Prediction and prognostic significance of ALOX12B and PACSIN1 expression in gastric cancer by genome-wide RNA expression and methylation analysis
Introduction
Globally, gastric cancer (GC), also known as stomach cancer, is one of the most common malignancies and the third-leading contributor to cancer deaths, accounting for 8.2% of the total mortality caused by tumors (1). The past several decades have witnessed a substantial decline in the incidence and mortality of GC (2), and there have been breakthroughs in the treatment of early-stage GC (3). However, GC has a high rate of recurrence, metastasis, and mortality. Between 70% and 80% of GC recurrences occur within the first 2 years after local therapy, and almost all (~90%) recurrences occur by 5 years (4-6). It tends to metastasize into lymph nodes, adjacent tissues, and organs (7). Although more and more studies focus on gastric cancer, there are still limited studies between metastasis and non-metastasis groups by integrated multi-layer omics method. As we all knows, gastric cancer is hard to detect micro-metastasis by conventional method which was crucial for following treatment and prognosis. Once the tumor cell metastasis to distance sites, the stage will be defined as advanced. Patients with advanced GC often have poor prognoses; the 5-year survival rate of under 10% is extremely low (8). Tumor metastasis is an important cause of mortality and represents a major barrier to improving the survival of patients with GC (9). It is challenging to overcome metastasis in GC with the current medical methods. Therefore, novel sensitive biomarkers that detect and predict metastasis in GC are urgently needed. However, the underlying mechanism of distant metastasis remains unclear in GC. It is a key imperative to investigate the molecular mechanisms that are associated with aggressive GC behavior, and reveal the potential target genes for early intervention to elevate the clinical treatment efficiency of GC. Dysregulated expression of RNA plays a role in tumor metastasis (10), and DNA methylation is also an important regulator of gene expression via epigenetic mechanisms (11), which can inhibit the binding of transcription factors or the recruitment of repression proteins.
DNA methylation is an important epigenetic modification which influence downstream gene silencing, genetic imprinting, X-chromosome inactivation (XCI), genome stability, and cell fate determination (12). DNA methyltransferase 3 alpha (DNMT3A) and beta (DNMT3B) had been demonstrated play a vital role in tumorigenesis mainly by changing methylation stage of specific genes (13). Aberrant methylated regions often lead to silencing tumor suppressor genes or activating the oncogenes. Epigenetic alterations played a crucial role in regulatory of PI3K/AKT/mTOR signaling pathway which were important for gastric cancer development, prognosis, and metastasis (14). Another study also showed ALKBH5 promotes invasion and metastasis of gastric cancer by decreasing methylation of the lncRNA NEAT1 (15).
Therefore, combining RNA dysregulation and DNA methylation can provide a more comprehensive view in the search for biomarkers of GC metastasis. In this study, we aimed to mining metastasis markers for gastric cancer.
We present the following article in accordance with the REMARK reporting checklist (available at https://dx.doi.org/10.21037/jgo-21-508).
Methods
Data sources
The Cancer Genome Atlas (TCGA) stomach adenocarcinoma (STAD) RNAseq reads count data from 329 samples and DNA methylation data (Illumina HumanMethylation450 k Array, Illumina Inc., San Diego, USA) from 353 samples were obtained from The Genomic Data Commons (GDC) Data Portal (https://portal.gdc.cancer.gov) (16). Clinical information, including tumor stage and sample ID were also downloaded. The study was conducted in accordance with the Declaration of Helsinki (as revised in 2013).
Preprocessing and analysis of RNA data
Samples were divided into M0 and M1 groups according to clinical stage information. The RNA reads count data were preprocessed via DESeq2 (17), log2-fold change >1, P value <0.05 was selected as threshold. All differential expressed genes were obtained. A volcano plot of differential expressed genes was generated by ggplot2 (R package, https://ggplot2.tidyverse.org). All differential expressed genes for GSE11617 were analyzed by GEO2R (https://www.ncbi.nlm.nih.gov/geo/geo2r/).
Functional enrichment analyses
Gene Ontology (GO) and Kyoto Encyclopedia of Genes and Genomes (KEGG) enrichment analysis were performed by DAVID online tools (https://david.ncifcrf.gov) (18) for differentially expressed genes. The R package ggplot2 was utilized to visualize the gene number involved in GO term and KEGG pathways.
