Construction of a competing endogenous RNA network related to the prognosis of cholangiocarcinoma and comprehensive analysis of the immunological correlation
Introduction
Cholangiocarcinoma (CCA) is a malignant tumor originating from bile duct epithelial cells. The incidence of CCA is low, accounting for approximately 3% of all gastrointestinal tumors (1). CCA has an insidious onset and is difficult to diagnose in the early stage, and is thus often found at an advanced stage. In the early stage of CCA, lymph node metastasis, vascular invasion, and nerve invasion are more likely to occur. Statistics from recent years indicate that the average survival time after diagnosis is no more than 24 months (2), with a poor prognosis. Surgery is the only effective treatment at present, but most patients have lost the chance of radical surgery because they are already in the advanced stage when diagnosed (3). For patients with advanced, gemcitabine combined cisplatin chemotherapy is the standard first-line treatment, but the median survival period is still less than 1 year (4-6), at present, some retrospective study showed that the synchronization of the radiation and chemotherapy can make the part not resection of intrahepatic bile duct carcinoma patients benefit, but for distal bile duct carcinoma and liver bile duct carcinoma, its curative effect remains to be evaluate (7), Currently, molecular targeted therapies that have made great progress in the treatment of liver cancer are mostly in the stage of clinical trials for CCA. In 2020, FDA approved the first molecular targeted drug Pemigatinib, which has achieved encouraging results (8). Several current clinical studies suggest that PD-1 monoclonal antibody (mAb) pembrolizumab is effective in some patients with advanced biliary tract cancer (9). Therefore, the exploration of new prognostic factors and immunorelated therapeutic targets in CCA remains an urgent issue.
In recent years, the importance of non-coding RNA in the occurrence and development of tumors has attracted increasing attention. In 2011, Salmena et al. (10) proposed the hypothesis of competing endogenous RNA (ceRNA) for the first time, suggesting that in addition to the traditional miRNA→mRNA regulatory mechanism, there is also an inverse mRNA→miRNA regulatory effect. That is, non-coding RNA can achieve mRNA regulation by competing with coding RNA and binding to miRNA. There is growing evidence that circular RNAs (circRNAs) can act as a sponge to adsorb miRNA as part of ceRNA, in order to mitigate miRNA inhibition of its target (11). CircRNA is derived from the reverse splicing of precursor mRNA, has a circular covalent closed structure, with a high tolerance to exonuclease, and is more stable than the general linear non-coding RNA structure (12). Piwecka et al. (13) studied the regulatory effect of circRNA on miRNA in mammalian brain tissue, and revealed that circRNA can act as a molecular sponge for miRNA to regulate the expression of its target genes.
Tumor microenvironment (TME) refers to the internal environment of tumor occurrence and development. The genesis and progression of tumor is largely determined by the interaction between cancer cells and non-cancer components in the TME. TME not only affects tumor development, but also the effect of anti-tumor therapy (14). CCA’s TME is composed of infiltrating immune cells, tumor-associated fibroblasts, and a variety of extracellular components that promote tumor progression, which can influence tumor progression and treatment resistance (15). Among them, the decrease of adaptive immune response cells and the accumulation of innate immune cells were correlated with the poor prognosis and gemcitabine resistance after recurrence of CCA (16). For example, tumor-associated macrophages (TAMs) promote tumor growth and metastasis by secreting tumor-promoting factors. Activation of CD4+ and CD8+ T cells has an anti-tumor effect, however, TME can inhibit this anti-tumor effect by inhibiting T cell infiltration, decreasing antigen presentation, and lymphocyte activation (15). With the rapid development of immunotherapy, the application of immune checkpoint (ICP) inhibitors has presented significant survival benefits to patients with a variety of malignant tumors; however, only a small number of relevant studies are related to CCA. Meanwhile, accurate prediction of the efficacy of immunotherapy in CCA requires further exploration, in order to identify immunotherapeutic markers. It has been confirmed that key notional factors in the ceRNA network may affect the proportion of tumor-infiltrating immune cells (TICs) in the TME and the efficacy of immunotherapy. For example, Chang et al. (17) showed that ceRNAs (FAS, hsa-mir125b-5P) and TICs (T-cell follicular helper cells, macrophage M0) may be associated with the distant metastasis and prognosis of colon adenocarcinoma. Huang et al. (18) found that the RP11-1094m14.8/mir-1269a/CXCL9 axis may be a potential immunotherapeutic target for gastric cancer patients with different degrees of immune cell infiltration. However, there are few studies on the immune infiltration patterns of ceRNA and CAA, and most of the current studies take long non-coding RNA (lncRNA) as the starting point to establish ceRNA networks and conduct immune infiltration analysis, but there remains a lack of relevant studies focusing on circRNA. Therefore, we established the ceRNA network of circRNA-miRNA-mRNA and conducted immune correlation analysis. We proposed that hsa-circ-0002073 regulates the expression of SLC16A3 through sponge adsorption of hsa-miR-4524A-3p, thus affecting the TME of CCA and immune infiltrating cells in CCA and affecting the prognosis of patients, which is an innovation of our study. In this study, a circRNA-miRNA-mRNA-related ceRNA network was established based on the Gene Expression Omnibus (GEO) and The Cancer Genome Atlas (TCGA) databases, and the prognosis-related subnetwork was constructed based on the clinical data of TCGA database. Meanwhile, the TME and immune infiltration of CCA were also analyzed. Correlation analysis of immune cells and ICPs was conducted according to the genes in the prognosis subnets of ceRNA, in order to explore the relationship between the mechanism of CCA and the pattern of immune infiltration. Our study elucidated new mechanism of CCA development, discovered markers associated with the poor prognosis of CCA, and determined the associated ICP, which may provide an important basis for the immunotherapy and efficacy evaluation of CCA.
We present the following article in accordance with the REMARK reporting checklist (available at https://dx.doi.org/10.21037/jgo-21-619).
Methods
Acquisition of clinical characteristics and RNA data
CircRNA expression data of CCA were obtained from the GEO database (https://www.ncbi.nlm.nih.gov/geo). CircRNA expression data were obtained from GSE148561 (six pairs of CCA and paracancer tissues). The clinical and RNA expression data of 41 CCA patients were obtained from the TCGA database (https://portal.gdc.cancer.gov/), including 33 CCA samples and 8 adjacent normal samples. The study conformed to the provisions of the Declaration of Helsinki (as revised in 2013).
Identification of differentially expressed circRNAs, miRNAs, and survival-related prognostic mRNAs
Through Rstudio software, we used the limma bioconductor packages and edgeR bioconductor packages to explore the significantly differentially expressed mRNAs, circRNAs, and miRNAs between CCA tissues and adjacent normal tissues. Fold-change was used to measure the degree of change. For P values, the false discovery rate (FDR) was applied to determine the significance threshold for multiple tests.
