Six mutator-derived lncRNA signature of genome instability for predicting the clinical outcome of colon cancer
Introduction
Worldwide, despite advances in the diagnosis and treatment of colon adenocarcinoma (COAD), its morbidity and mortality rates remain high (1). The TNM staging system remains the world’s gold standard for selecting cancer treatment or predicting prognosis. Nonetheless, despite identical clinical characteristics, the prognosis amongst patients can differ significantly due to the high levels of heterogeneity in colon cancer (2). From the progress, development, and response to treatment, colon cancer has shown high complexity in terms of clinical and molecular heterogeneity (3). Hence, to assess the clinical outcomes of patients with colon cancer more accurately, the urgent identification of novel biomarkers is imperative.
One of the hallmarks of cancer has been reported to be genomic instability. There are many forms of genomic instability. Most cancers have a form called chromosomal instability (CIN), which refers to the high rate of change in the structure and number of chromosomes in cancer cells over time compared to normal cells. Other forms of genomic instability have also been described, including microsatellite instability (MSI; also known as MIN), and forms of genomic instability characterized by an increased frequency of base pair mutations (4). Genomic instability has been linked with the progression and survival of colon cancer, and is an important prognostic factor (5). In colorectal cancer (CRC), the cancer was induced by mutated genes The evolution of CRC is caused by a variety of genetic and epigenetic abnormalities, including defective DNA mismatch repair (DMMR) and mutations in Kirsten-RAS (KRAS) and BRAF proto-oncogenes (6,7). This suggests the potential of molecular signatures of genomic instability to predict prognosis. For example, a 12-gene genomic instability signature was identified through an analysis of the gene expression profiles of breast cancer specimens by Habermann et al. (8). A meta-analysis of the expression of miRNAs showed their association with MSI in colon cancer tissues (9). Many studies have also shown that upregulated immune checkpoints (e.g., CTLA 4, PD-1, and/or PD-L1/CD274) have been found in highly mutated tumors with DMMR or high MSI (MSI-H), which may benefit from immunotherapy (10,11).
Transcripts that do not indicate any potential for protein coding and are longer than 200 nt have been broadly classified as long non-coding RNAs (lncRNAs) (12). Growing in vivo and in vitro evidence over the years have indicated the significant role played by lncRNAs across various biological processes. In particular, aberrant lncRNA expression has been shown to play a role in metastasis, tumor progression, and cell proliferation (13). Despite lncRNAs being abnormally expressed in numerous cancers, the functions of these lncRNAs have not been determined. In the maintenance of genomic instability, the crucial role of lncRNAs has been revealed from emerging evidence. A study showed that the non-coding RNA NORAD regulates genomic stability by chelating pumilio proteins (14). Another study showed that RNA exosomes control super-enhancer activity by regulating lncRNA transcription (15). In colon cancer, it has been shown that lncRNA CCAT2 induces CIN through BOP1-AURKb signaling, leading to poor prognosis (16). However, so far, lncRNAs associated with genomic instability and their clinical significance have not been mapped and explored.
To evaluate the potential of the lncRNA signature being considered as an indicator of genomic stability in colon cancer, a mutator hypothesis-derived computational frame integrating lncRNA expression and somatic mutation profiles in a tumor genome was developed in this study. This study explored the role of lncRNAs in genomic instability and cancer prognosis in colon cancer which was the main difference from the article you mentioned. We further explored the expression of immune checkpoint between high-risk group and low-risk group in this article. We used the CIBERSORT algorithm to estimate the proportions of 22 immune cell types in colon cancer samples to further investigate the relationship between the genome instability-derived lncRNA signature (GILncSig) and immune cell infiltration.
We present the following article in accordance with the TRIPOD reporting checklist (available at https://dx.doi.org/10.21037/jgo-21-494).
Methods
Data download
The Cancer Genome Atlas (TCGA) database (https://portal.gdc.cancer.gov/) provided the information of the somatic mutations, the fragments per kilobase of exon model per million mapped fragments (FPKM) type of RNA-seq expression data, and the clinical features of patients with COAD. For further study, a total of 446 paired samples with common clinicopathological features, somatic mutation information, survival information, and mRNA and lncRNA expression profiles were obtained. All the colon cancer patients were divided into two sets, namely the test and training sets. To identify the prognostic lncRNA signature and build a prognostic risk model, a total of 224 patients from TCGA were placed in the training set, while 222 patients were used to validate the performance of the prognostic risk model. Furthermore, from TCGA database, the corresponding lncRNA expression data and the somatic mutation information of patients were also downloaded. Table 1 provides a brief summary of the pathological and clinical characteristics. The study was conducted in accordance with the Declaration of Helsinki (as revised in 2013).