Preprocessing and analysis of DNA methylation data
The DNA methylation data were preprocessed and normalized using the minfi (R package, https://bioconductor.org/packages/release/bioc/html/minfi.html) (19), P value <0.05 was selected as threshold. All differentially methylated probes were obtained.
Overlap statics between RNA and methylation, copy number variation (CNV) analysis
Genes up-regulated at RNA levels and low methylated associated probes were extracted for further analysis. CNV analyses were performed by cBioPortal online tools (https://www.cbioportal.org) (20), TCGA PanCancer Atlas Studies were selected as the reference database, which enrolls 32 studies and 10,967 samples.
Gene set enrichment analysis (GSEA)
Fragments per kilobase of exon model per million reads mapped (FPKM) data of STAD was downloaded from GDC Data Portal (https://portal.gdc.cancer.gov) (16). After annotation, the expression matrix was ordered by selected gene FPKM value from low to high, all participants were divided into high and low groups by mean expression of ALOX12B or Protein Kinase C and Casein Kinase Substrate in Neurons 1 (PACSIN1). Hallmark gene sets (H), curated gene sets (C2), computational gene sets (C4), GO gene sets (C5), and oncogenic gene sets (C6) were selected as the reference molecular signature database (21) to perform GSEA. The GSEA analysis of selected genes were performed by GSEA official desktop tools (22).
Survival analysis
Survival plots of selected genes were generated by Kaplan-Meier Plotter tools (https://kmplot.com) (23); the GC database was selected, and overall survival (OS), progression-free survival (PFS), and post-progression survival (PPS) plots were obtained. The level of significance was set at P<0.05.
Statistical analysis
This study used R software v4.0.3 for statistical analysis. DESeq statistical test was used for comparison of different expression genes between metastasis and non-metastasis samples. Kaplan-Meier curves, log-rank test are used in survival analysis. ALOX12B and PACSIN1 expression comparison in GSE11617 using Wilcoxon test. For all statistical methods, the P<0.05 was considered to indicate statistical significance.
Results
Identification of different genes between metastasis and non-metastasis samples
The sample stages landscape information was analyzed. Samples with metastasis information were divided into 2 groups (M0: non-metastasis, M1: distant metastasis) by labels from clinical data. Totally, 391 samples were enrolled in M0 group, and 30 samples enrolled in M1 group (Figure 1 and https://cdn.amegroups.cn/static/public/jgo-21-508-1.xlsx). After preprocessing of the data and annotation, 34,598 genes were retained for further analysis.
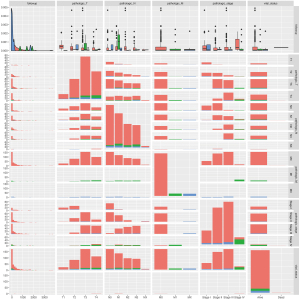
The genes between the STAD metastasis samples and non-metastasis control samples were then determined using DESeq2 package in R. In total, 229 genes were identified between metastasis and control tissue samples according to the following parameters: log2 fold change >1, P<0.05. Of the 229 genes, 29 were upregulated genes and 200 were downregulated genes (Figure 2A and https://cdn.amegroups.cn/static/public/jgo-21-508-2.xlsx).
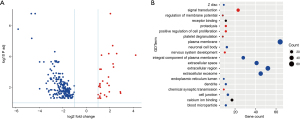
Through GO functional analysis, differentially expressed genes were found mainly involving in cell extracellular GO terms (Figure 2B), such as: plasma membrane, extracellular space, extracellular region, extracellular exosome, and so on.
Identification of methylation probes between metastasis and non-metastasis samples
The DNA methylation data of 485,577 loci were downloaded from the TCGA database. After preprocessing of the data and quality control, 467,971 probes were retained for further analysis. After normalization of expression value, the methylation level of all samples was evaluated, and a relatively low methylation level was observed in all groups (Figure 3A). The different methylation probes (DMPs) between the metastasis samples and control tissue samples were then determined using a minfi package by default parameter. In total, 13,608 DMPs were identified. Among the 13,608 DMPs, 5,046 were hypermethylated probes and 8,563 were hypomethylated probes.