The cut-off value was |log2FC| >1 for circRNA, false discovery rate (FDR) <0.05 was considered significant [called differentially expressed circRNA (DEcircRNA), differentially expressed miRNA (DEmiRNA)].
The mRNA and miRNA expressions of data downloaded from TCGA database meeting |log2FC| >1 and FDR <0.05 were considered differential genes. Based on TCGA database, we combined the transcriptomic data of the above differential mRNA with relevant clinical information, and performed univariate Cox regression analysis for each differential mRNA to evaluate the relationship between survival rate and differential mRNA expression level, and to obtain high-risk genes [hazard ratio (HR) >1, P<0.05]. According to the median value of the expression of each gene, the patients were divided into two groups with high and low expression. The survival curve was plotted using the Kaplan-Meier (KM) method according to the survival time and survival status. P<0.05 was considered a high-risk target gene [differentially expressed mRNA (DEmRNA)] with survival significance.
Construction of circRNA-miRNA-mRNA networks associated with survival
According to the results of the differential expression analysis, the circBase database (http://www.circbase.org/) was used to obtain the information of the top 10 highly-expressed circRNAs with significant differences in the above circRNA (DEcircRNA). The Cancer-Specific CircRNA Database (CSCD, http://gb.whu.edu.cn/CSCD/) analysis of the structure and the target of microRNAs DEcircRNA (T-microRNAs) was then predicted. Next, the target miRNA (T-miRNA) was intersected with the aforementioned DEmiRNA.
In order to extract the common mRNAs and the construct miRNA-mRNA network, we used the TargetScan (TargetScanHuman 7.1) database to predict target mRNAs in the above intersection, and selected target mRNAs confirmed in the database. The intersection between the predicted results and the DEmRNA selected from the above TCGA database was taken.
According to ceRNA theory, circRNA regulates mRNA expression by acting as a miRNA molecular sponge; that is, circRNA is negatively correlated with the expression of its target miRNA, and miRNA is negatively correlated with the expression of its target mRNA. Based on this principle, we constructed the circRNA-miRNA-mRNA regulatory network using the aforementioned-screened circRNA, miRNA, and mRNA. Finally, the regulatory network was visualized using Cytoscape 3.5.0 software (https://cytoscape.org/).
Construction of the ceRNA prognostic subnetwork
In order to understand whether mRNA in the ceRNA network is an independent prognostic factor for CCA patients, we combined the transcriptomic data of TCGA database with clinical data, performed single gene paired difference analysis and multivariate Cox regression analysis on the mRNA in the network, and screened out the independent prognostic factors. At the same time, we combined the transcriptome data of miRNAs in the network with clinical data in TCGA database to perform mass survival analysis and screen out the DEmiRNAs related to survival. Finally, we completed the construction of the ceRNA prognostic subnetwork using the independent prognostic factors and survivor-related miRNAs, and visualized the subnetwork using Cytoscape 3.5.0 software.
Analysis of the TME in CCA
In order to understand the TME in CCA, the “Estimate” package was used to score stromal and immune cells in the TME of CCA based on the transcriptomic data of CCA in TCGA. Patients were divided into high and low groups according to the median scores of stromal and immune cells, respectively, and the differential genes between the two groups were compared. This was combined with survival data from TCGA for survival analysis. To elucidate the expression of TICs in CCA and adjacent tissues, the CIBERSORT algorithm was used to calculate the scores of 22 TICs in each CCA sample based on TCGA RNA-seq data, and the correlation between these 22 TICs was evaluated.
Pan-cancer analysis of target genes and the correlation between target genes, immune cells and ICPs
In order to understand whether the target genes in the ceRNA prognostic subnetwork of CCA are also differentially expressed in other types of tumor tissues, we performed a pan-cancer cancer analysis of mRNAs in the prognostic subnetwork based on the UCSC Xena platform (https://xenabrowser.net/datapages), which was verified using the ONCOMINE database. At the same time, TIMER2.0 (http://timer.cistrome.org/) was used to analyze the correlation between target genes and immune cells, the target genes and six immune check points (CD274, PDCD1, CTLA4, HAVCR2, IL10RB, and TGFBR1) obtained by retrieval, and obtain the correlation scatter plot. Finally, we further verified the protein expression levels of the above genes in CCA using the Human Protein Atlas (HPA https://www.proteinatlas.org) database.
Statistical analysis
The differences among variables were tested using the exactTest of Negative-Binomial Counts and eBayes. The survival curve was plotted by KM method, and compared by log-rank test. Cox proportional hazards model was utilized for analysis of prognostic factors. All data was statistically processed by software Rstudio 4.1.0, P<0.05 was considered statistically significant.
Results
Identification of DEcircRNA, DEmiRNA, and DEmRNA in CCA patients
The circRNA expression data of six CCA samples and six adjacent normal samples were downloaded from the GEO database. The miRNA and mRNA expression data of 33 CCA samples and 8 adjacent normal samples were downloaded from the TCGA project. According to differential analysis (as described above), 97 DEcircRNAs (76 up-regulated and 21 down-regulated), 212 DEmiRNAs (120 up-regulated and 92 down-regulated), and 6,780 differentially-expressed mRNAs (4,196 up-regulated and 2,584 down-regulated) were significantly dysregulated, and heat map was drawn (Figure 1A-1C).
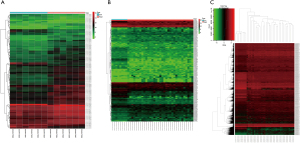
Univariate Cox regression analysis was performed on the above differentially-expressed mRNAs to obtain 96 prognostic factors. Next, an intermediate expression value of these prognostic RNAs was used as the cut-off point, the patients were divided into high- and low-expression groups, and KM survival analysis was performed. The results showed that there were significant survival differences between the two groups in the high and low expression of 29 genes (Figure 2).
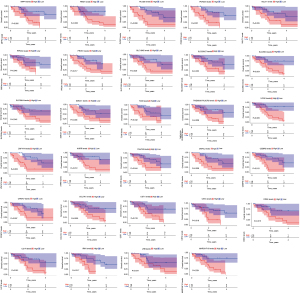
Construction of the ceRNA network
According to the significance of differential expression, we analyzed the top 10 significantly overexpressed DEcircRNAs using the circBase database to obtain their location information, and the CSCD database was used to obtain their structure diagrams and corresponding miRNA information. The results showed that nine circRNAs had miRNA responding elements (MREs). After visualizing the structure of the nine DEcircRNAs (Figure 3), 487 T-miRNAs of DEcircRNA were found using the CSCD database. The above 487 T-miRNAs were matched with 212 DEmiRNAs screened from TCGA database, and 25 intersection miRNAs were finally obtained (Figure 4A). Next, the TargetScan database was used to predict the target genes of the above 25 intersection miRNAs, and 17,747 T-mRNAs were obtained, which were then combined with 29 prognostic factors screened in TCGA database to obtain 25 mRNAs (Figure 4B). According to ceRNA theory, a total of four up-regulated DEcircRNAs, 10 down-regulated intersection miRNAs, and 18 up-regulated intersection mRNAs were selected to construct a competitive endogenous RNA network (Figure 4C), which contained 32 nodes and 54 links.
