis via modulating RHBDD1 in colorectal cancer via the EGFR-associated signaling pathway. Int J Biochem Cell Biol 2019;117:105641. [Crossref] [PubMed]
- Azar MRMH, Aghazadeh H, Mohammed HN, et al. miR-193a-5p as a promising therapeutic candidate in colorectal cancer by reducing 5-FU and Oxaliplatin chemoresistance by targeting CXCR4. Int Immunopharmacol 2021;92:107355. [Crossref] [PubMed]
- Xu H, Liu Y, Cheng P, et al. CircRNA_0000392 promotes colorectal cancer progression through the miR-193a-5p/PIK3R3/AKT axis. J Exp Clin Cancer Res 2020;39:283. [Crossref] [PubMed]
- Wei R, Chen L, Qin D, et al. Liquid Biopsy of Extracellular Vesicle-Derived miR-193a-5p in Colorectal Cancer and Discovery of Its Tumor-Suppressor Functions. Front Oncol 2020;10:1372. [Crossref] [PubMed]
- Wang X, Tao G, Huang D, et al. Circular RNA NOX4 promotes the development of colorectal cancer via the microRNA-485-5p/CKS1B axis. Oncol Rep 2020;44:2009-20. [Crossref] [PubMed]
- Liu J, Zhang J, Wang Z, et al. Knockdown of circAPLP2 Inhibits Progression of Colorectal Cancer by Regulating miR-485-5p/FOXK1 Axis. Cancer Biother Radiopharm 2021;36:737-52. [Crossref] [PubMed]
- Pan Y, Sun H, Hu X, et al. The inhibitory role of miR-485-5p in colorectal cancer proliferation and invasion via targeting of CD147. Oncol Rep 2018;39:2201-8. [Crossref] [PubMed]
- Xin H, Wang C, Chi Y, et al. MicroRNA-196b-5p promotes malignant progression of colorectal cancer by targeting ING5. Cancer Cell Int 2020;20:119. [Crossref] [PubMed]
- Stiegelbauer V, Vychytilova-Faltejskova P, Karbiener M, et al. miR-196b-5p Regulates Colorectal Cancer Cell Migration and Metastases through Interaction with HOXB7 and GALNT5. Clin Cancer Res 2017;23:5255-66. [Crossref] [PubMed]
- Xu J, Meng Q, Li X, et al. Long Noncoding RNA MIR17HG Promotes Colorectal Cancer Progression via miR-17-5p. Cancer Res 2019;79:4882-95. [Crossref] [PubMed]
- Guan B, Li G, Wan B, et al. RNA-binding protein RBM38 inhibits colorectal cancer progression by partly and competitively binding to PTEN 3'UTR with miR-92a-3p. Environ Toxicol 2021;36:2436-47. [Crossref] [PubMed]
- Peng W, Li J, Chen R, et al. Upregulated METTL3 promotes metastasis of colorectal Cancer via miR-1246/SPRED2/MAPK signaling pathway. J Exp Clin Cancer Res 2019;38:393. [Crossref] [PubMed]
- Fang Z, Rajewsky N. The impact of miRNA target sites in coding sequences and in 3'UTRs. PLoS One 2011;6:e18067. [Crossref] [PubMed]
- Poliseno L, Salmena L, Zhang J, et al. A coding-independent function of gene and pseudogene mRNAs regulates tumour biology. Nature 2010;465:1033-8. [Crossref] [PubMed]
- Xu M, Chen X, Lin K, et al. lncRNA SNHG6 regulates EZH2 expression by sponging miR-26a/b and miR-214 in colorectal cancer. J Hematol Oncol 2019;12:3. [Crossref] [PubMed]
- Xi C, Ye NY, Wang YB. LncRNA LINC01278 accelerates colorectal cancer progression via miR-134-5p/KDM2A axis. Eur Rev Med Pharmacol Sci 2020;24:10526-34. [PubMed]
- Xu M, Chen X, Lin K, et al. The long noncoding RNA SNHG1 regulates colorectal cancer cell growth through interactions with EZH2 and miR-154-5p. Mol Cancer 2018;17:141. [Crossref] [PubMed]
- Li S, Wu T, Zhang D, et al. The long non-coding RNA HCG18 promotes the growth and invasion of colorectal cancer cells through sponging miR-1271 and upregulating MTDH/Wnt/β-catenin. Clin Exp Pharmacol Physiol 2020;47:703-12. [Crossref] [PubMed]
- Chen ZY, Wang XY, Yang YM, et al. LncRNA SNHG16 promotes colorectal cancer cell proliferation, migration, and epithelial-mesenchymal transition through miR-124-3p/MCP-1. Gene Ther 2020; Epub ahead of print. [Crossref] [PubMed]
- Luo K, Geng J, Zhang Q, et al. LncRNA CASC9 interacts with CPSF3 to regulate TGF-β signaling in colorectal cancer. J Exp Clin Cancer Res 2019;38:249. [Crossref] [PubMed]
- Gu X, Zheng Q, Chu Q, et al. HAND2-AS1: A functional cancer-related long non-coding RNA. Biomed Pharmacother 2021;137:111317. [Crossref] [PubMed]
- Wang J, Dong S, Zhang J, et al. LncRNA NR2F1-AS1 Regulates miR-371a-3p/TOB1 Axis to Suppress Proliferation of Colorectal Cancer Cells. Cancer Biother Radiopharm 2020;35:760-4. [Crossref] [PubMed]
(English Language Editor: L. Huleatt)