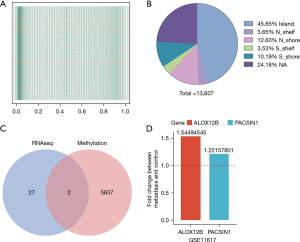
Genomic characteristics of DMPs between metastasis and non-metastasis tissue samples
A total of 13,608 DMPs were mapped onto genomic features based on the annotations provided by Illumina under GPL18809. Genomic distribution of 13,608 DMPs across different genomic regions was investigated and it was found that the DMPs were not randomly distributed across the genome. The 13,608 DMPs were located in Island (6,239, accounting for 45.8% of total DMPs), N_shelf (497, accounting for 3.68%), N_shore (1,714, accounting for 12.6%), S_shelf (480, accounting for 3.52%), S_shore (1,387, accounting for 10.2%), NA (3,290, accounting for 24.2%). The majority of the 13,608 DMPs were found locating in the Island (Figure 3B and https://cdn.amegroups.cn/static/public/jgo-21-508-3.xlsx).
RNA expression, DNA methylation and CNV
Genes that were up-regulated in RNA analysis and with low methylation were extracted, and 2 genes (ALOX12B and PACSIN1) were obtained under this criterion (Figure 3C). In order to validate the similar up-regulation pattern, another dataset from GEO database (GSE11617) was analysis, results also showed an up-regulated trend (Figure 3D). The CNV of ALOX12B and PACSIN1 was investigated, and low CNV levels in both ALOX12B (Figure 4A) and PACSIN1 (Figure 4B) (ALOX12B: 0.68% deletion, PACSIN1: 0.68% amplification and 0.23% deletion) were found, indicating that ALOX12B and PACSIN1 up-regulation may not associate with copy number alteration.
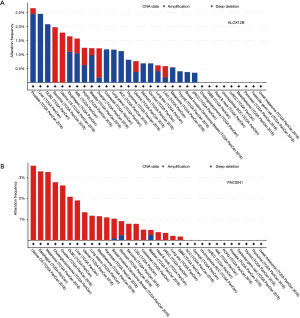
GSEA assay of ALOX12B and PACSIN1
To further investigate the potential functions of ALOX12B and PACSIN1, GSEA was performed on the TCGA STAD RNAseq data. Genes in high expression groups of ALOX12B were enriched in “ATP dependent microtubule motor activity” and “Mechanoreceptor differentiation” pathways (Figure 5A). Meanwhile, genes in high expression groups of PACSIN1 were enriched in AKT, Cyclin D1, KRAS, PTEN, and VEGF associated pathways (Figure 5B). These results implied that ALOX12B and PACSIN1 involved in typical cancer pathways and tumor invasion process.
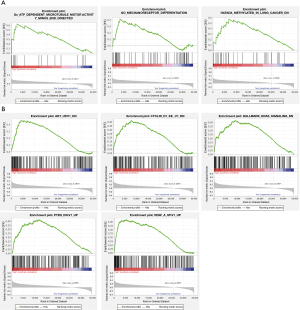
The ALOX12B and PACSIN1 genes predict the survival level in STAD
In ALOX12B and PACSIN1 survival analysis, the information of 876 and 631 patients were enrolled, respectively. Kaplan-Meier survival analysis revealed that the high expression of ALOX12B and PACSIN1 were associated with significantly poor patient survival in OS, PFS, and PPS (Figure 6; all subtypes).
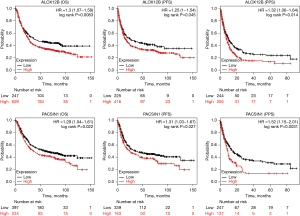
Discussion
GC is a very common gastrointestinal disease, which has been regarded as the most common risk factor for eastern people. It is also well accepted that the possibility of metastasis is crucial during clinical treatment (8,24). Therefore, investigating the metastasis biomarkers of GC will provide comprehensive information for effective therapeutic indicators.
In recent years, several studies have investigated the functions and clinical implications of Epstein-Barr virus (EBV) infection (25-27) and molecular genotype in GC (2,28,29). However, the molecular factor of metastasis on GC has not been well addressed. In this study, we showed that both ALOX12B and PACSIN1 RNA high expression were negative prognostic factors in patients with STAD. In our screening results for all tumor samples, we found the general methylation level was low, which may indicate relatively high expression for all genes in GC. However, among all genes, we found only 29 genes upregulated in comparison with the non-metastasis group, which may indicate these 29 genes play crucial role in metastasis progress.