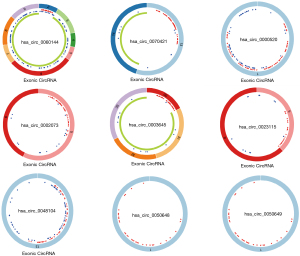
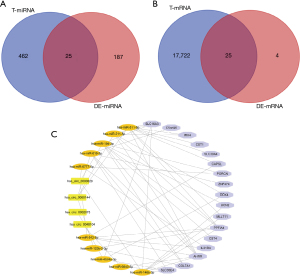
Construction of the prognostic subnetworks
We performed paired difference analysis and multivariate Cox regression analysis on 18 mRNAs in the ceRNA network, and the results showed that COL7A1, DEAD box polypeptide 4 (DDX4), IRX4, SLC16A3, SLC35E4, UCN2, and ZNF474 were independent prognostic factors and were high expression in tumor tissues compared with normal tissues (Figure 5). For the 10 miRNAs in ceRNA, patients were divided into high and low expression groups according to the median value of their gene expression levels. Survival curves were plotted using the KM method based on the survival time and survival status. The results showed that the 5-year survival rate of CCA patients with a high expression of hsa-miR-4524a-3p was higher than those with low expression (Figure 6A). Based on the seven independent prognostic factors related to survival and miRNAs with survival significance, combined with their corresponding circRNAs in ceRNA, the ceRNA prognostic subnetwork was plotted according to the ceRNA network theory (Figure 6B), which consisted of five nodes and four links. It was concluded that this circRNA likely acts as a miRNA molecular sponge in CCA and plays an important role in gene expression regulation.
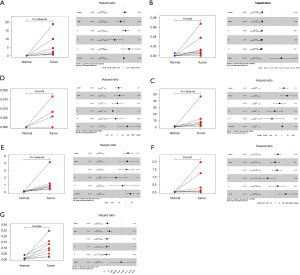
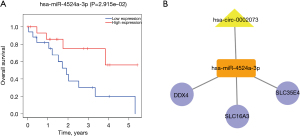
Analysis of the TME of CCA
The TME of CCA was scored based on the transcriptomic data of CCA in TCGA database. The patients were divided into high and low score groups according to the median stromal cell score, and 1,590 differential genes were obtained. The patients were also divided into high and low score groups according to the median immune cell score, and 1,067 differential genes were obtained. The top 50 high expression genes and 50 low expression genes with significant differences were selected to draw the heat map (Figure 7A,7B). We combined the scores of the TME with the clinical data in the TCGA database to conduct survival analysis of the TME. We obtained the survival curve of stromal and immune cells, as well as the survival curve of the sum of the two scores (Figure 7C-7E). A correlation was observed between the number of immune cells in the TME of CCA and patient survival time.
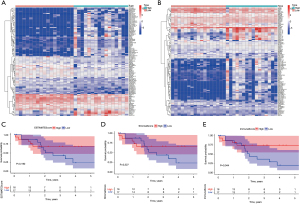
Distribution and correlation analysis of TICs in CCA
Since the immune cell scores in the TME of CCA were correlated with patient survival time, we evaluated TICs using the CIBERSORT deconvolution algorithm, constructed 22 immune cell profiles in CCA, and analyzed the proportion of TICs in each sample (Figure 8A). In addition, a positive correlation was found between activated natural killer (NK) cells and gamma delta T cells (r=0.76) by analyzing the correlation between immune cells in all tumor samples. Meanwhile, there was a significant negative correlation between regulatory T cells (Tregs) and resting CD4 memory T cells (r=−0.65) (Figure 8B).
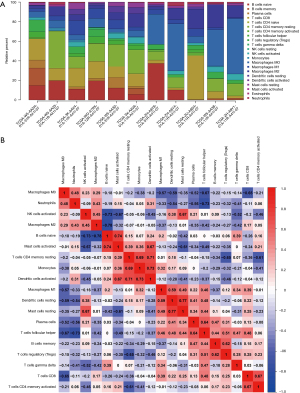
Pan-cancer analysis of target genes in the ceRNA prognostic subnetwork
In order to understand whether the target genes in the ceRNA prognostic subnetwork of CCA are also differentially expressed in other tumor tissue types, we analyzed the three target genes (SLC16A3, SLC35E4, and DDX4) in the ceRNA prognostic subnetwork based on the UCSC Xena database, and presented them in a box diagram (Figure 9A-9C). The results showed that SLC16A3 was significantly overexpressed in bladder urothelial carcinoma (BLCA), breast invasive carcinoma (BRCA), cholangiocarcinoma (CHOL), esophageal carcinoma (ESCA), glioblastoma multiforme (GBM), head and neck squamous cell carcinoma (HNSC), kidney renal clear cell carcinoma (KIRC), kidney renal papillary cell carcinoma (KIRP), liver hepatocellular carcinoma (LIHC), lung adenocarcinoma (LUAD), lung squamous cell carcinoma (LUSC), stomach adenocarcinoma (STAD), thyroid carcinoma (THCA), and uterine corpus endometrial carcinoma (UCEC), and markedly down-regulated in kidney chromophobe (KICH) and prostate adenocarcinoma (PRAD). SLC35E4 was notably highly expressed in BRCA, CHOL, colon adenocarcinoma (COAD), HNSC, KIRC, LIHC, LUAD, LUSC, rectum adenocarcinoma (READ), STAD, and THCA, and significantly down-regulated in GBM and PRAD. DDX4 was markedly overexpressed in BLCA, CHOL, KICH, LIHC, LUAD, LUSC, STAD, and UCEC, and considerably down-regulated in GBM, KIRP, and THCA.
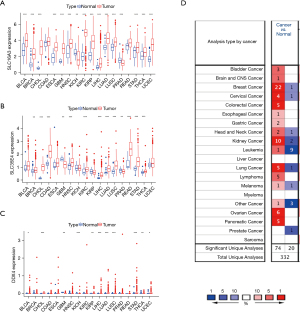
To further evaluate the expression of these target genes in pan-cancer, we used the ONCOMINE database to analyze the mRNA expression levels of SLC16A3, SLC35E4, and DDX4 between various tumor and normal tissues, and found that compared with normal tissues, SLC16A3 was highly expressed in bladder cancer, brain cancer, colorectal cancer, and other tumor tissues (Figure 9D).