Table 1
Covariates | Type | Total | Testing | Training | P value |
---|---|---|---|---|---|
Age | ≤65 | 183 | 93 | 90 | 0.786 |
>65 | 263 | 129 | 134 | ||
Gender | Female | 212 | 104 | 108 | 0.8459 |
Male | 234 | 118 | 116 | ||
Stage | Stage I–II | 250 | 126 | 124 | 0.7071 |
Stage III–IV | 185 | 89 | 96 | ||
Unknown | 11 | 7 | 4 | ||
T | T1–2 | 86 | 47 | 39 | 0.3878 |
T3–4 | 359 | 175 | 184 | ||
Unknown | 1 | 0 | 1 | ||
M | M0 | 329 | 161 | 168 | 1 |
M1 | 61 | 30 | 31 | ||
Unknown | 56 | 31 | 25 | ||
N | N0 | 265 | 134 | 131 | 0.7585 |
N1–2 | 181 | 88 | 93 |
COAD, colon adenocarcinoma.
Identification of genome instability-associated lncRNAs
We developed a mutator hypothesis-derived computational framework by integrating somatic mutation profiles and lncRNA expression profiles in tumor genomes to identify lncRNAs associated with genomic instability using the following steps: (I) the cumulative number of somatic mutations in each patient was calculated; (II) the cumulative number of somatic mutations among patients was ranked in decreasing order; (III) the bottom 25% of patients were defined as the genomically stable (GS)-like group, while the top 25% were defined as the genomically unstable (GU)-like group; (IV) the expression profiles of the lncRNAs between the GS and GU groups were compared; (V) the genome instability-associated lncRNAs were identified as differentially expressed lncRNAs [absolute value of the fold change greater than 2 and their false discovery rate (FDR) adjusted P value being less than 0.05].
Enrichment analysis
We identified mRNAs pairwise expressed with lncRNAs associated with genomic instability. The top 10 mRNAs associated with each lncRNA were selected. On this basis, a co-expression network was constructed. The ‘clusterProfiler’ R package and the ‘ggplot2’ R package were used for Gene Ontology (GO) enrichment analysis and Kyoto Encyclopedia of Genes and Genomes (KEGG) pathway analysis (17,18).
Estimation of immune cell infiltration
We used the CIBERSORT algorithm to estimate the proportions of 22 immune cell types in colon cancer samples from gene expression data to further investigate the relationship between the GILncSig and immune cell infiltration (19). We removed samples with P>0.05 and retained samples with P<0.05 for further analysis. We used the Wilcoxon rank-sum test to identify if there was a significant difference in the proportion of immune cells between the low- and high-risk groups. In addition, the Kaplan-Meier method was used to evaluate the relationship between immune cell infiltration and patient prognosis.
Statistical analysis
To evaluate the overall survival and the association with the expression levels of genome instability-associated lncRNAs, multivariate and univariate Cox proportional hazard regression analyses were conducted. A GILncSig for prognostic prediction was developed as described below, based on the coefficients from the multivariate regression analysis and the levels of expression of the prognostic genome instability-associated lncRNAs:
where GILncSig (patient) represented the prognostic risk score for the colon cancer patient, lncRNAi indicated the ith prognostic lncRNA, and expr (lncRNAi) was the expression level of lncRNAi for the patient. The coef (lncRNAi) was representative of the contribution of the lncRNAi to the prognostic risk scores that were obtained from the multivariate Cox analysis regression coefficient. To segregate patients into the low-risk group with a low GILncSig or the high-risk group with a high GILncSig, the median scores of patients in the training set were used as the risk cutoff.
To assess the difference in survival between the low- and high-risk groups with a 5% significance level, the log-rank test was applied. To determine the survival rate, the Kaplan-Meier method was applied. For assessing the independence of the GILncSig from the other key clinical factors, stratified and multivariate Cox regression analyses were conducted. With the Cox analysis, the 95% confidence interval (CI) and hazard ratio (HR) were calculated. The time-dependent receiver operating characteristic (ROC) curve was used to evaluate the performance of the GILncSig. R version 4.0.3 was used to perform all statistical analyses.