Previous studies have shown diseases associated with ALOX12B include collodion baby (30,31) with autosomal recessive congenital ichthyosis (32). Low expression levels of ALOX12B were found to significantly reduce both cell proliferation and clone formation ability, and block cell cycle at G1 phase in cervical cancer. Tumor growth was also suppressed in vivo in a xenograft tumor model (33). Studies associating PACSIN1 have revealed that it works as a critical regulator of synaptic inhibition (30), regulator for toll-like receptor (TLR)7/9 interferon response (34), for axonal elongation and branching (35), and α-amino-3-hydroxy-5-methyl-4-isoxazolepropionic acid receptor (AMPA) receptor trafficking (36). PACSIN1 had been detected low expression levels in grade IV, IDH1 wild-type and 1p/19q non-codel group gliomas, its expression levels were positively correlated with OS in all gliomas and negatively correlated with the malignant degree of gliomas. These results indicated that PACSIN1 would play an essential role in the occurrence and development of gliomas (37). However, the role of ALOX12B and PACSIN1 in cancer metastasis had not been explored, and we found these 2 genes have potential to be the metastasis biomarker in GC.
High-throughput data mining can extract the relevant biological information (38,39), and integrated analysis of multiple omics has become a new trend of disease research, especially in precision medicine (40,41). Cancer patients will benefit from biomarkers in personalized medicine, and they will enable doctors to improve cancer diagnosis, treatment, and prevention (42,43).
As we know, cancer epigenome is characterized by global DNA hypomethylation and CpG island promoter hypermethylation. Previous studies demonstrated that abnormal methylation changes occurred at the CGI shores, which were important in cancers (44). Besides, methylation alterations involved in the earliest stage of tumorigenesis (45). Therefore, comprehensively investigation of methylation between subtypes of cancers is helpful to uncover the role of methylation in cancer initiation and development. Such study identified PCR2 which responsible for H3K27 trimethylation as a promising therapeutic target in nasopharyngeal carcinoma (46).
In conclusion, our study generated a comprehensive view of RNAseq and methylation co-analysis in STAD associated with metastasis status. We identified 2 candidate metastasis biomarkers for STAD associating with prognosis. The proposed potential predicted biomarker could provide information for further effective therapeutics.
Acknowledgments
Funding: None.
Footnote
Reporting Checklist: The authors have completed the REMARK reporting checklist. Available at https://dx.doi.org/10.21037/jgo-21-508
Conflicts of Interest: All authors have completed the ICMJE uniform disclosure form (available at https://dx.doi.org/10.21037/jgo-21-508). The authors have no conflicts of interest to declare.
Ethical Statement: The authors are accountable for all aspects of the work in ensuring that questions related to the accuracy or integrity of any part of the work are appropriately investigated and resolved. The study was conducted in accordance with the Declaration of Helsinki (as revised in 2013). Institutional ethical approval and informed consent were waived.
Open Access Statement: This is an Open Access article distributed in accordance with the Creative Commons Attribution-NonCommercial-NoDerivs 4.0 International License (CC BY-NC-ND 4.0), which permits the non-commercial replication and distribution of the article with the strict proviso that no changes or edits are made and the original work is properly cited (including links to both the formal publication through the relevant DOI and the license). See: https://creativecommons.org/licenses/by-nc-nd/4.0/.
References
- Bray F, Ferlay J, Soerjomataram I, et al. Global cancer statistics 2018: GLOBOCAN estimates of incidence and mortality worldwide for 36 cancers in 185 countries. CA Cancer J Clin 2018;68:394-424. [Crossref] [PubMed]