Correlation analysis of target genes in the ceRNA subnetwork with tumor immune infiltrating cells and ICPs
Online analysis of the TIMER2.0 database demonstrated that mRNA expression in ceRNA subnetwork was correlated with the content of different types of immune cells (Figure 10A,10B). The specific results showed that SLC16A3 was significantly correlated with the levels of three TICs, which was positively correlated with the contents of resting myeloid dendritic cells (DCs) and neutrophils, and negatively correlated with the contents of CD4+ T cells. SLC35E4 was positively correlated with macrophages. These results further confirmed that the expression of the above mRNA significantly affected the composition of immune cells in the immune microenvironment of CCA.
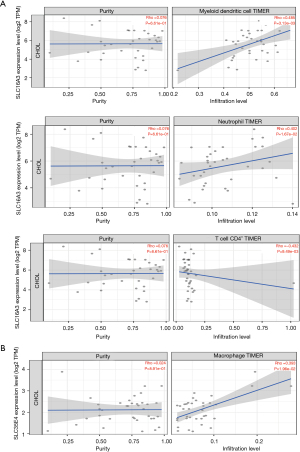
We used the same method to analyze the correlation between SLC16A3, SLC35E4, DDX4, and six ICPs (Figure 11A,11B). We found that SLC16A3 was positively correlated with CD274, HAVCR2, and TGFBR13, while DDX4 was negatively correlated with IL10RB. Therefore, we conclude that the key genes in the ceRNA prognostic subnetwork are likely to impact the effect of immunotherapy by regulating the types and functions of infiltrating immune cells in the tumor immune microenvironment, and that CCA patients with high SLC16A3 expression and low DDX4 expression may benefit from immunotherapy.
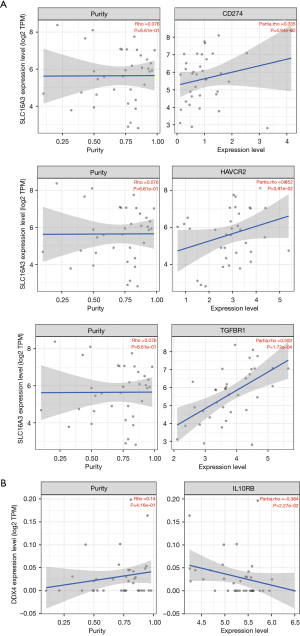
Validation of target gene expression in the ceRNA prognostic subnetwork of CCA
Through the above analysis, we found that in the ceRNA prognostic subnetwork, the target genes were correlated with immune cells and ICPs to varying degrees. Therefore, we used the HPA database to further verify the protein expression level of the target genes in CCA tissues. It was found that in CCA, the protein expression level of SLC16A3 was higher than that in normal tissues (Figure 12).
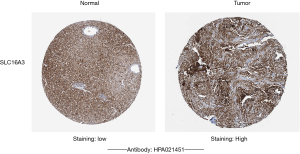
Discussion
CCA is the most common malignancy of the biliary system, accounting for 15–20% of primary hepatobiliary malignancies. Its incidence varies widely around the world, with Asia having the highest incidence (>80 per 100,000). Studies have shown that in recent years, its incidence in European and North American countries is on the rise (19). Due to its occult onset and high degree of malignancy (20), CCA is often at an advanced stage at the time of diagnosis, and surgical treatment is not feasible (4). Palliative chemotherapy is currently the only available option (5). For patients with unresectable or metastatic CCA, the historical 5-year survival rate is only 10–20% (21-23). Therefore, exploring the molecular mechanism of CCA is particularly importance for early diagnosis and personalized treatment.
At present, a large number of studies have reported that the ceRNA network may contribute to elucidating the mechanism of tumorigenesis (24), and that circRNAs, lncRNA, miRNAs, and mRNAs involved in the ceRNA network can be further used as potential therapeutic targets and prognostic biomarkers for tumors. However, the circRNA-based ceRNA network combined with TME analysis is relatively rare for CCA. Therefore, we constructed a circRNA-miRNA-mRNA network and a prognostic subnetwork based on multivariate Cox regression analysis. The relationship between the target genes in the network, TICs and ICPs was analyzed in order to identify new molecular mechanisms and prognostic molecular markers in CCA development, and to discover novel indicators to determine whether patients with CCA can benefit from immunotherapy.
In our study, we found that there were significant differences in some RNAs between the cancer and paracancer tissues of CCA patients. Combined with clinical data, 96 prognostic factors were screened out, and survival analysis was successively performed on each factor to obtain 29 survival-related genes. Finally, a ceRNA network containing four up-regulated circRNAs, 10 down-regulated miRNAs, and 18 up-regulated mRNAs was constructed. According to the multivariate Cox regression analysis of mRNA in the ceRNA network, we obtained seven independent prognostic factors, including COL7A1, DDX4, IRX4, SLC16A3, SLC35E4, UCN2, and ZNF474. Survival analysis of 10 miRNAs in the ceRNA network was performed, and survival-related hsa-miR-4524a-3p was obtained. Therefore, a prognostic ceRNA subnetwork containing one circRNA (hsa_circ_0002073), one miRNA (hsa-miR-4524a-3p), and three mRNAs (SLC16A3, SLC35E4, and DDX4) was constructed.
Next, we analyzed each gene in the prognostic subnetwork. SLC16A3, also known as MCT4, is a member of the monocarboxylic acid transporter (MCT) family. It has been found to be highly expressed in a variety of malignant tumor tissues, such as renal cell renal carcinoma, hepatocellular carcinoma, osteosarcoma, and is associated with patient prognosis (25-27). DDX4 is an ATP-dependent RNA helicase belonging to the DEAD box protein family, and is also expressed by spermatogonial progenitors. It is involved in several cellular processes that regulate RNA secondary structure, including the initiation of translation and nuclear/mitochondrial splicing, as well as ribosome and spliceosome assembly, and is thought to play a regulatory role in the reproductive system (28). Studies have shown that DDX4 may be involved in the occurrence of ovarian cell carcinoma, gastric cancer, lung cancer, and other tumors by down-regulating 14-3-3σ activity, and the occurrence of tumors may be related to its ability to induce cell proliferation (29,30).
Our study found that SLC16A3 and DDX4 were differentially and highly expressed in CCA, and were independent prognostic factors related to survival. According to our analysis of SLC16A3 and DDX4 in widespread cancer, we found that SLC16A3 and DDX4 were highly expressed in a variety of tumor tissues, which was consistent with the above study results. In addition, studies have shown that miR-4524a, which is lowly expressed in colorectal cancer, can mediate tumorigenesis by regulating the lactate dehydrogenase A (LDHA) (31), and SLC16A3 can also promote tumor progression by regulating LDHA levels in tumor tissues (32). However, our study found that miR-4524a was down-regulated and SLC16A3 was highly expressed in CCA, and a regulatory axis including miR-4524a and SLC16A3 was obtained. Therefore, we hypothesized that in CCA, hsa_circ_0002073 may be involved in the tumor-promoting process of SLC16A3 and DDX4 as a sponge molecule of hsa-miR-4524a-3p. Thus, these molecules may become new diagnostic markers and therapeutic targets for CCA.