Results
Identification of genomic instability-related lncRNAs in colon cancer patients
We calculated and sorted the cumulative number of somatic mutations per patient to identify the lncRNAs associated with genomic instability. Based on the results of somatic mutations in decreasing order, the top 25% (n=112) of patients were divided into the GU-like group and the bottom 25% (n=101) of patients were divided into the GS-like group. In order to identify the lncRNAs with significant differences, the lncRNA expression profiles of the 112 patients from the GU-like group were compared with the 101 patients in the GS-like group. A total of 137 lncRNAs were found to be significantly differentially expressed, with an absolute value of fold change greater than 2 and FDR-adjusted P value less than 0.05. In total, 81 lncRNAs were upregulated and 56 downregulated, as detailed in Table S1. Figure 1A shows the top 40 lncRNAs that were significantly different. Using the set of 137 differentially expressed lncRNAs, the samples from TCGA cohort were divided into GS-like and GU-like groups based on the expression levels of the differentially expressed lncRNAs (Figure 1B). Between the two groups, the somatic mutation pattern was found to be significantly different. The GU-like group contained higher cumulative somatic mutations compared to the GS-like group (P=0.014; Figure 1C). Further study showed higher PD-L1 (CD274) expression in the GU group, consistent with another previous study (P=1.4×10−8; Figure 1D) (11).
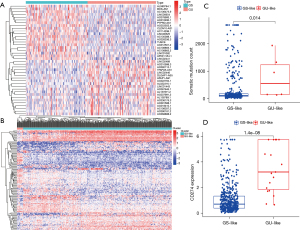
Enrichment analysis
We measured the expression correlation between the 137 differentially expressed lncRNAs and protein coding genes, and constructed a lncRNA-mRNA co-expression network (Figure 2A). The mRNAs co-expressed with lncRNAs were enriched by GO and KEGG analysis. Based on GO enrichment analysis, the mRNAs in this co-expression network were mainly enriched in gland development, organelle subcompartment, and steroid binding (Figure 2B). According to KEGG pathway enrichment analysis, the main enrichment pathways were the chemokine signaling pathway, proteoglycans in cancer, and the NOD-like receptor signaling pathway (Figure 2C). These pathways are closely related to the occurrence and progression of cancer.
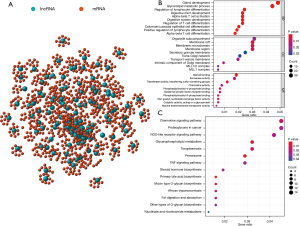
Development of the GILncSig
Colon cancer patients from TCGA were randomly divided into the training set and test set to further investigate the prognostic roles of these candidate genome instability-associated lncRNAs, as detailed in Table 1. It was observed that 12 genome instability-associated lncRNAs were significantly associated with the prognosis of colon cancer patients in the training set (all P<0.05), and the prognostic forest plot of these 12 lncRNAs is shown in Figure 3. Furthermore, the multivariate Cox proportional hazards regression analysis was conducted between the 12 candidate lncRNAs to single out the lncRNAs with independent prognostic value. Finally, 6 of the 12 candidate lncRNAs (LINC00896, AC007996.1, NKILA, AP003555.2, MIRLET7BHG, and AC009237.14) were identified as independent prognostic lncRNAs as their P values in the multivariate Cox analysis were less than 0.05 (Table 2). Subsequently, based on the expression levels of the six independent prognostic genomic instability-associated lncRNAs and the coefficients of the multivariate Cox analysis, a GILncSig was developed to determine the prognostic risk of patients with colon cancer. GILncSig score = (0.244155 × expression level of LINC00896) + (0.626462 × expression level of AC007996.1) + (0.295227 × expression level of NKILA) + (0.209866 × expression level of AP003555.2) + (0.351340 × expression level of MIRLET7BHG) + (0.196452 × expression level of AC009237.14). In the GILncSig score, the coefficients of all lncRNAs were positive, and their high expression levels were associated with poorer survival. The expression levels of lncRNAs in the GILncSig in the high- and low-risk groups are shown in Figure 4. Results showed that all 6 lncRNAs in the GILncSig were upregulated in the high-risk group, both in the training group (Figure 4A) and in TCGA patient groups (Figure 4C). However, only four lncRNAs (AC007996.1, NKILA, AP003555.2, and AC009237.14) were upregulated in the test group (Figure 4B).
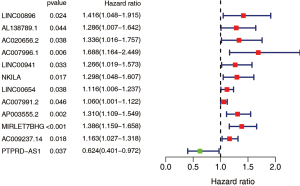
Table 2
ID | Coef | HR | HR.95L | HR.95H | P value |
---|---|---|---|---|---|
LINC00896 | 0.2441547 | 1.276541 | 0.960602 | 1.696392 | 0.092392 |
AC007996.1 | 0.6264617 | 1.870978 | 1.234731 | 2.835079 | 0.003133 |
NKILA | 0.2952265 | 1.343430 | 1.080575 | 1.670227 | 0.007871 |
AP003555.2 | 0.2098663 | 1.233513 | 1.002866 | 1.517206 | 0.046915 |
MIRLET7BHG | 0.3513404 | 1.420971 | 1.164792 | 1.733492 | 0.000532 |
AC009237.14 | 0.1964520 | 1.217076 | 1.068190 | 1.386715 | 0.003169 |
LncRNAs, long non-coding RNAs; coef, coefficient; HR, hazard ratio.