- Sitarz R, Skierucha M, Mielko J, et al. Gastric cancer: epidemiology, prevention, classification, and treatment. Cancer Manag Res 2018;10:239-48. [Crossref] [PubMed]
- Cai Z, Yin Y, Shen C, et al. Comparative effectiveness of preoperative, postoperative and perioperative treatments for resectable gastric cancer: A network meta-analysis of the literature from the past 20 years. Surg Oncol 2018;27:563-74. [Crossref] [PubMed]
- Youn HG, An JY, Choi MG, et al. Recurrence after curative resection of early gastric cancer. Ann Surg Oncol 2010;17:448-54. [Crossref] [PubMed]
- Song J, Lee HJ, Cho GS, et al. Recurrence following laparoscopy-assisted gastrectomy for gastric cancer: a multicenter retrospective analysis of 1,417 patients. Ann Surg Oncol 2010;17:1777-86. [Crossref] [PubMed]
- D'Angelica M, Gonen M, Brennan MF, et al. Patterns of initial recurrence in completely resected gastric adenocarcinoma. Ann Surg 2004;240:808-16. [Crossref] [PubMed]
- Valastyan S, Weinberg RA. Tumor metastasis: molecular insights and evolving paradigms. Cell 2011;147:275-92. [Crossref] [PubMed]
- Orditura M, Galizia G, Sforza V, et al. Treatment of gastric cancer. World J Gastroenterol 2014;20:1635-49. [Crossref] [PubMed]
- Tan HL, Chia CS, Tan GHC, et al. Metastatic gastric cancer: Does the site of metastasis make a difference? Asia Pac J Clin Oncol 2019;15:10-7. [Crossref] [PubMed]
- Luo X, Qiu Y, Jiang Y, et al. Long non-coding RNA implicated in the invasion and metastasis of head and neck cancer: possible function and mechanisms. Mol Cancer 2018;17:14. [Crossref] [PubMed]
- Han TS, Ban HS, Hur K, et al. The Epigenetic Regulation of HCC Metastasis. Int J Mol Sci 2018;19:3978. [Crossref] [PubMed]
- Bird A. DNA methylation patterns and epigenetic memory. Genes Dev 2002;16:6-21. [Crossref] [PubMed]
- Cheng Y, Yan Z, Liu Y, et al. Analysis of DNA methylation patterns associated with the gastric cancer genome. Oncol Lett 2014;7:1021-6. [Crossref] [PubMed]
- Fattahi S, Amjadi-Moheb F, Tabaripour R, et al. PI3K/AKT/mTOR signaling in gastric cancer: Epigenetics and beyond. Life Sci 2020;262:118513 [Crossref] [PubMed]
- Zhang J, Guo S, Piao HY, et al. ALKBH5 promotes invasion and metastasis of gastric cancer by decreasing methylation of the lncRNA NEAT1. J Physiol Biochem 2019;75:379-89. [Crossref] [PubMed]
- Grossman RL, Heath AP, Ferretti V, et al. Toward a Shared Vision for Cancer Genomic Data. N Engl J Med 2016;375:1109-12. [Crossref] [PubMed]
- Love MI, Huber W, Anders S. Moderated estimation of fold change and dispersion for RNA-seq data with DESeq2. Genome Biol 2014;15:550. [Crossref] [PubMed]
- Huang da W. Sherman BT, Lempicki RA. Systematic and integrative analysis of large gene lists using DAVID bioinformatics resources. Nat Protoc 2009;4:44-57. [Crossref] [PubMed]
- Aryee MJ, Jaffe AE, Corrada-Bravo H, et al. Minfi: a flexible and comprehensive Bioconductor package for the analysis of Infinium DNA methylation microarrays. Bioinformatics 2014;30:1363-9. [Crossref] [PubMed]
- Cerami E, Gao J, Dogrusoz U, et al. The cBio cancer genomics portal: an open platform for exploring multidimensional cancer genomics data. Cancer Discov 2012;2:401-4. [Crossref] [PubMed]
- Liberzon A, Subramanian A, Pinchback R, et al. Molecular signatures database (MSigDB) 3.0. Bioinformatics 2011;27:1739-40. [Crossref] [PubMed]
- Subramanian A, Tamayo P, Mootha VK, et al. Gene set enrichment analysis: a knowledge-based approach for interpreting genome-wide expression profiles. Proc Natl Acad Sci U S A 2005;102:15545-50. [Crossref] [PubMed]
- Györffy B, Lanczky A, Eklund AC, et al. An online survival analysis tool to rapidly assess the effect of 22,277 genes on breast cancer prognosis using microarray data of 1,809 patients. Breast Cancer Res Treat 2010;123:725-31. [Crossref] [PubMed]
- Ghajar CM. Metastasis prevention by targeting the dormant niche. Nat Rev Cancer 2015;15:238-47. [Crossref] [PubMed]
- Naseem M, Barzi A, Brezden-Masley C, et al. Outlooks on Epstein-Barr virus associated gastric cancer. Cancer Treat Rev 2018;66:15-22. [Crossref] [PubMed]