Up to now, the mechanism of hsa-miR-4524a-3p targeted regulation of SLC16A3, SLC35E4, and DDX4 has not been reported in CCA. Our study provides a direction for the screening of CCA prognostic markers in the future.
In addition, we also found that SLC16A3, a member of the MCT family, mediates the protein-conjugated transport of low-molecular-weight monocarboxylic acids such as pyruvate and lactic acid, whose changes have been associated with cancer (33). The high expression of SLC16A3 can increase the level of lactate in tumor tissues, resulting in the decrease of pH, which has a direct impact on the increase in myeloid-deriver suppressor cells (MDSCs) in tumor tissues in tumor tissues and the degree of low PH in the TME. MDSCs and lactic acid esters have been shown to inhibit the function of NK cells and T lymphocytes, thereby promoting the progress of the tumor (32). Bovenzi et al. (34) also showed that the high expression of SLC16A3 in glycolysis-dependent tissues, which are functional biomarkers for metabolic compartmentalization in cancer, is associated with tumor invasiveness and prognosis. Therefore, we hypothesized that the effect of SLC16A3 on the prognosis of CCA patients may be realized by regulating the lactate level and changing the TME.
The TME refers to the environment in which tumor cells grow. Studies have shown that the TME not only plays a key role in the initiation, progression, and metastasis of tumors, but also has a profound impact on the therapeutic effect (35). Innate and acquired immune cells of the immune system into the TME and participate in the regulation of tumor progression (36,37), whereas immunotherapy eliminate the effect of tumor cells by enhancing the natural defense, and immune cells is the basis of immune therapy. Therefore, understanding the immune cells infiltrating in the TME is the important precondition for improving the effect of anti-tumor therapy There are many technical means to study the composition of tumor immune microenvironment, and the method based on sequencing technology and bioinformatics analysis is the more common method at present. Through transcriptome sequencing, we can obtain the expression data of different genes in tumor samples, and obtain the expression levels of various cells in tumor immune microenvironment through the corresponding bioinformatics software, so as to classify the TME and identify the infiltrating immune cells. Known immunotherapies include: oncolytic viruses that use genetically modified viruses to infect tumor cells and thus stimulate a pro-inflammatory environment to enhance systemic antitumor immunity (38); cancer vaccines, using tumor-specific antigens to trigger T cell-mediated antitumor immune responses (39); cytokine therapy, which can rapidly propagate immune signals, resulting in immune-response against target antigens (40). Adoptive cell transfer against tumor cells by using autoimmune cells to expand in vitro and re-transfer in vivo (41); ICP inhibitors that reactivate anti-tumor immune responses by blocking coinhibitory signaling (42). Among them, ICP inhibitor therapy has become one of the most important immunotherapies. In order to further understand the TME of CCA and its impact on patient survival time, we analyzed it based on TCGA database, and found that patients with a high proportion of immune cells had a higher 5-year survival rate. Therefore, we conclude that the infiltration of immune cells may be related to the prognosis of patients with CCA.
Next, we evaluated 22 TICs in CCA using CIBERSORT software, and analyzed the correlation between key target genes SLC16A3, SLC35E4, DDX4 and TICs in the ceRNA prognostic subnetwork using TIMER2.0, the results showed that the target genes in the CCA ceRNA prognostic subnetwork were correlated with the distribution of different TICs. SLC16A3 was correlated with the infiltration degree of resting myeloid DCs (MDCs), neutrophils, and CD4+ T cells, while SLC35E4 was correlated with the infiltration degree of macrophages. Although there were not many immune cells associated with target genes in our ceRNA prognostic subnetwork, these immune cells all play an important role in the development of tumors. From the previous analysis, we know that SLC16A3 can promote the aggregation of MDSCs and promote tumor development by changing the TME. Meanwhile, DCs, macrophages, and granulocytes are derived from MDSCs; that is, MDSCs are their precursor.
DCs are antigen presenting cells, which are divided into MDCs, also known as DC1, and lymphoid dendritic cells (LDC) or plasmacytoid DCs (piX), such as DC2 (43,44). As antigen-presenting cells, DCs play a crucial role in linking innate and acquired immune responses (45), and activate CD8+ T cells by cross-presentation (46). Studies have shown that the TME can achieve tumor immune escape by regulating DC function through tumor or tumor-related cytokines, and this mechanism may be related to functional defects of DC-mediated immune suppression (47,48). Melief et al. (49) showed that the TME tends to cause DC dysfunction, leading to failure of cross initiation, and thus, its mediated cross presentation of tumor antigens in tumor hosts usually induces T cell tolerance rather than immunity.
Similarly, relevant studies have shown that TAMs are key regulators of therapeutic response to the TME. In the case of TAMs, M1 macrophages are believed to have anti-tumor effects, while M2 macrophages are believed to promote the occurrence of tumors. M1-type and M2-type macrophages are interchangeable, and the TME plays a major role in the functional regulation of TAMs (50,51).
Neutrophils, another form of immune cell expression, have also been found to infiltrate in many types of tumors. Neutrophil particles contain a variety of antibacterial and cytotoxic substances that can destroy malignant cells and are effective antitumor effector cells, while the cytokines and chemokines secreted by neutrophils can also aggregate other cells with antitumor activity (52). However, several studies have shown that tumor-associated neutrophils may promote tumor progression, and N2-polarized neutrophils are morphologically similar to granulocytes or polymorphonuclear myeloid suppressor cells, which may play a tumor suppressive role (53).
Generally, in addition to cancer cells, stromal cells and infiltrating immune cells are also important components of the TME, among which the infiltrating immune cells are considered to be effective predictors of patient prognosis and may be effective targets for drugs (54). In summary, we can understand that the immune cells associated with target genes in the ceRNA prognostic subnetwork all play different roles in the occurrence and development of tumors, and their tumorigenesis is related to the TME. MiRNA-4524a can inhibit tumor development by targeting LDHA to alter glucose metabolism in the TME (31). Also, SLC16A3 can mediate changes in the TME by regulating lactate levels and promoting tumor development (32). Meanwhile, related immune cells have different effects on tumor development in different TMEs. Therefore, we speculated that the poor survival prognosis of CCA patients might be the result of the joint action of target genes in the ceRNA network.
It is well known that the standard advanced first-line treatment for CCA is gemcitabine combined with cisplatin, while a standard second-line treatment is currently unavailable (6). In recent years, with the development of bioinformatics, tumor immunotherapy has gradually attracted people’s attention, and was ranked first among the top 10 scientific breakthroughs in 2013 by Science. Targeted drugs targeting several ICPs, PD-1 and PD-L1, have achieved good results in anti-tumor therapy of a variety of solid tumors (55,56). PD-1 is one of the checkpoints that regulate the immune response, and its expression can promote the apoptosis of antigen-specific T cells, while reducing the apoptosis of heterogeneous T cells. PD-L1 is the ligand of PD-1. The binding of PD-L1, which is highly expressed on the tumor, to PD-1 in T cells induces the apoptosis of T cells, and thus, tumor cells can escape from the anti-tumor immune response and undergo immune escape. Therefore, blocking PD-1/PD-L1 is an important strategy for cancer immunotherapy. Studies have shown that 9.1–72.2% of CCA patients exhibit a high expression of PD-L1 (57-59). Geynisman (60) observed the high mutation rate of programmed death protein PD-1 and its programmed death ligand PD-L1 in CCA tissues, proving that PD-L1 plays an important role in the pathogenesis of CCA.