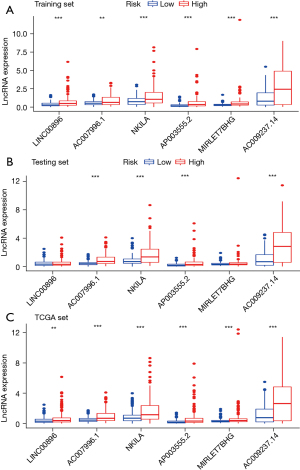
Risk scores for patients in both the training set and the test set were obtained, and these patients were then classified into different prognostic groups using the median risk score as a threshold. We then performed Kaplan-Meier analyses of patients in the high- and low-risk groups, and the results showed that survival outcomes in the low-risk group were significantly better than those in the high-risk group in TCGA groups (training set, P<0.001, Figure 5A; test set, P=0.005, Figure 5B; TCGA sets, P<0.001, Figure 5C). Time-dependent ROC curve analysis of the GILncSig showed that the areas under the curve (AUCs) of the training set, testing set and TCGA groups were 0.691, 0.661, and 0.675, respectively (Figure 5D-5F).
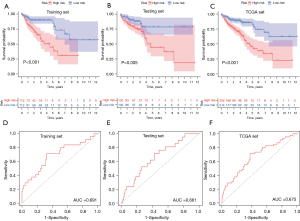
The number of somatic mutations and the expression level of PD-L1 in each group
We then explored the number of somatic mutations and the differences in PD-L1 expression levels between the high- and low-risk patients. We first developed a set of risk figures for the three datasets, including a heat map of lncRNA expression and the distribution of patient risk scores. As is shown in Figure 6A, the expression levels of all lncRNAs in the training set increased with the increase in GILncSig score. These results were further verified in the test set and the TCGA set (Figure 6B,6C). As shown in Figure 6D-6F, the count of somatic mutations in patients in the high-risk group was higher than that of patients in the low-risk group (P=0.088, training set; P=0.066, test set; P=0.013, total set). The expression level of PD-L1 (CD274) was significantly higher in the high-risk group than in the low-risk group (P=0.01, training set; Figure 6G). However, in the test set, the expression of PD-L1 (CD274) was not significantly different among the high- and low-risk groups (P=0.15, Figure 6H). The expression level of PD-L1 (CD274) was significantly higher in the high-risk group than in the low-risk group (TCGA set; Figure 6I). We also did mutations of the molecule CD274 across different groups, but we found that it was very stable, with only two mutations in 400 patient samples (Table S2). Our results show that PD-L1 expression is higher in the high-risk group, suggesting that these patients may benefit from immunotherapy.
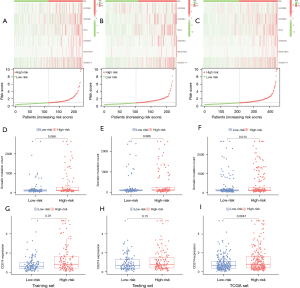
GILncSig and clinical factors
We used the chi-square test to investigate the relationship between risk and clinical characteristics. As shown in Table 3, the stages, T stage, and N stage were significantly correlated with the risk score in the training group and the total set. The higher a patient’s risk score, the higher their stage and the higher their N stage. All the clinical characteristics showed no significant differences between the high- and low-risk groups in the test set.