- Morales-Sanchez A, Fuentes-Panana EM. Epstein-Barr Virus-associated Gastric Cancer and Potential Mechanisms of Oncogenesis. Curr Cancer Drug Targets 2017;17:534-54. [Crossref] [PubMed]
- Shinozaki-Ushiku A, Kunita A, Fukayama M. Update on Epstein-Barr virus and gastric cancer Int J Oncol 2015;46:1421-34. (review). [Crossref] [PubMed]
- Sanjeevaiah A, Cheedella N, Hester C, et al. Gastric Cancer: Recent Molecular Classification Advances, Racial Disparity, and Management Implications. J Oncol Pract 2018;14:217-24. [Crossref] [PubMed]
- Röcken C. Molecular classification of gastric cancer. Expert Rev Mol Diagn 2017;17:293-301. [Crossref] [PubMed]
- Santesteban Muruzábal R, Larumbe Irurzun A, Yanguas Bayona I, et al. Self-healing Collodion Baby: A New Mutation in the ALOX12B Gene. Actas Dermosifiliogr 2016;107:433-5. [PubMed]
- Bland PJ, Chronnell C, Plagnol V, et al. A severe collodion phenotype in the newborn period associated with a homozygous missense mutation in ALOX12B. Br J Dermatol 2015;173:285-7. [Crossref] [PubMed]
- Lolas IB, Sommerlund M, Okkels H, et al. A novel deletion mutation in the ALOX12B gene in a Kurdish family with autosomal recessive congenital ichthyosis. J Eur Acad Dermatol Venereol 2016;30:e144-5. [Crossref] [PubMed]
- Jiang T, Zhou B, Li YM, et al. ALOX12B promotes carcinogenesis in cervical cancer by regulating the PI3K/ERK1 signaling pathway. Oncol Lett 2020;20:1360-8. [Crossref] [PubMed]
- Esashi E, Bao M, Wang YH, et al. PACSIN1 regulates the TLR7/9-mediated type I interferon response in plasmacytoid dendritic cells. Eur J Immunol 2012;42:573-9. [Crossref] [PubMed]
- Liu Y, Lv K, Li Z, et al. PACSIN1, a Tau-interacting protein, regulates axonal elongation and branching by facilitating microtubule instability. J Biol Chem 2012;287:39911-24. [Crossref] [PubMed]
- Widagdo J, Fang H, Jang SE, et al. PACSIN1 regulates the dynamics of AMPA receptor trafficking. Sci Rep 2016;6:31070. [Crossref] [PubMed]
- Zimu Z, Jia Z, Xian F, et al. Decreased Expression of PACSIN1 in Brain Glioma Samples Predicts Poor Prognosis. Front Mol Biosci 2021;8:696072 [Crossref] [PubMed]
- Chen B, Chen W, Jin J, et al. Data Mining of Prognostic Microenvironment-Related Genes in Clear Cell Renal Cell Carcinoma: A Study with TCGA Database. Dis Markers 2019;2019:8901649 [Crossref] [PubMed]
- Zhu XF, Zhu BS, Wu FM, et al. DNA methylation biomarkers for the occurrence of lung adenocarcinoma from TCGA data mining. J Cell Physiol 2018;233:6777-84. [Crossref] [PubMed]
- Chen Y, Wang X, Wang G, et al. Integrating multiple omics data for the discovery of potential Beclin-1 interactions in breast cancer. Mol Biosyst 2017;13:991-9. [Crossref] [PubMed]
- Roy Sarkar T, Maity AK, Niu Y, et al. Multiple Omics Data Integration to Identify Long Noncoding RNA Responsible for Breast Cancer-Related Mortality. Cancer Inform 2019;18:1176935119871933 [Crossref] [PubMed]
- Pacheco JM, Byers LA. Temozolomide plus PARP Inhibition in Small-Cell Lung Cancer: Could Patient-Derived Xenografts Accelerate Discovery of Biomarker Candidates? Cancer Discov 2019;9:1340-2. [Crossref] [PubMed]
- Mehner C, Oberg AL, Goergen KM, et al. EGFR as a prognostic biomarker and therapeutic target in ovarian cancer: evaluation of patient cohort and literature review. Genes Cancer 2017;8:589-99. [Crossref] [PubMed]
- Doi A, Park IH, Wen B, et al. Differential methylation of tissue- and cancer-specific CpG island shores distinguishes human induced pluripotent stem cells, embryonic stem cells and fibroblasts. Nat Genet 2009;41:1350-3. [Crossref] [PubMed]
- Keshet I, Schlesinger Y, Farkash S, et al. Evidence for an instructive mechanism of de novo methylation in cancer cells. Nat Genet 2006;38:149-53. [Crossref] [PubMed]
- Dai W, Cheung AK, Ko JM, et al. Comparative methylome analysis in solid tumors reveals aberrant methylation at chromosome 6p in nasopharyngeal carcinoma. Cancer Med 2015;4:1079-90. [Crossref] [PubMed]
(English Language Editor: J. Jones)