In 2020, a report on PD-1 treatment for melanoma showed that patients with low expression of SLC16A3 had better responses to pembrolizumab than patients with high expression of SLC16A3 (61). Pembrolizumab is a mAb that binds to the PD-1 receptor, and exerts anti-tumor effects (62-65). It is Food and Drug Administration (FDA) approved for the treatment of advanced melanoma and non-small cell lung cancer (55,66,67). However, evaluation for its use in the treatment of CCA is lacking. In recent years, a large number of clinical trials have been conducted for immunotargeted therapy of CCA (68). In 2018, Mou et al. (69) reported a case of complete response after treatment with pembrolizumab combined with chemotherapy in a CCA patient with tumor mutation load and a high expression of PD-L1, suggesting that pembrolizumab may have certain clinical therapeutic effects for CCA patients with high expression of PD-L1; however, the mechanism is unclear. Although this is inconsistent with the results of anti-PD-1 in the treatment of melanoma, it provides a certain reference value for the targeted therapy of CCA.
Existing clinical trials and research findings suggest that targeted ICP therapy may be a potential therapy for CCA (70). Based on the above analysis results, we further verified that the protein levels of SLC16A3 in CCA tissues were higher than that in normal tissues through the HPA database. Therefore, we conclude that SLC16A3 is an independent factor of CCA. Also, the poor prognosis of some CCA patients with high SLC16A3 expression may be related to PD-L1-mediated tumor immune escape, and these patients may be a potential population that could benefit from treatment with ICP inhibitors such as pembrolizumab.
In this study, a ceRNA network was constructed based on bioinformatics analysis, and a ceRNA prognostic subnetwork was constructed based on independent prognostic factors. Moreover, a correlation analysis of the target genes of the subnetwork species among immune infiltrating cells and ICPs was also conducted. The HPA database further confirmed that the protein expression levels of SLC16A3 were higher in CCA tissues than in normal tissues, and the ONCOMINE online analysis verified the differential expression of SLC16A3 in various tumor tissues. Based on the above studies, we believe that SLC16A3, as a key factor in the ceRNA prognostic subnetwork, may become a new diagnostic marker for CCA and a key target for immunotherapy. In addition, other circRNAs and miRNAs in the ceRNA network are likely to play an important role in the occurrence and development of CCA, clinical prognosis, and the remodeling of the TME through the regulation of the above three target genes, which is worthy of further study.
However, there are several limitations to our study that should be noted. Firstly, the small sample size used for our analysis may lead to potential error or bias. Secondly, the regulatory mechanism of the ceRNA prognostic subnetwork in this study lacks biological verification. In addition, transcriptome analysis cannot fully explain the effects of immune composition or immune status on tumor cells, but can only reflect the overall levels of cells. In the future, we will verify the expression and regulatory relationship of each node molecule in the ceRNA network through tissue cell experiments.
Conclusions
In our study, a ceRNA prognostic subnetwork related to the survival of CCA patients was constructed based on the TCGA database, which plays an important role in the study of the pathogenesis of CCA and the screening of prognostic markers. At the same time, the target genes in the network were found to regulate the immune state of the tumor, which is expected to provide new insights and ideas for CCA immunotherapy.
Acknowledgments
Funding: This work was supported by grants from the Natural Science Foundation of Liaoning Province (2020-MS-067), the Key Projects of Liaoning Natural Science Foundation (20180530019), the Natural Fund Guidance Program of Liaoning Province (2019-ZD-1072), the Major Scientific and Technological Innovation Research and Development Plan (19-112-4-079), the China Medical University Youth Backbone Support Program (1210519002), the Shanxi Administration of Traditional Chinese Medicine (2019-GJ-JC005); and the Program of Science and Technology Commission of Shanghai Municipality (18411969200).
Footnote
Reporting Checklist: The authors have completed the REMARK reporting checklist. Available at https://dx.doi.org/10.21037/jgo-21-619
Conflicts of Interest: All authors have completed the ICMJE uniform disclosure form (available at https://dx.doi.org/10.21037/jgo-21-619). The authors have no conflicts of interest to declare.
Ethical Statement: The authors are accountable for all aspects of the work in ensuring that questions related to the accuracy or integrity of any part of the work are appropriately investigated and resolved. All procedures performed in this study involving human participants were in accordance with the Declaration of Helsinki (as revised in 2013). Institutional ethical approval and informed consent were waived.
Open Access Statement: This is an Open Access article distributed in accordance with the Creative Commons Attribution-NonCommercial-NoDerivs 4.0 International License (CC BY-NC-ND 4.0), which permits the non-commercial replication and distribution of the article with the strict proviso that no changes or edits are made and the original work is properly cited (including links to both the formal publication through the relevant DOI and the license). See: https://creativecommons.org/licenses/by-nc-nd/4.0/.
References
- Cai Y, Cheng N, Ye H, et al. The current management of cholangiocarcinoma: A comparison of current guidelines. Biosci Trends 2016;10:92-102. [Crossref] [PubMed]
- Blechacz B, Komuta M, Roskams T, et al. Clinical diagnosis and staging of cholangiocarcinoma. Nat Rev Gastroenterol Hepatol 2011;8:512-22. [Crossref] [PubMed]
- Jarnagin WR, Fong Y, DeMatteo RP, et al. Staging, resectability, and outcome in 225 patients with hilar cholangiocarcinoma. Ann Surg 2001;234:507-17; discussion 517-9. [Crossref] [PubMed]
- Jödicke L, Zender L, Malek NP. Personalized treatment of cholangiocellular carcinoma (CCA). Internist (Berl) 2020;61:170-4. [PubMed]
- Razumilava N, Gores GJ. Cholangiocarcinoma. Lancet 2014;383:2168-79. [Crossref] [PubMed]
- Weigt J, Malfertheiner P. Cisplatin plus gemcitabine versus gemcitabine for biliary tract cancer. Expert Rev Gastroenterol Hepatol 2010;4:395-7. [Crossref] [PubMed]
- Hong TS, Wo JY, Yeap BY, et al. Multi-Institutional Phase II Study of High-Dose Hypofractionated Proton Beam Therapy in Patients With Localized, Unresectable Hepatocellular Carcinoma and Intrahepatic Cholangiocarcinoma. J Clin Oncol 2016;34:460-8. [Crossref] [PubMed]
- Garcia-Pardo M, Ortega L, Fernández-Aceñero MJ, et al. Molecular Profiling and Targeted Therapy in Cholangiocarcinoma: An Observational, Retrospective Multicenter Study. J Gastrointest Cancer 2021;52:814-8. [Crossref] [PubMed]
- Bang Y J, Ueno M, Malka D, et al. Pembrolizumab (pembro) for advanced biliary adenocarcinoma: Results from the KEYNOTE-(KN028) and KEYNOTE-158 (KN158) basket studies. J Clin Oncol 2019;37:abstr 4079.