Table 3
Parameters | Training set (n=224) | Testing set (n=222) | Total set (n=446) | |||||||||||
---|---|---|---|---|---|---|---|---|---|---|---|---|---|---|
HR | LR | χ2 | P | HR | LR | χ2 | P | HR | LR | χ2 | P | |||
Age (y) | ||||||||||||||
≤65 | 42 | 48 | 0.4643 | 0.4956 | 52 | 41 | 0.1127 | 0.7371 | 94 | 89 | 0.0178 | 0.8938 | ||
>65 | 70 | 64 | 68 | 61 | 138 | 125 | ||||||||
Gender | ||||||||||||||
Male | 57 | 59 | 66 | 52 | 123 | 111 | ||||||||
Female | 55 | 53 | 0.0179 | 0.8936 | 54 | 50 | 0.2145 | 0.6432 | 109 | 103 | 0.0218 | 0.8826 | ||
Stage | ||||||||||||||
Stage I–II | 52 | 72 | 7.4875 | 0.0062 | 64 | 62 | 0.9353 | 0.3335 | 116 | 134 | 7.3455 | 0.0067 | ||
Stage III–IV | 59 | 37 | 52 | 37 | 111 | 74 | ||||||||
Unknown | 4 | 1 | 5 | 6 | ||||||||||
T | ||||||||||||||
T1–2 | 18 | 21 | 0.1035 | 0.7476 | 19 | 28 | 3.79 | 0.0516 | 37 | 49 | 2.9457 | 0.0861 | ||
T3–4 | 93 | 91 | 101 | 74 | 194 | 165 | ||||||||
Unknown | 1 | 0 | 1 | 0 | ||||||||||
M | ||||||||||||||
M0 | 79 | 89 | 1.5986 | 0.2061 | 86 | 75 | 0.6441 | 0.4222 | 165 | 164 | 2.5732 | 0.1087 | ||
M1 | 19 | 12 | 19 | 11 | 38 | 23 | ||||||||
Unknown | 14 | 11 | 15 | 16 | 29 | 27 | ||||||||
N | ||||||||||||||
N0 | 53 | 78 | 10.5905 | 0.0011 | 68 | 66 | 1.1722 | 0.2789 | 121 | 144 | 9.956 | 0.0016 | ||
N1–2 | 59 | 34 | 52 | 36 | 111 | 70 |
LncRNAs, long non-coding RNAs; HR, high risk; LR, low risk.
The landscape of immune infiltration in COAD
To further investigate the relationship between risk score and immune cell infiltration, we used the CIBERSORT algorithm to estimate the proportions of 22 immune cell types in the COAD cohort from gene expression data. The Wilcoxon rank-sum test was used to explore whether there were differences between the 22 kinds of immune cells in different groups. As shown in Figure 7A, T follicular helper cells (P=0.018), resting NK cells (P=0.029), and M1 macrophages (P=0.026) varied significantly between high- and low-risk score patients. In addition, Kaplan-Meier analysis showed that a lower proportion of regulatory T cells (Tregs) were associated with better overall survival (P=0.018, Figure 7B). However, a higher proportion of resting mast cells were associated with better overall survival (P=0.008, Figure 7C).
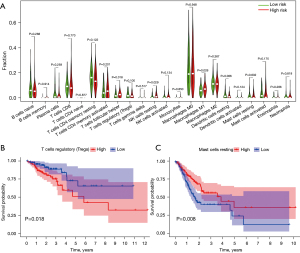
Discussion
The initiation, development, and treatment of colon cancer have been the subject of investigation for the past several years (20-22). Patients continue to be classified according to different therapeutic groups based on their pathological features, while the most important prognostic factors, such as the traditional histopathological features of tumor size, grade, and stage, also continue to be used (23,24). Nonetheless, due to the various limitations of traditional clinicopathological features, the clinical outcome of patients with colon cancer remains highly heterogeneous (25). Genomic instability is not only a common feature of most cancers, but is also considered to be one of the factors affecting the prognosis of colon cancer (26). In colon cancer progression and recurrence, genomic instability has a crucial and dominating role, thereby suggesting the important diagnostic and prognostic implications indicated by the pattern and degree of genomic instability (27,28). Nevertheless, quantitative measures of the degree of genomic instability have remained a challenge. For predicting genomic instability, concerted efforts are being made to develop gene or miRNA signatures and identify miRNAs associated with genomic instability (29).
LncRNAs, a novel class of non-coding RNAs, have recently gained significance as important components of tumors. The dysregulated expression of lncRNAs in cancer is related to disease progression, and lncRNAs have the potential to be used as prognostic markers for patients (30,31). With recent advances in the understanding of the functional mechanisms of lncRNAs, lncRNAs are also critical to genome stability (32). The systematic exploration of their clinical significance in cancers and the genome-wide identification of genome instability-associated lncRNAs remain in their infancy despite certain efforts being made. Hence, to identify genome instability-associated lncRNAs combining lncRNA expression and the tumor mutator phenotype, a computational frame was developed. The results showed that 137 novel genome instability-associated lncRNAs were identified after the lncRNA expression profiles were combined with the somatic mutation profiles of colon cancer. We then constructed a lncRNA-mRNA co-expression network analysis of 128 genes that co-expressed lncRNAs associated with genomic instability. GO enrichment analysis showed that the mRNAs in this co-expression network were mainly enriched in gland development, organelle subcompartment, and steroid binding. According to KEGG pathway enrichment analysis, the main enrichment pathways were the chemokine signaling pathway, proteoglycans in cancer, and the NOD-like receptor signaling pathway, which are closely related to the occurrence and progression of cancer.