- Salmena L, Poliseno L, Tay Y, et al. A ceRNA hypothesis: the Rosetta Stone of a hidden RNA language? Cell 2011;146:353-8. [Crossref] [PubMed]
- Wang J, Zhao X, Wang Y, et al. circRNA-002178 act as a ceRNA to promote PDL1/PD1 expression in lung adenocarcinoma. Cell Death Dis 2020;11:32. [Crossref] [PubMed]
- Li X, Yang L, Chen LL. The Biogenesis, Functions, and Challenges of Circular RNAs. Mol Cell 2018;71:428-42. [Crossref] [PubMed]
- Piwecka M, Glažar P, Hernandez-Miranda LR, et al. Loss of a mammalian circular RNA locus causes miRNA deregulation and affects brain function. Science 2017;357:eaam8526 [Crossref] [PubMed]
- Jin MZ, Jin WL. The updated landscape of tumor microenvironment and drug repurposing. Signal Transduct Target Ther 2020;5:166. [Crossref] [PubMed]
- Høgdall D, Lewinska M, Andersen JB. Desmoplastic Tumor Microenvironment and Immunotherapy in Cholangiocarcinoma. Trends Cancer 2018;4:239-55. [Crossref] [PubMed]
- Kitano Y, Okabe H, Yamashita YI, et al. Tumour-infiltrating inflammatory and immune cells in patients with extrahepatic cholangiocarcinoma. Br J Cancer 2018;118:171-80. [Crossref] [PubMed]
- Chang Z, Huang R, Fu W, et al. The Construction and Analysis of ceRNA Network and Patterns of Immune Infiltration in Colon Adenocarcinoma Metastasis. Front Cell Dev Biol 2020;8:688. [Crossref] [PubMed]
- Huang R, Wu J, Zheng Z, et al. The Construction and Analysis of ceRNA Network and Patterns of Immune Infiltration in Mesothelioma With Bone Metastasis. Front Bioeng Biotechnol 2019;7:257. [Crossref] [PubMed]
- Blechacz B. Cholangiocarcinoma: Current Knowledge and New Developments. Gut Liver 2017;11:13-26. [Crossref] [PubMed]
- Song F, Hu B, Cheng JW, et al. Anlotinib suppresses tumor progression via blocking the VEGFR2/PI3K/AKT cascade in intrahepatic cholangiocarcinoma. Cell Death Dis 2020;11:573. [Crossref] [PubMed]
- DeOliveira ML, Cunningham SC, Cameron JL, et al. Cholangiocarcinoma: thirty-one-year experience with 564 patients at a single institution. Ann Surg 2007;245:755-62. [Crossref] [PubMed]
- Park J, Kim MH, Kim KP, et al. Natural History and Prognostic Factors of Advanced Cholangiocarcinoma without Surgery, Chemotherapy, or Radiotherapy: A Large-Scale Observational Study. Gut Liver 2009;3:298-305. [Crossref] [PubMed]
- Spolverato G, Bagante F, Weiss M, et al. Comparative performances of the 7th and the 8th editions of the American Joint Committee on Cancer staging systems for intrahepatic cholangiocarcinoma. J Surg Oncol 2017;115:696-703.
- Qi X, Zhang DH, Wu N, et al. ceRNA in cancer: possible functions and clinical implications. J Med Genet 2015;52:710-8. [Crossref] [PubMed]
- Cao YW, Liu Y, Dong Z, et al. Monocarboxylate transporters MCT1 and MCT4 are independent prognostic biomarkers for the survival of patients with clear cell renal cell carcinoma and those receiving therapy targeting angiogenesis. Urol Oncol 2018;36:311.e15-25. [Crossref] [PubMed]
- Yorita K, Ohno A, Nishida T, et al. Intratumoral reciprocal expression of monocarboxylate transporter 4 and glypican-3 in hepatocellular carcinomas. BMC Res Notes 2019;12:741. [Crossref] [PubMed]
- Liu Y, Sun X, Huo C, et al. Monocarboxylate Transporter 4 (MCT4) Overexpression Is Correlated with Poor Prognosis of Osteosarcoma. Med Sci Monit 2019;25:4278-84. [Crossref] [PubMed]
- Silvestris E, Cafforio P, Felici C, et al. Ddx4+ Oogonial Stem Cells in Postmenopausal Women's Ovaries: A Controversial, Undefined Role. Cells 2019;8:650. [Crossref] [PubMed]
- Poon J, Wessel GM, Yajima M. An unregulated regulator: Vasa expression in the development of somatic cells and in tumorigenesis. Dev Biol 2016;415:24-32. [Crossref] [PubMed]
- Schudrowitz N, Takagi S, Wessel GM, et al. Germline factor DDX4 functions in blood-derived cancer cell phenotypes. Cancer Sci 2017;108:1612-9. [Crossref] [PubMed]
- Wang J, Wang H, Liu A, et al. Lactate dehydrogenase A negatively regulated by miRNAs promotes aerobic glycolysis and is increased in colorectal cancer. Oncotarget 2015;6:19456-68. [Crossref] [PubMed]
- Husain Z, Seth P, Sukhatme VP. Tumor-derived lactate and myeloid-derived suppressor cells: Linking metabolism to cancer immunology. Oncoimmunology 2013;2:e26383 [Crossref] [PubMed]
- Walenta S, Voelxen NF, Mueller-Klieser W. Lactate-An Integrative Mirror of Cancer Metabolism. Recent Results Cancer Res 2016;207:23-37. [Crossref] [PubMed]
- Bovenzi CD, Hamilton J, Tassone P, et al. Prognostic Indications of Elevated MCT4 and CD147 across Cancer Types: A Meta-Analysis. Biomed Res Int 2015;2015:242437 [Crossref] [PubMed]
- Wu T, Dai Y. Tumor microenvironment and therapeutic response. Cancer Lett 2017;387:61-8. [Crossref] [PubMed]
- Grivennikov SI, Greten FR, Karin M. Immunity, inflammation, and cancer. Cell 2010;140:883-99. [Crossref] [PubMed]
- Seager RJ, Hajal C, Spill F, et al. Dynamic interplay between tumour, stroma and immune system can drive or prevent tumour progression. Converg Sci Phys Oncol 2017;3:034002 [Crossref] [PubMed]
- Sinkovics J, Horváth J. Oncolytic virotherapy. Orv Hetil 2008;149:1187-author reply 1187-8. [PubMed]
- van der Bruggen P, Traversari C, Chomez P, et al. A gene encoding an antigen recognized by cytolytic T lymphocytes on a human melanoma. Science 1991;254:1643-7. [Crossref] [PubMed]
- Sudan R. Cytokines in Cancer Immunotherapy. In: Singh S. editor. Systems and Synthetic Immunology. Singapore: Springer, 2020.