Colon cancer patients from TCGA were randomly divided into the training set and test set. Through multivariate Cox analysis, we observed that six genomic instability-related lncRNAs (LINC00896, AC007996.1, NKILA, AP003555.2, MIRLET7BHG, and AC009237.14) were significantly associated with the prognosis of patients in the training set. A GILncSig was developed to determine the prognostic risk of patients with colon cancer: GILncSig score = (0.244155 × expression level of LINC00896) + (0.626462 × expression level of AC007996.1) + (0.295227 × expression level of NKILA) + (0.209866 × expression level of AP003555.2) + (0.351340 × expression level of MIRLET7BHG) + (0.196452 × expression level of AC009237.14). The results showed that all six lncRNAs were associated with poor prognosis, which has rarely been reported in the literature. NKILA is a lncRNA that interacts with NF-κB. A study showed that NKILA promotes tumor immune evasion by sensitizing T cells to activation-induced cell death in breast cancer (33). Another study also showed that lncRNA AP003555.2 was associated with poor prognosis in patients with colon cancer (34). Furthermore, one study suggested that MIRLET7BHG was associated with polycystic ovary syndrome in women (35). Another study showed that autophagy-related lncRNA AC009237.14 was associated with poor prognosis in colon cancer patients (36). We further investigated the association between the GILncSig and clinical features. The results showed that higher risk scores were associated with more advanced stage and N stage. In addition, there was an obvious survival difference between the high- and low-risk groups according to our model, that is, the high-risk group had a shorter survival time and worse prognosis, and the AUC in TCGA groups was greater than 0.65, indicating that the prediction results of our model were relatively accurate.
Maintaining genomic stability to a large extent, nucleotide excision repair can specifically prevent mutations induced by environmental carcinogens. Moreover, some patients with MSI-H or DMMR CRC appear to be prone to persistent clinical responses to checkpoint inhibitors, providing a new treatment option for patients with advanced disease (37). We then examined the number of somatic mutations and the expression of PD-L1 (CD274) between high- and low-risk patients. The results showed that the number of somatic mutations in the high-risk group was higher than that in the low-risk group, and the expression level of PD-L1 (CD274) in the high-risk group was significantly higher than that in the low-risk group. This suggests that our GILncSig is beneficial in differentiating patients who are sensitive to immunotherapy.
We further investigated the relationship between risk score and immune cell infiltration, and showed that there were more T follicular helper cells, resting NK cells, and M1 macrophages in the high-risk group. Besides, a low proportion of Tregs is related to a better prognosis. In a previous study, Tregs from cancer patients inhibited the mechanism of traditional T cell migration and thus affected patient outcomes (38). Mast cells have been shown to promote the development of colon cancer and could be a potential therapeutic target (39). All of these studies confirm our conclusions.
Although our study provides important insights for better assessing genomic instability and prognosis in colon cancer patients, it still has some limitations that require further investigation. We need more independent datasets such as Gene Expression Omnibus (GEO) datasets to validate the GILncSig to ensure its robustness and repeatability. In addition, we need to conduct further experimental validation to understand the regulatory mechanisms of the GILncSig in maintaining genomic instability.
Conclusions
In conclusion, examining the role of lncRNAs in genome instability through a mutator hypothesis-derived computational frame for identifying genome instability-associated lncRNAs was proposed by this study, providing a critical approach and resource for further studies. The levels of somatic mutations and PD-L1 (CD274) expression in the high-risk group were higher than those in the low-risk group, which indicated that high-risk groups may be sensitive to immunotherapy. The GILncSig can predict the outcome of patients with COAD and provides a new therapeutic direction.
Acknowledgments
For contributors to the TRIPOD statement, see
Funding: This work was supported by the National Natural Science Foundation of China (No: 81970358), and Natural Science Foundation of Liaoning Province (1561449995778).
Footnote
Reporting Checklist: The authors have completed the TRIPOD reporting checklist. Available at https://dx.doi.org/10.21037/jgo-21-494
Conflicts of Interest: All authors have completed the ICMJE uniform disclosure form (available at https://dx.doi.org/10.21037/jgo-21-494). The authors have no conflicts of interest to declare.
Ethical Statement: The authors are accountable for all aspects of the work in ensuring that questions related to the accuracy or integrity of any part of the work are appropriately investigated and resolved. The study was conducted in accordance with the Declaration of Helsinki (as revised in 2013). Institutional ethical approval and informed consent were waived.
Open Access Statement: This is an Open Access article distributed in accordance with the Creative Commons Attribution-NonCommercial-NoDerivs 4.0 International License (CC BY-NC-ND 4.0), which permits the non-commercial replication and distribution of the article with the strict proviso that no changes or edits are made and the original work is properly cited (including links to both the formal publication through the relevant DOI and the license). See: https://creativecommons.org/licenses/by-nc-nd/4.0/.
References
- Bray F, Ferlay J, Soerjomataram I, et al. Global cancer statistics 2018: GLOBOCAN estimates of incidence and mortality worldwide for 36 cancers in 185 countries. CA Cancer J Clin 2018;68:394-424. [Crossref] [PubMed]
- Dienstmann R, Mason MJ, Sinicrope FA, et al. Prediction of overall survival in stage II and III colon cancer beyond TNM system: a retrospective, pooled biomarker study. Ann Oncol 2017;28:1023-31. [Crossref] [PubMed]
- Hutchins G, Southward K, Handley K, et al. Value of mismatch repair, KRAS, and BRAF mutations in predicting recurrence and benefits from chemotherapy in colorectal cancer. J Clin Oncol 2011;29:1261-70. [Crossref] [PubMed]
- Negrini S, Gorgoulis VG, Halazonetis TD. Genomic instability--an evolving hallmark of cancer. Nat Rev Mol Cell Biol 2010;11:220-8. [Crossref] [PubMed]
- Samowitz WS, Sweeney C, Herrick J, et al. Poor survival associated with the BRAF V600E mutation in microsatellite-stable colon cancers. Cancer Res 2005;65:6063-9. [Crossref] [PubMed]
- Jass JR. Classification of colorectal cancer based on correlation of clinical, morphological and molecular features. Histopathology 2007;50:113-30. [Crossref] [PubMed]
- Grady WM, Carethers JM. Genomic and epigenetic instability in colorectal cancer pathogenesis. Gastroenterology 2008;135:1079-99. [Crossref] [PubMed]
- Habermann JK, Doering J, Hautaniemi S, et al. The gene expression signature of genomic instability in breast cancer is an independent predictor of clinical outcome. Int J Cancer 2009;124:1552-64. [Crossref] [PubMed]
- Mjelle R, Sjursen W, Thommesen L, et al. Small RNA expression from viruses, bacteria and human miRNAs in colon cancer tissue and its association with microsatellite instability and tumor location. BMC Cancer 2019;19:161. [Crossref] [PubMed]
- Le DT, Uram JN, Wang H, et al. PD-1 blockade in tumors with mismatch-repair deficiency. N Engl J Med 2015;372:2509-20. [Crossref] [PubMed]
- Llosa NJ, Cruise M, Tam A, et al. The vigorous immune microenvironment of microsatellite instable colon cancer is balanced by multiple counter-inhibitory checkpoints. Cancer Discov 2015;5:43-51. [Crossref] [PubMed]
- Sun M, Kraus WL. From discovery to function: the expanding roles of long noncoding RNAs in physiology and disease. Endocr Rev 2015;36:25-64. [Crossref] [PubMed]
- Sun M, Gadad SS, Kim DS, et al. Discovery, annotation, and functional analysis of long noncoding RNAs controlling cell-cycle gene expression and proliferation in breast cancer cells. Mol Cell 2015;59:698-711. [Crossref] [PubMed]
- Lee S, Kopp F, Chang TC, et al. Noncoding RNA NORAD regulates genomic stability by sequestering PUMILIO proteins. Cell 2016;164:69-80. [Crossref] [PubMed]
- Pefanis E, Wang J, Rothschild G, et al. RNA exosome-regulated long non-coding RNA transcription controls super-enhancer activity. Cell 2015;161:774-89. [Crossref] [PubMed]
- Chen B, Dragomir MP, Fabris L, et al. The long noncoding RNA CCAT2 induces chromosomal instability through BOP1-AURKB signaling. Gastroenterology 2020;159:2146-2162.e33. [Crossref] [PubMed]
- Ashburner M, Ball CA, Blake JA, et al. Gene Ontology: tool for the unification of biology. The Gene Ontology Consortium. Nat Genet 2000;25:25-9. [Crossref] [PubMed]
- Kanehisa M, Goto S. KEGG: Kyoto Encyclopedia of Genes and Genomes. Nucleic Acids Res 2000;28:27-30.