- Rosenberg SA, Restifo NP. Adoptive cell transfer as personalized immunotherapy for human cancer. Science 2015;348:62-8. [Crossref] [PubMed]
- Sharma P, Allison JP. Immune checkpoint targeting in cancer therapy: toward combination strategies with curative potential. Cell 2015;161:205-14. [Crossref] [PubMed]
- Murphy TL, Grajales-Reyes GE, Wu X, et al. Transcriptional Control of Dendritic Cell Development. Annu Rev Immunol 2016;34:93-119. [Crossref] [PubMed]
- Anderson DA 3rd, Murphy KM, Briseño CG. Development, Diversity, and Function of Dendritic Cells in Mouse and Human. Cold Spring Harb Perspect Biol 2018;10:a028613 [Crossref] [PubMed]
- Banchereau J, Steinman RM. Dendritic cells and the control of immunity. Nature 1998;392:245-52. [Crossref] [PubMed]
- Ferris ST, Durai V, Wu R, et al. cDC1 prime and are licensed by CD4+ T cells to induce anti-tumour immunity. Nature 2020;584:624-9. [Crossref] [PubMed]
- Veglia F, Gabrilovich DI. Dendritic cells in cancer: the role revisited. Curr Opin Immunol 2017;45:43-51. [Crossref] [PubMed]
- Tran Janco JM, Lamichhane P, Karyampudi L, et al. Tumor-infiltrating dendritic cells in cancer pathogenesis. J Immunol 2015;194:2985-91. [Crossref] [PubMed]
- Melief CJ. Cancer immunotherapy by dendritic cells. Immunity 2008;29:372-83. [Crossref] [PubMed]
- Duluc D, Corvaisier M, Blanchard S, et al. Interferon-gamma reverses the immunosuppressive and protumoral properties and prevents the generation of human tumor-associated macrophages. Int J Cancer 2009;125:367-73. [Crossref] [PubMed]
- Hagemann T, Lawrence T, McNeish I, et al. "Re-educating" tumor-associated macrophages by targeting NF-kappaB. J Exp Med 2008;205:1261-8. [Crossref] [PubMed]
- Ocana A, Nieto-Jiménez C, Pandiella A, et al. Neutrophils in cancer: prognostic role and therapeutic strategies. Mol Cancer 2017;16:137. [Crossref] [PubMed]
- Rakic A, Beaudry P, Mahoney DJ. The complex interplay between neutrophils and cancer. Cell Tissue Res 2018;371:517-29. [Crossref] [PubMed]
- Chen DS, Mellman I. Elements of cancer immunity and the cancer-immune set point. Nature 2017;541:321-30. [Crossref] [PubMed]
- Garon EB, Rizvi NA, Hui R, et al. Pembrolizumab for the treatment of non-small-cell lung cancer. N Engl J Med 2015;372:2018-28. [Crossref] [PubMed]
- Sul J, Blumenthal GM, Jiang X, et al. FDA Approval Summary: Pembrolizumab for the Treatment of Patients With Metastatic Non-Small Cell Lung Cancer Whose Tumors Express Programmed Death-Ligand 1. Oncologist 2016;21:643-50. [Crossref] [PubMed]
- Fontugne J, Augustin J, Pujals A, et al. PD-L1 expression in perihilar and intrahepatic cholangiocarcinoma. Oncotarget 2017;8:24644-51. [Crossref] [PubMed]
- Walter D, Herrmann E, Schnitzbauer AA, et al. PD-L1 expression in extrahepatic cholangiocarcinoma. Histopathology 2017;71:383-92. [Crossref] [PubMed]
- Ma K, Wei X, Dong D, et al. PD-L1 and PD-1 expression correlate with prognosis in extrahepatic cholangiocarcinoma. Oncol Lett 2017;14:250-6. [Crossref] [PubMed]
- Geynisman DM. E-mail anonymous: a physician's addiction. J Clin Oncol 2015;33:285-6. [Crossref] [PubMed]
- Li N, Kang Y, Wang L, et al. ALKBH5 regulates anti-PD-1 therapy response by modulating lactate and suppressive immune cell accumulation in tumor microenvironment. Proc Natl Acad Sci U S A 2020;117:20159-70. [Crossref] [PubMed]
- Topalian SL, Drake CG, Pardoll DM. Immune checkpoint blockade: a common denominator approach to cancer therapy. Cancer Cell 2015;27:450-61. [Crossref] [PubMed]
- Parsa AT, Waldron JS, Panner A, et al. Loss of tumor suppressor PTEN function increases B7-H1 expression and immunoresistance in glioma. Nat Med 2007;13:84-8. [Crossref] [PubMed]
- Kwok G, Yau TC, Chiu JW, et al. Pembrolizumab (Keytruda). Hum Vaccin Immunother 2016;12:2777-89. [Crossref] [PubMed]
- Reck M, Rodríguez-Abreu D, Robinson AG, et al. Pembrolizumab versus Chemotherapy for PD-L1-Positive Non-Small-Cell Lung Cancer. N Engl J Med 2016;375:1823-33. [Crossref] [PubMed]
- Robert C, Ribas A, Wolchok JD, et al. Anti-programmed-death-receptor-1 treatment with pembrolizumab in ipilimumab-refractory advanced melanoma: a randomised dose-comparison cohort of a phase 1 trial. Lancet 2014;384:1109-17. [Crossref] [PubMed]
- Herbst RS, Baas P, Kim DW, et al. Pembrolizumab versus docetaxel for previously treated, PD-L1-positive, advanced non-small-cell lung cancer (KEYNOTE-010): a randomised controlled trial. Lancet 2016;387:1540-50. [Crossref] [PubMed]
- Hezel AF, Deshpande V, Zhu AX. Genetics of biliary tract cancers and emerging targeted therapies. J Clin Oncol 2010;28:3531-40. [Crossref] [PubMed]
- Mou H, Yu L, Liao Q, et al. Successful response to the combination of immunotherapy and chemotherapy in cholangiocarcinoma with high tumour mutational burden and PD-L1 expression: a case report. BMC Cancer 2018;18:1105. [Crossref] [PubMed]
- Bang Y, Doi T, Braud F, et al. Safety and efficacy of pembrolizumab (MK-3475) in patients (pts) with advanced biliary tract cancer: Interim results of KEYNOTE-028. Eur J Cancer 2015;51:S112. [Crossref]
(English Language Editor: A. Kassem)