- Newman AM, Liu CL, Green MR, et al. Robust enumeration of cell subsets from tissue expression profiles. Nat Methods 2015;12:453-7. [Crossref] [PubMed]
- Dienstmann R, Salazar R, Tabernero J. Personalizing colon cancer adjuvant therapy: selecting optimal treatments for individual patients. J Clin Oncol 2015;33:1787-96. [Crossref] [PubMed]
- Gavin PG, Colangelo LH, Fumagalli D, et al. Mutation profiling and microsatellite instability in stage II and III colon cancer: an assessment of their prognostic and oxaliplatin predictive value. Clin Cancer Res 2012;18:6531-41. [Crossref] [PubMed]
- Brenner H, Kloor M, Pox CP. Colorectal cancer. Lancet 2014;383:1490-502. [Crossref] [PubMed]
- Klaver CEL, Wisselink DD, Punt CJA, et al. Adjuvant hyperthermic intraperitoneal chemotherapy in patients with locally advanced colon cancer (COLOPEC): a multicentre, open-label, randomised trial. Lancet Gastroenterol Hepatol 2019;4:761-70. [Crossref] [PubMed]
- Pagès F, Mlecnik B, Marliot F, et al. International validation of the consensus Immunoscore for the classification of colon cancer: a prognostic and accuracy study. Lancet 2018;391:2128-39. [Crossref] [PubMed]
- Boyne DJ, Cuthbert CA, O'Sullivan DE, et al. Association between adjuvant chemotherapy duration and survival among patients with stage II and III colon cancer: a systematic review and meta-analysis. JAMA Netw Open 2019;2:e194154 [Crossref] [PubMed]
- Pino MS, Chung DC. The chromosomal instability pathway in colon cancer. Gastroenterology 2010;138:2059-72. [Crossref] [PubMed]
- Chen WS, Chen JY, Liu JM, et al. Microsatellite instability in sporadic-colon-cancer patients with and without liver metastases. Int J Cancer 1997;74:470-4. [Crossref] [PubMed]
- Westra JL, Schaapveld M, Hollema H, et al. Determination of TP53 mutation is more relevant than microsatellite instability status for the prediction of disease-free survival in adjuvant-treated stage III colon cancer patients. J Clin Oncol 2005;23:5635-43. [Crossref] [PubMed]
- Mettu RK, Wan YW, Habermann JK, et al. A 12-gene genomic instability signature predicts clinical outcomes in multiple cancer types. Int J Biol Markers 2010;25:219-28. [Crossref] [PubMed]
- Gupta RA, Shah N, Wang KC, et al. Long non-coding RNA HOTAIR reprograms chromatin state to promote cancer metastasis. Nature 2010;464:1071-6. [Crossref] [PubMed]
- Wu Y, Zhang L, Zhang L, et al. Long non-coding RNA HOTAIR promotes tumor cell invasion and metastasis by recruiting EZH2 and repressing E-cadherin in oral squamous cell carcinoma. Int J Oncol 2015;46:2586-94. [Crossref] [PubMed]
- Hu WL, Jin L, Xu A, et al. GUARDIN is a p53-responsive long non-coding RNA that is essential for genomic stability. Nat Cell Biol 2018;20:492-502. [Crossref] [PubMed]
- Huang D, Chen J, Yang L, et al. NKILA lncRNA promotes tumor immune evasion by sensitizing T cells to activation-induced cell death. Nat Immunol 2018;19:1112-25. [Crossref] [PubMed]
- Liu Y, Liu B, Jin G, et al. An integrated three-long non-coding RNA signature predicts prognosis in colorectal cancer patients. Front Oncol 2019;9:1269. [Crossref] [PubMed]
- Butler AE, Hayat S, Dargham SR, et al. Alterations in long noncoding RNAs in women with and without polycystic ovarian syndrome. Clin Endocrinol (Oxf) 2019;91:793-7. [Crossref] [PubMed]
- Cheng L, Han T, Zhang Z, et al. Identification and validation of six autophagy-related long non-coding RNAs as prognostic signature in colorectal cancer. Int J Med Sci 2021;18:88-98. [Crossref] [PubMed]
- Oliveira AF, Bretes L, Furtado I. Review of PD-1/PD-L1 inhibitors in metastatic dMMR/MSI-H colorectal cancer. Front Oncol 2019;9:396. [Crossref] [PubMed]
- Sundström P, Stenstad H, Langenes V, et al. Regulatory T cells from colon cancer patients inhibit effector T-cell migration through an adenosine-dependent mechanism. Cancer Immunol Res 2016;4:183-93. [Crossref] [PubMed]
- Wang S, Li L, Shi R, et al. Mast cell targeted chimeric toxin can be developed as an adjunctive therapy in colon cancer treatment. Toxins (Basel) 2016;8:71. [Crossref] [PubMed]
(English Language Editor: C. Betlzar)